Artificial Intelligence
Is DALL-E 2 Just ‘Gluing Things Together’ Without Understanding Their Relationships?
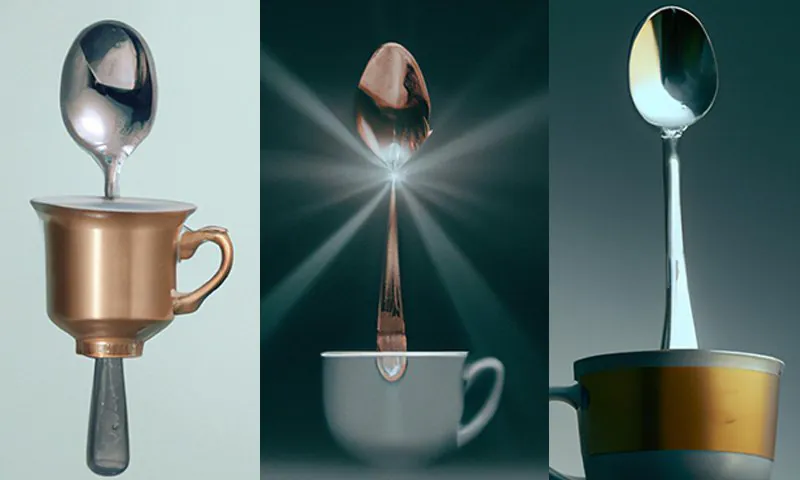
A new research paper from Harvard University suggests that OpenAI's headline-grabbing text-to-image framework DALL-E 2 has notable difficulty in reproducing even infant-level relations between the elements that it composes into synthesized photos, despite the dazzling sophistication of much of its output.
The researchers undertook a user study involving 169 crowdsourced participants, who were presented with DALL-E 2 images based on the most basic human principles of relationship semantics, together with the text-prompts that had created them. When asked if the prompts and the images were related, less than 22% of images were perceived to be pertinent to their associated prompts, in terms of the very simple relationships that DALL-E 2 was asked to visualize.
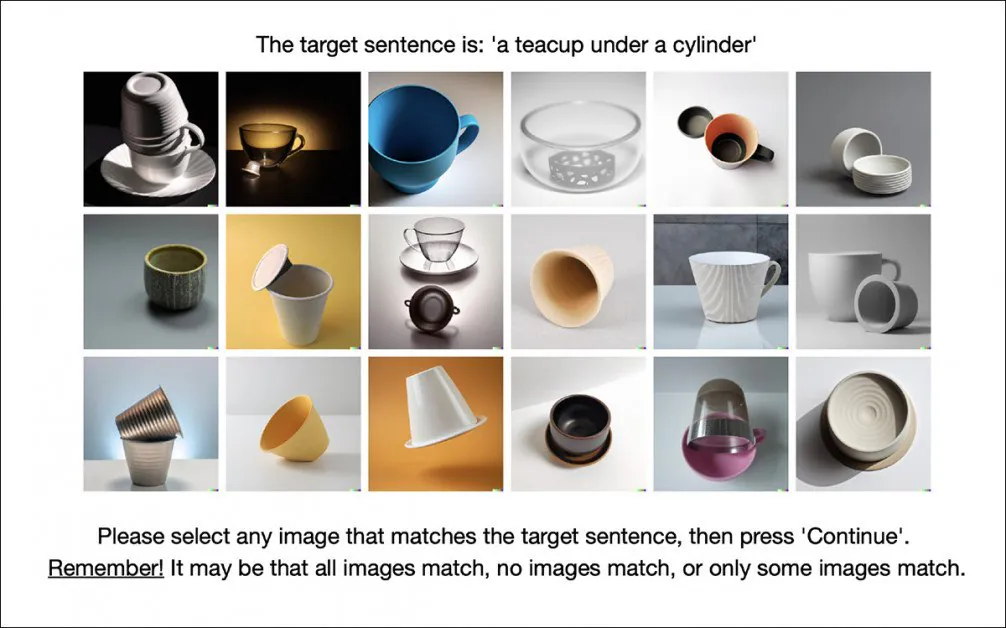
A screen-grab from the trials conducted for the new paper. Participants were tasked with selecting all the images that matched the prompt. Despite the disclaimer at the bottom of the interface, in all cases the images, unbeknownst to the participants, were in fact generated from the displayed associated prompt. Source: https://arxiv.org/pdf/2208.00005.pdf
The results also suggest that DALL-E's apparent ability to conjoin disparate elements may diminish as those elements become less likely to have occurred in the real-world training data that powers the system.
For instance, images for the prompt ‘child touching a bowl' obtained an 87% agreement rate (i.e. the participants clicked on most of the images as being relevant to the prompt), whereas similarly photorealistic renders of ‘a monkey touching an Iguana' achieved only 11% agreement:
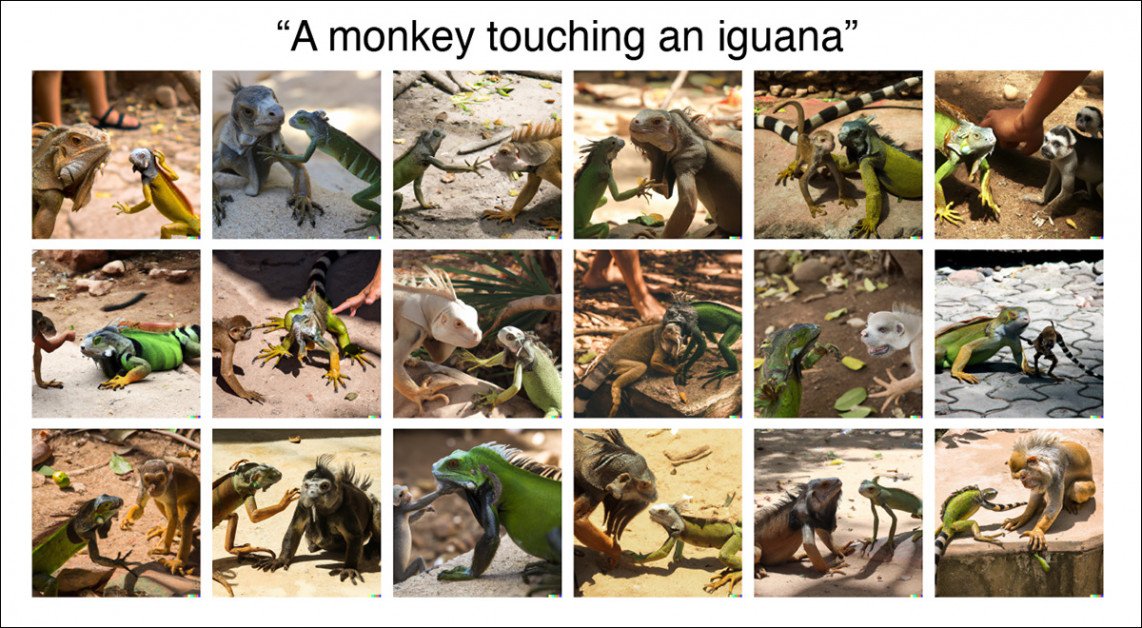
DALL-E struggles to depict the unlikely event of a ‘monkey touching an Iguana', arguably because it is uncommon, more likely non-existent, in the training set.
In the second example, DALL-E 2 frequently gets the scale and even the species wrong, presumably because of a dearth of real-world images that depict this event. By contrast, it's reasonable to expect a high number of training photos related to children and food, and that this sub-domain/class is well-developed.
DALL-E's difficulty in juxtaposing wildly contrastive image elements suggests that the public is currently so dazzled by the system's photorealistic and broadly interpretive capabilities as to not have developed a critical eye for cases where the system has effectively just ‘glued' one element starkly onto another, as in these examples from the official DALL-E 2 site:
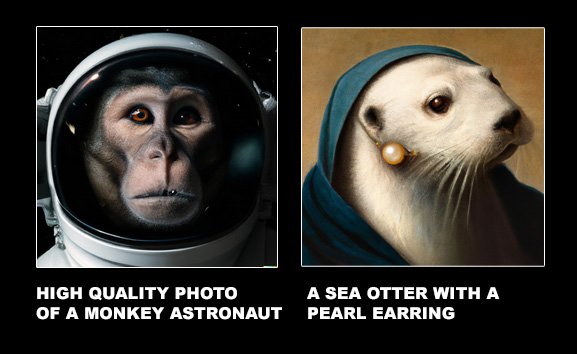
Cut-and-paste synthesis, from the official examples for DALL-E 2. Source: https://openai.com/dall-e-2/
The new paper states*:
‘Relational understanding is a fundamental component of human intelligence, which manifests early in development, and is computed quickly and automatically in perception.
‘DALL-E 2’s difficulty with even basic spatial relations (such as in, on, under) suggests that whatever it has learned, it has not yet learned the kinds of representations that allow humans to so flexibly and robustly structure the world.
‘A direct interpretation of this difficulty is that systems like DALL-E 2 do not yet have relational compositionality.'
The authors suggest that text-guided image generation systems such as the DALL-E series could benefit from leveraging algorithms common to robotics, which model identities and relations simultaneously, due to the need for the agent to actually interact with the environment rather than merely fabricate a blend of diverse elements.
One such approach, titled CLIPort, uses the same CLIP mechanism that serves as a quality assessment element in DALL-E 2:
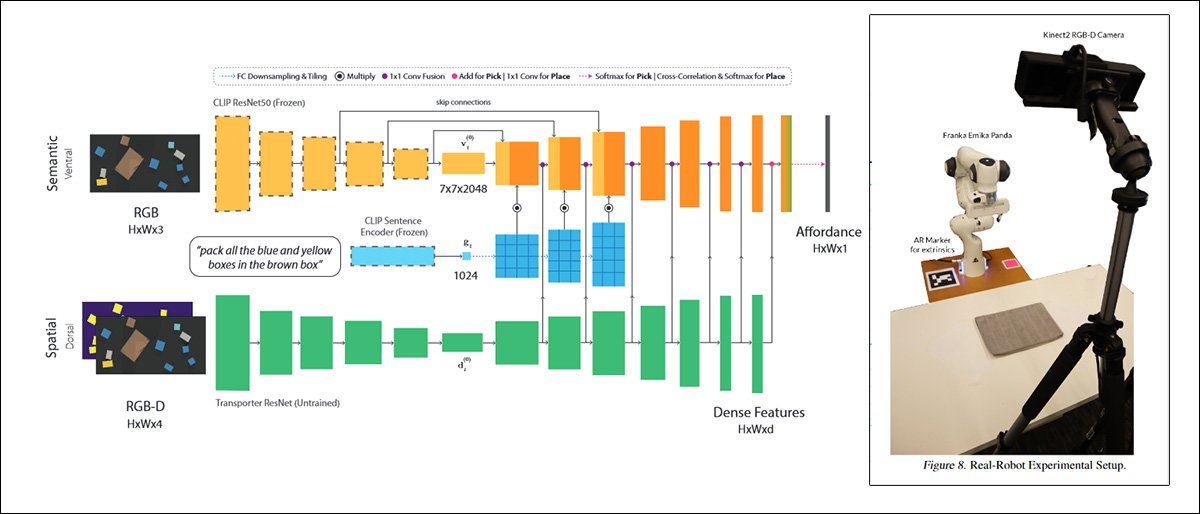
CLIPort, a 2021 collaboration between the University of Washington and NVIDIA, uses CLIP in a context so practical that the systems trained on it must necessarily develop an understanding of physical relationships, a motivator that is absent in DALL-E 2 and similar ‘fantastical' image synthesis frameworks. Source: https://arxiv.org/pdf/2109.12098.pdf
The authors further suggest ‘another plausible upgrade' might be for the architecture of image synthesis systems such as DALL-E to incorporate multiplicative effects in a sole layer of computation, allowing the calculation of relationships in a manner inspired by the information processing capacities of biological systems.
The new paper is titled Testing Relational Understanding in Text-Guided Image Generation, and comes from Colin Conwell and Tomer D. Ullman at Harvard's Department of Psychology.
Beyond Early Criticism
Commenting on the ‘sleight of hand' behind the realism and integrity of DALL-E 2's output, the authors note prior works that have found shortcomings in DALL-E-style generative image systems.
In June this year, UoC Berkeley noted the difficulty DALL-E has in handling reflections and shadows; the same month, a study from Korea investigated the ‘uniqueness' and originality of DALL-E 2-style output with a critical eye; a preliminary analysis of DALL-E 2 images, shortly after launch, from NYU and the University of Texas, found various issues with compositionality and other essential factors in DALL-E 2 images; and last month, a joint work between the University of Illinois and MIT offered suggestions for architectural improvements to such systems in terms of compositionality.
The researchers further note that DALL-E luminaries such as Aditya Ramesh have conceded the framework's issues with binding, relative size, text, and other challenges.
The developers behind Google's rival image synthesis system Imagen have also proposed DrawBench, a novel comparison system that gauges image accuracy across frameworks with diverse metrics.
Instead, the new paper's authors suggest that a better result might be obtained by pitting human estimation – rather than internecine, algorithmic metrics – against the resulting images, to establish where the weaknesses lie, and what could be done to mitigate them.
The Study
To this end, the new project bases its approach on psychological principles, and seeks to retreat from the current surge of interest in prompt engineering (which is, in effect, a concession to the shortcomings of DALL-E 2, or any comparable system), to investigate and potentially address the limitations that make such ‘workarounds' necessary.
The paper states:
‘The current work focuses on a set of 15 basic relations previously described, examined, or proposed in the cognitive, developmental, or linguistic literature. The set contains both grounded spatial relations (e.g. ’X on Y’), and more abstract agentic relations (e.g. ’X helping Y’).
‘The prompts are intentionally simple, without attribute complexity or elaboration. That is, instead of a prompt like ‘a donkey and an octopus are playing a game. The donkey is holding a rope on one end, the octopus is holding onto the other. The donkey holds the rope in its mouth. A cat is jumping over the rope’, we use ‘a box on a knife’.
‘The simplicity still captures a broad range of relations from across various subdomains of human psychology, and makes potential model failures more striking and specific.'
For their study, the authors recruited 169 participants from Prolific, all located in the USA, with an average age of 33, and 59% female.
The participants were shown 18 images organized into a 3×6 grid with the prompt at the top, and a disclaimer at the bottom stating that all, some or none of the images may have been generated from the displayed prompt, and were then asked to select the images that they thought were related in this way.
The images presented to the individuals were based on linguistic, developmental and cognitive literature, comprising a set of eight physical and seven ‘agentic' relations (this will become clear in a moment).
Physical relations
in, on, under, covering, near, occluded by, hanging over, and tied to.
Agentic Relations
pushing, pulling, touching, hitting, kicking, helping, and hindering.
All of these relations were drawn from the previous mentioned non-CS fields of study.
Twelve entities were thus derived for use in the prompts, with six objects and six agents:
Objects
box, cylinder, blanket, bowl, teacup, and knife.
Agents
man, woman, child, robot, monkey, and iguana.
(The researchers concede that including the iguana, not a mainstay of dry sociological or psychological research, was ‘a treat')
For each relation, five different prompts were created by randomly sampling two entities five times, resulting in 75 total prompts, each of which was submitted to DALL-E 2, and for each of which the initial 18 supplied images were used, with no variations or second chances allowed.
Results
The paper states*:
‘Participants on average reported a low amount of agreement between DALL-E 2’s images and the prompts used to generate them, with a mean of 22.2% [18.3, 26.6] across the 75 distinct prompts.
‘Agentic prompts, with a mean of 28.4% [22.8, 34.2] across 35 prompts, generated higher agreement than physical prompts, with a mean of 16.9% [11.9, 23.0] across 40 prompts.'
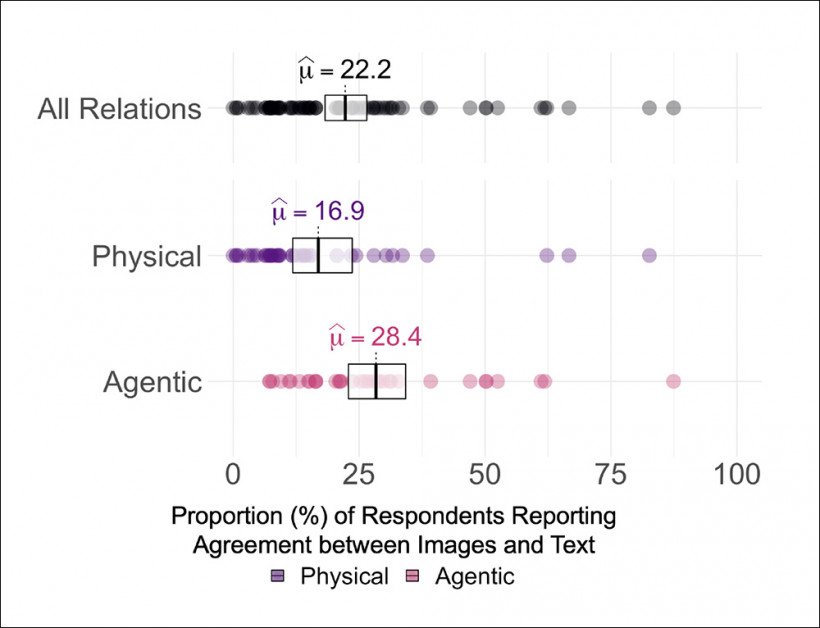
Results from the study. Points in black denote all prompts, with each point an individual prompt, and color breaks down according to whether the prompt subject was agentic or physical (i.e. an object).
To compare the difference between human and algorithmic perception of the images, the researchers ran their renders through OpenAI's open source ViT-L/14 CLIP-based framework. Averaging the scores, they found a ‘moderate relationship' between the two sets of results, which is perhaps surprising, considering the extent to which CLIP itself helps to generate the images.
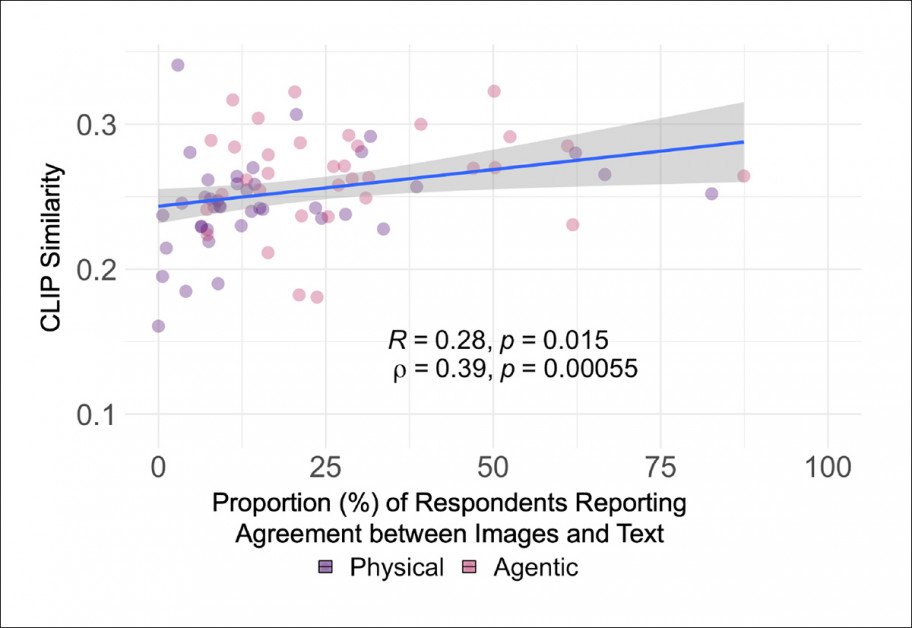
Results of the CLIP (ViT-L/14) comparison against human responses.
The researchers suggest that other mechanisms within the architecture, perhaps combined with a happenstance preponderance (or lack) of data in the training set may account for the way that CLIP can recognize DALL-E's limitations without being able, in all cases, to do anything much about the problem.
The authors conclude that DALL-E 2 only has a notional facility, if any, to reproduce images which incorporate relational understanding, a fundamental facet of human intelligence which develops in us very early.
‘The notion that systems like DALL-E 2 do not have compositionality may come as a surprise to anyone that has seen DALL-E 2’s strikingly reasonable responses to prompts like ‘a cartoon of a baby daikon radish in a tutu walking a poodle’. Prompts such as these often generate a sensible approximation of a compositional concept, with all parts of the prompts present, and present in the right places.
‘Compositionality, however, is not only the ability to glue things together – even things you may never have observed together before. Compositionality requires an understanding of the rules that bind things together. Relations are such rules.'
Man Bites T-Rex
Opinion As OpenAI embraces a greater number of users after its recent beta monetization of DALL-E 2, and since one now has to pay for most of the generations, the shortcomings in DALL-E 2's relational understanding may become more apparent as each ‘failed' attempt has a financial weight to it, and refunds are not available.
Those of us who received an invite a little earlier have had time (and, until recently, greater leisure to play with the system) to observe some of the ‘relationship glitches' that DALL-E 2 can emit.
For instance, for a Jurassic Park fan, it is very difficult to get a dinosaur to chase a person in DALL-E 2, even though the concept of ‘chase' does not appear to be in the DALL-E 2 censorship system, and even though the long history of dinosaur movies should provide abundant training examples (at least in the form of trailers and publicity shots) for this otherwise impossible meeting of species.
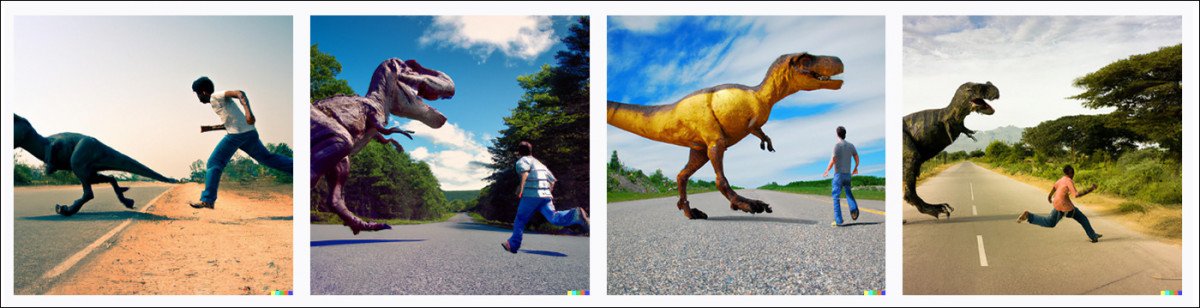
A typical DALL-E 2 response to the prompt ‘A color photo of a T-Rex chasing a man down a road'. Source: DALL-E 2
I've found that the images above are typical for variations on the ‘[dinosaur] chasing [a person]' prompt design, and that no amount of elaboration in the prompt can get the T-Rex to actually comply. In the first and second photos, the man is (more or less) chasing the T-Rex; in the third, approaching it with a casual disregard for safety; and in the final image, apparently jogging in parallel to the great beast. Across about 10-15 attempts at this theme, I've found that the dinosaur is similarly ‘distracted'.
It could be that the only training data that DALL-E 2 could access was in the line of ‘man fights dinosaur', from publicity shots for older movies such as One Million Years B.C. (1966), and that Jeff Goldblum's famous flight from the king of predators is simply an outlier in that small tranche of data.
* My conversion of the authors' inline citations to hyperlinks.
First published 4th August 2022.
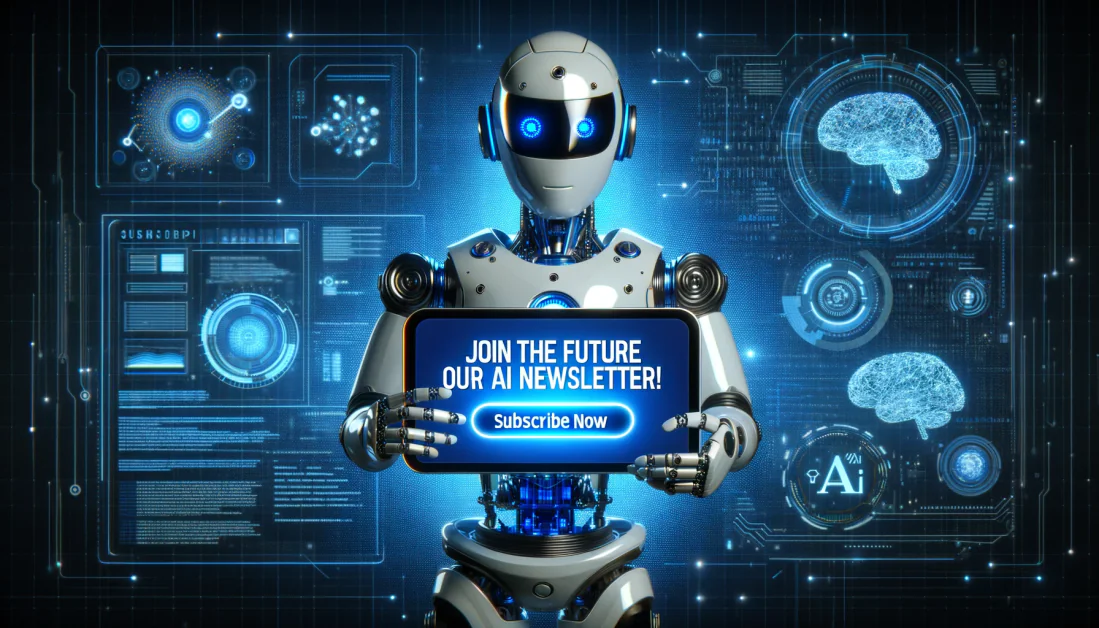
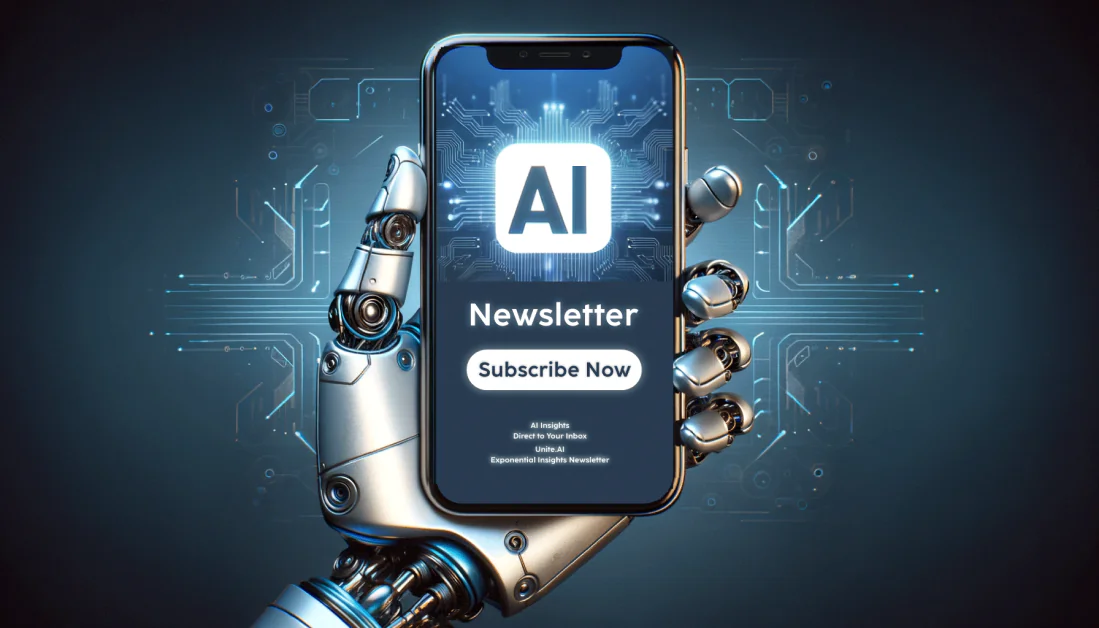