Investments
Japanese Startup Creates Smart Mask Capable of Translating Eight Languages
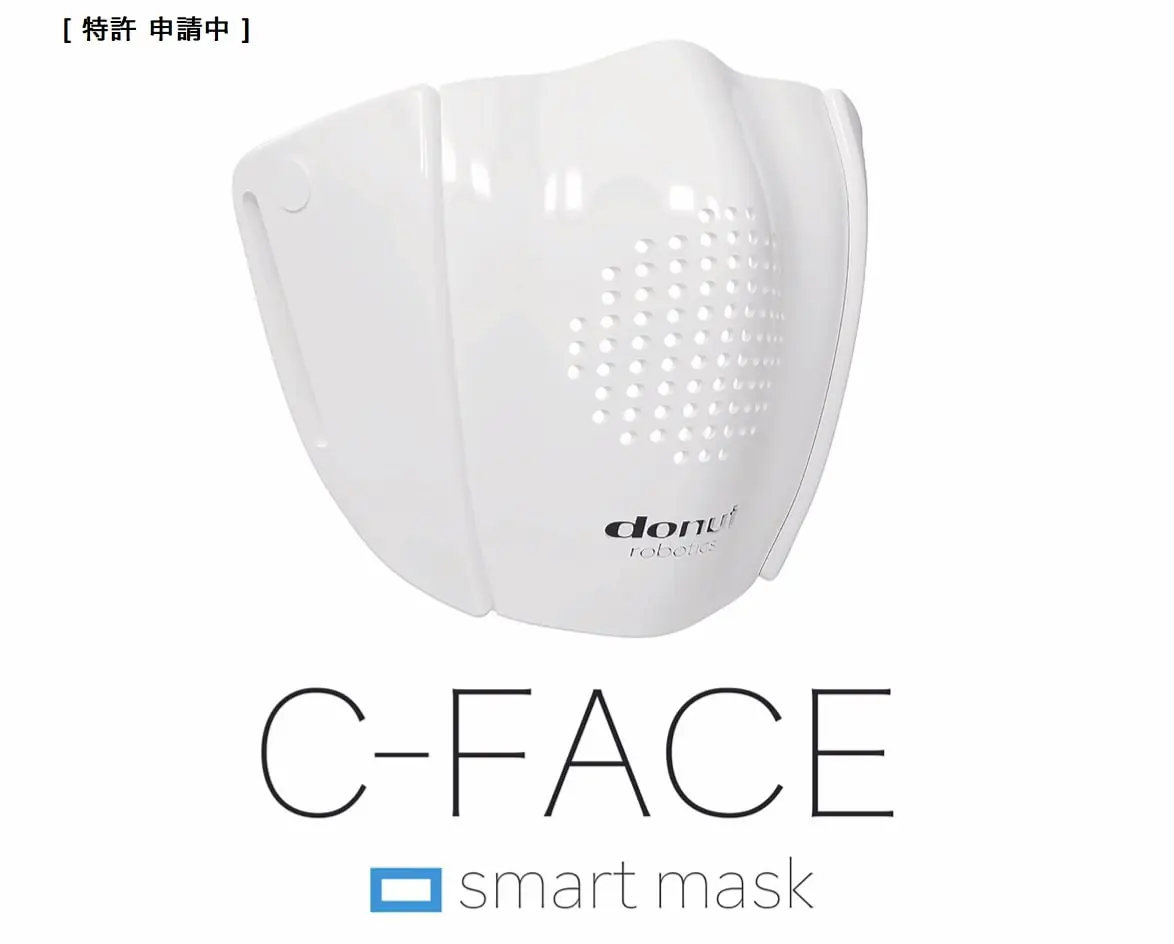
Shortly after the outbreak of the COVID-19 pandemic, the use of face masks became popular throughout the globe. While governments were trying to handle all of the chaos, companies like Donut Robotics were working on new innovations applicable to the time. The Japanese startup created a high-tech version of the cloth face covering with their C-Face Smart mask.
C-Face Smart mask is designed to aid in communication and social distancing during times like this, but it has many other non-pandemic related capabilities as well, such as the ability to translate speech into eight different languages.
According to a statement by the company, “C-face is the world’s first smart mask that works with smartphones … developed by applying robotics technology. We hope this device will be useful in a society where people naturally practice social distancing.”
Cinnamon Robot and Donut Robotics
Donut Robotics did not create the mask during the pandemic, but they did adapt it to use during this time. It’s original intended use was as translation software for the company’s robot called Cinnamon. However, the project was put on hold due to COVID-19, which resulted in the team turning their focus to the face mask.
The company was founded in Kitakyushu City, in Fukuoka prefecture by CEO Taisuke Ono and engineer Takafumi Okabe. The pair wanted to “change the world with small and mobile communication robots.”
After receiving venture capital investment, Ono and Okabe applied to an initiative called Haneda Robotics Lab, which aimed to use robots to provide services to visitors at Tokyo's Haneda Airport.
That is when the company developed the Cinnamon robot as one of four translation robot prototypes that were selected by the initiative in 2016. According to Haneda Robotics Lab, Cinnamon was chosen due to its user-friendly design and impressive aesthetics, as well as the software’s ability to operate efficiently in loud environments.
Following the company’s success with Cinnamon, it was moved to Tokyo with an expanded team.
According to Ono, the software relies on Japanese language-specialized machine learning that was developed by translation experts.
Ono says that when it comes to Japanese language users, “the technology is better than Google API, or other popular technologies,” due to other competitor apps mostly translating to and from English.
Once the COVID-19 pandemic hit Asia earlier this year, the team switched gears and focused on adapting its software to come up with a solution.
“We were running short of money and wondering how to keep the company going,” Ono says.
In the past two months, Donut Robotics has raised more than $800,000 (Dh2.94 million) through Fundinno, a Japanese crowdfunding platform.
The company plans on launching the product in Japan in December, starting with 5,000 and 10,000 masks. The cost is expected to be between $40 and $50, and the company will charge a monthly fee for translation and transcription services. Donut Robotics then plans on releasing it in other parts of the world starting in the second quarter of next year.
C-Face Smart Mask
The C-Face Smart mask can translate from Japanese into English, Chinese, French, Spanish, Korean, Vietnamese, and Indonesian.
The face mask has cutouts in the front in order to keep the air flowing, so it is still necessary for users to wear a standard face mask underneath. It is constructed out of white plastic and silicone, and the impressive technology is Bluetooth capable with an embedded microphone that links to the user’s smartphone.
The Bluetooth chip can connect to smartphones up to 32 feet away, and Ono hopes that the technology will aid in social distancing during the pandemic, especially in environments like hospitals and offices.
“We still have many situations where we have to meet in person, Ono says. “In this new normal…the mask and the app are very helpful.”
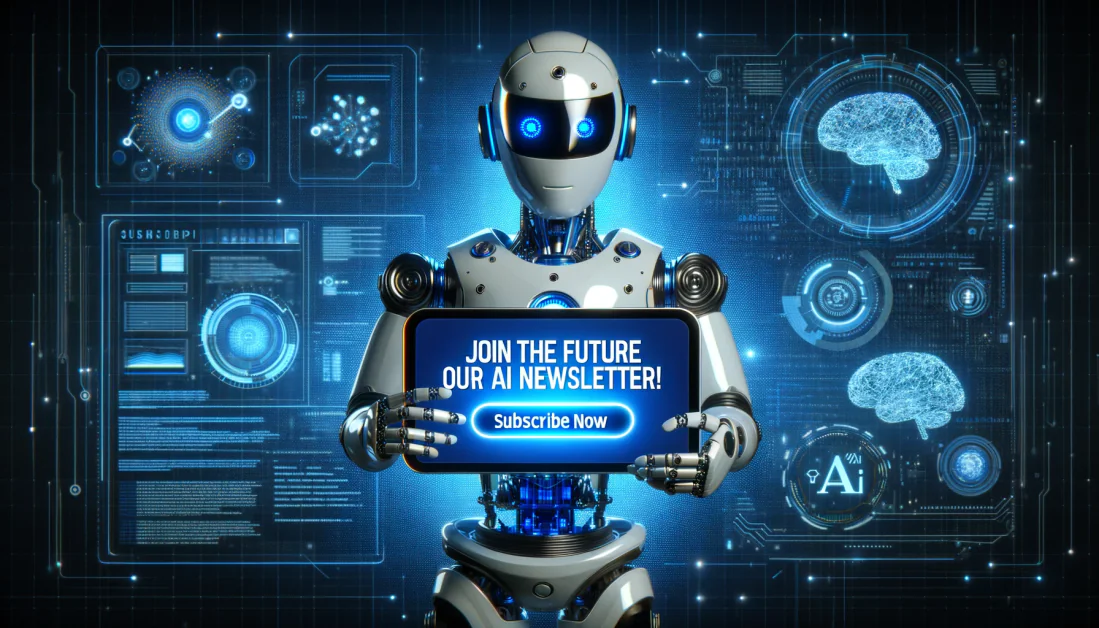
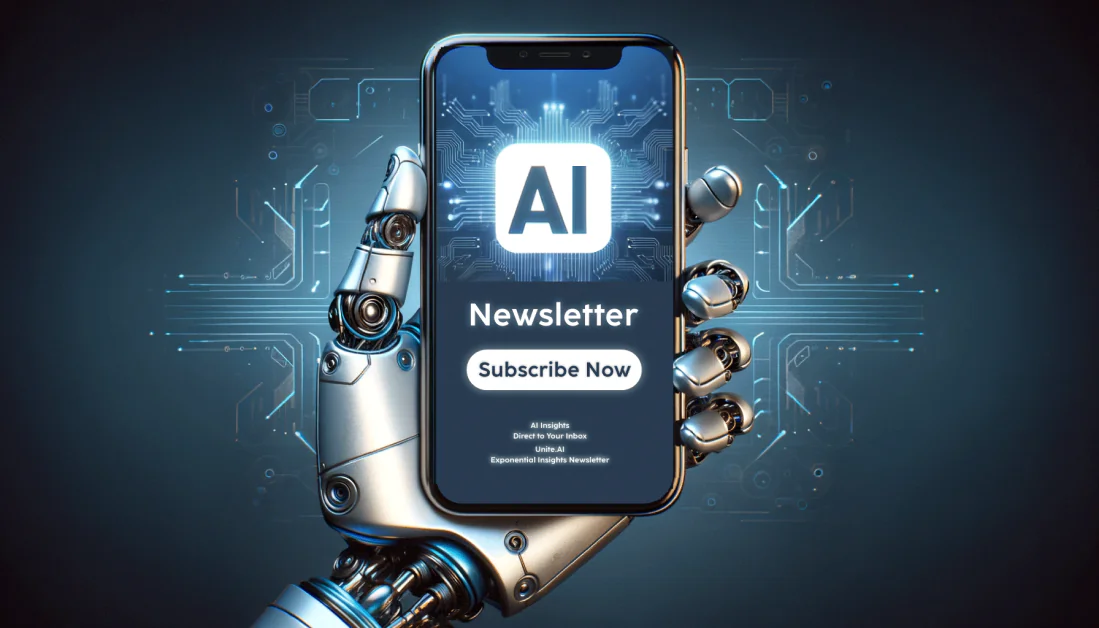