Artificial Intelligence
Plastic Surgery for GAN-Generated Faces
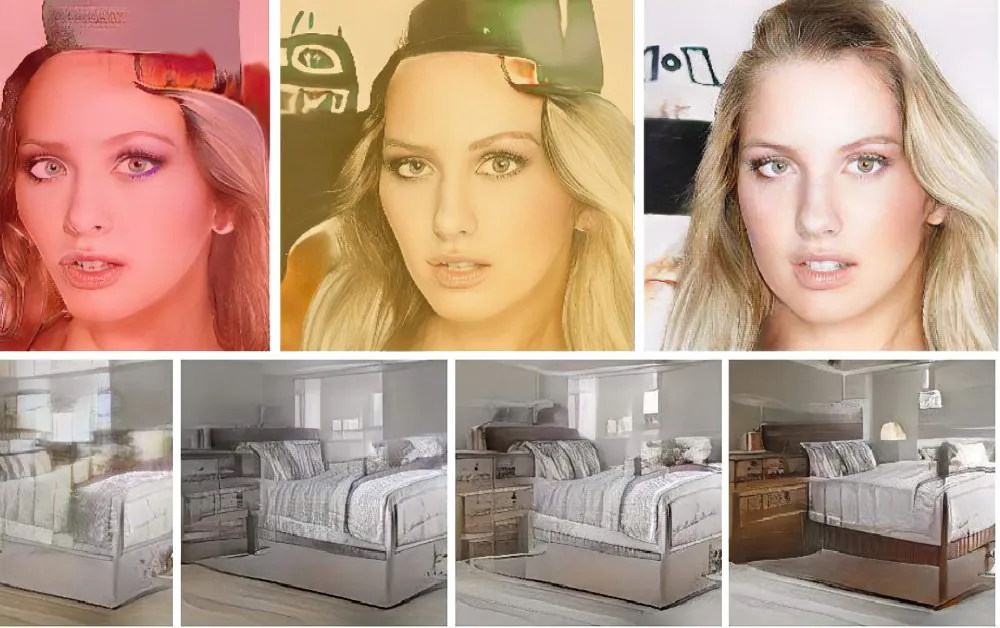
New research out of South Korea promises to improve the quality of synthetic face data created by Generative Adversarial Networks (GANs).
The system is capable of identifying image artifacts produced by GAN processes, and remediating them, even to the point of replacing hair that was obscured by a cap, replacing parts of the face entirely missing in the original, and removing occlusions such as hands and sunglasses, and also works well on scenic and architectural output.
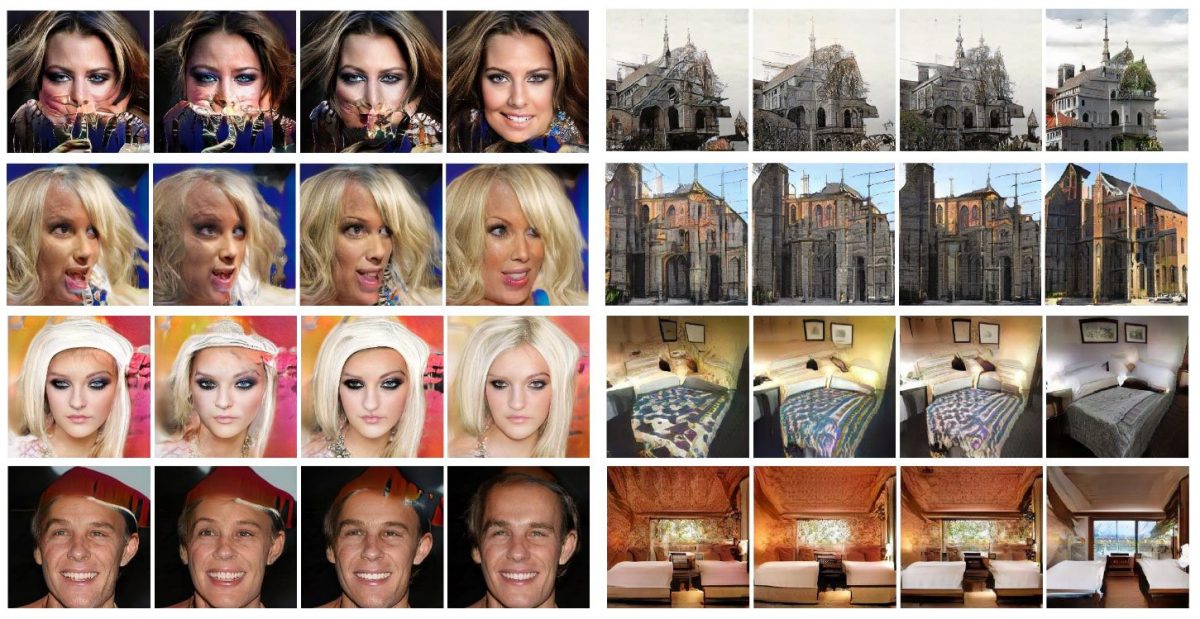
Left for each column, the original GAN output with defects, followed by two other approaches to the artifacts, and finally by the method used by the South Korean researchers. Source: https://arxiv.org/pdf/2104.06118.pdf
Most recent approaches to improving the quality of GAN-generated images have taken the stance that artifacts are an occupational hazard of the process, treating the methodology as a ‘force of nature', and the more psychedelic or aberrational results that it can produce as an inevitable by-product.
Instead, the South Korean research proposes actually ‘fixing' affected images in a way that doesn't interfere with the continued generative chain, by identifying facets that are causing artifacts, and reducing or eliminating their influence in the GAN network at a semi-supervised level that exceeds and extends the native self-correcting mechanisms in GAN architecture.
For the project, it was necessary to create a widely-applicable hand-labeled dataset of images that were badly affected by GAN artifacts. Initially, the researchers used Frechet Inception Distance (FID), a metric that evaluates the quality of GAN output by comparing features in the images, as a qualifying unit. The 10,000 images with the highest FID scores among a run of 200,000 images were used as discrete ‘artifact units'. Subsequently the researchers hand-labeled 2,000 generated images, classifying each as ‘normal' or affected by FID artifacts. Then a model was created to classify the dataset into artifact, normal, and random real-world samples.
After this, Gradient-weighted Class Activation Mapping (Grad-CAM) was used to generate masks for artifact-affected regions, effectively automating the labeling of defects.
In the image above, the Grad-CAM masks have been applied to output from the LSUN-Church outdoor dataset, and the CelebA-HQ dataset.
By analyzing the top 20 most afflicted results from a run of 20,000 images, segmentation masks are generated, into which area representative results across the generations (which are likely to be more accurate or convincing than the artifacts) can be substituted by lowering the activation of the artifact-producing units in subsequent generations.
Human evaluation of the corrections resulted in 53% of the ‘repaired' images being labeled as ‘normal', while 97% of the original images still demonstrate significant improvements on the originals.
The researchers contend that this method, with some minor re-tooling, can also be adapted to NVIDIA's StyleGAN2.
The Benefits Of Synthetic Data
Primarily in regard to face data, the general scarcity of real world datasets for computer vision is an obstacle to diverse research in important research sectors, such as facial recognition, emotion recognition, medical research, and studies into more granular segmentation of the topology of the face, among various other fields.
The current backlash towards free use of web-facing data and the ad hoc collection of real-world face images for inclusion in face databases is an additional obstacle to research, with a growing number of states and nations cracking down on web-scraping, and the appropriation of social media images for these purposes.
Over the last ten years, a limited number of highly curated face datasets have offered refuge from this kind of uncertainty, with various annual public research challenges centered around them. However, this has arguably led to research projects skewing their methodologies specifically towards those datasets, with consistent and comparable year-on-year results obtained at the high cost of a lack of diversity in the source material – a situation that gets worse every year that new research limits itself to these confines.
Additionally, some of these ‘traditional' datasets have come under criticism for a lack of racial diversity, which suggests that these benchmarking stalwarts may not be considered suitable resources in the near future.
This signifies the need for high quality face data that is realistic, but where the contributing ‘real world' images have been transformed far beyond recognition. Even if this use of real face data ‘at one remove' may itself eventually trigger issues over the provenance of GAN-generated faces, it's a stumbling block that's not likely to crop up until the advent of legal and technical mechanisms for data-gathering of this kind have become established; and, regarding possible changes to legal frameworks around the issue, it's still a lesser hazard than using images of real people.
Further reading:
Improving the Realism of Synthetic Images
Automatic Correction of Internal Units in Generative Neural Networks
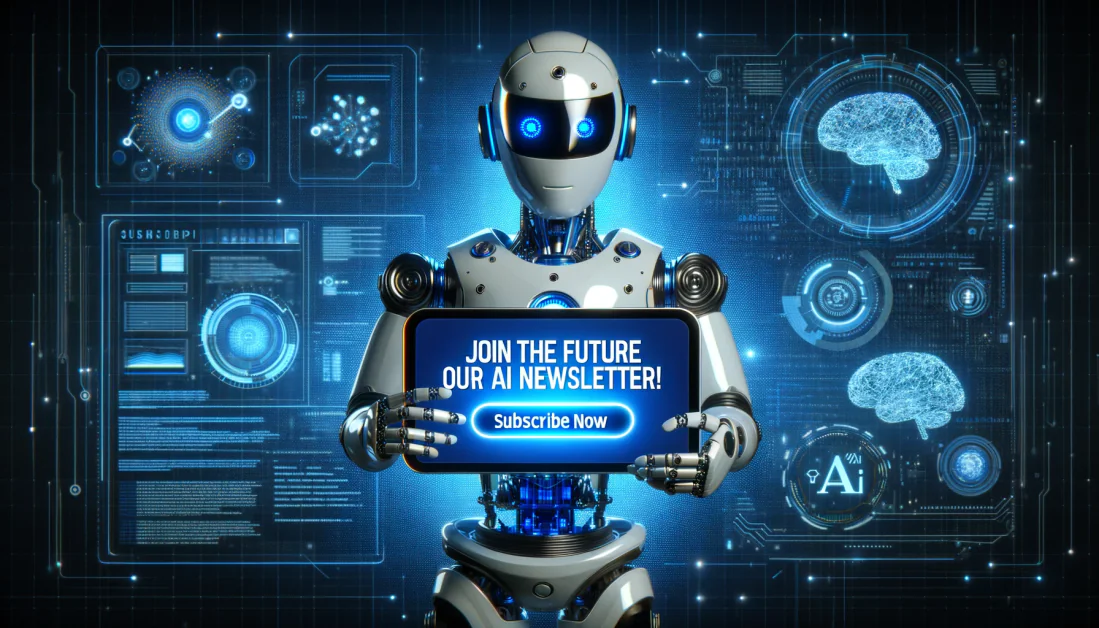
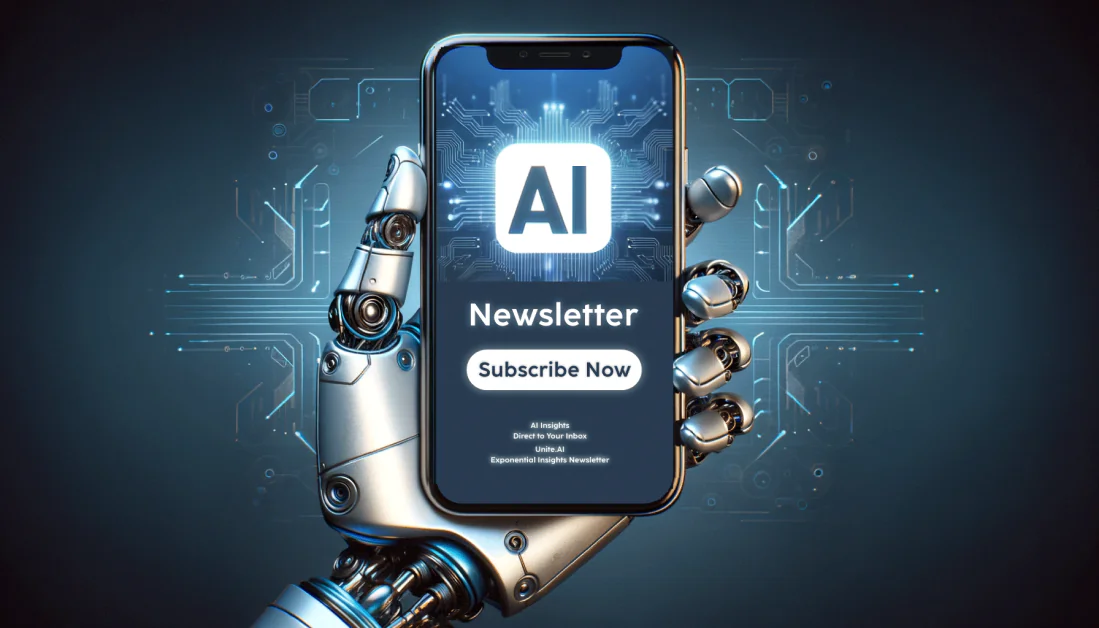