Robotics
Human-guided AI Framework Promises Quicker Robotic Learning in Novel Environments
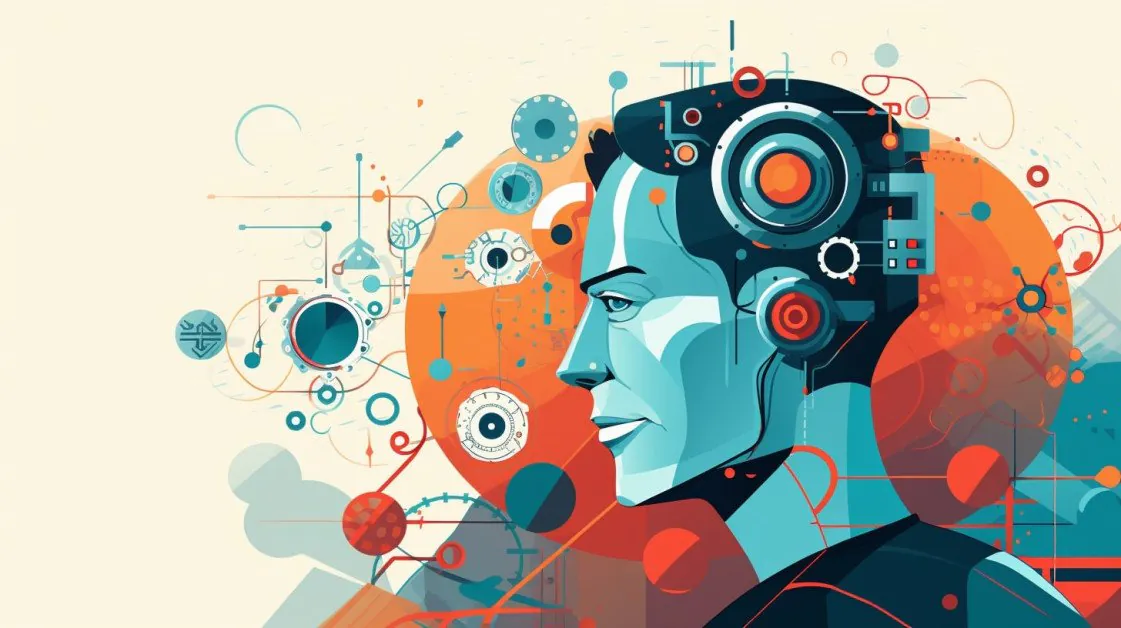
In the future era of smart homes, acquiring a robot to streamline household tasks will not be a rarity. Nevertheless, frustration could set in when these automated helpers fail to perform straightforward tasks. Enter Andi Peng, a scholar from MIT's Electrical Engineering and Computer Science department, who, along with her team, is crafting a path to improve the learning curve of robots.
Peng and her interdisciplinary team of researchers have pioneered a human-robot interactive framework. The highlight of this system is its ability to generate counterfactual narratives that pinpoint the changes needed for the robot to perform a task successfully.
To illustrate, when a robot struggles to recognize a peculiarly painted mug, the system offers alternative situations in which the robot would have succeeded, perhaps if the mug were of a more prevalent color. These counterfactual explanations coupled with human feedback streamline the process of generating new data for the fine-tuning of the robot.
Peng explains, “Fine-tuning is the process of optimizing an existing machine-learning model that is already proficient in one task, enabling it to carry out a second, analogous task.”
A Leap in Efficiency and Performance
When put to the test, the system showed impressive results. Robots trained under this method showcased swift learning abilities, while reducing the time commitment from their human teachers. If successfully implemented on a larger scale, this innovative framework could help robots adapt rapidly to new surroundings, minimizing the need for users to possess advanced technical knowledge. This technology could be the key to unlocking general-purpose robots capable of assisting elderly or disabled individuals efficiently.
Peng believes, “The end goal is to empower a robot to learn and function at a human-like abstract level.”
Revolutionizing Robot Training
The primary hindrance in robotic learning is the ‘distribution shift,' a term used to explain a situation when a robot encounters objects or spaces it hasn't been exposed to during its training period. The researchers, to address this problem, implemented a method known as ‘imitation learning.' But it had its limitations.
“Imagine having to demonstrate with 30,000 mugs for a robot to pick up any mug. Instead, I prefer to demonstrate with just one mug and teach the robot to understand that it can pick up a mug of any color,” Peng says.
In response to this, the team's system identifies which attributes of the object are essential for the task (like the shape of a mug) and which are not (like the color of the mug). Armed with this information, it generates synthetic data, altering the “non-essential” visual elements, thereby optimizing the robot's learning process.
Connecting Human Reasoning with Robotic Logic
To gauge the efficacy of this framework, the researchers conducted a test involving human users. The participants were asked whether the system's counterfactual explanations enhanced their understanding of the robot's task performance.
Peng says, “We found humans are inherently adept at this form of counterfactual reasoning. It's this counterfactual element that allows us to translate human reasoning into robotic logic seamlessly.”
In the course of multiple simulations, the robot consistently learned faster with their approach, outperforming other techniques and needing fewer demonstrations from users.
Looking ahead, the team plans to implement this framework on actual robots and work on shortening the data generation time via generative machine learning models. This breakthrough approach holds the potential to transform the robot learning trajectory, paving the way for a future where robots harmoniously co-exist in our day-to-day life.
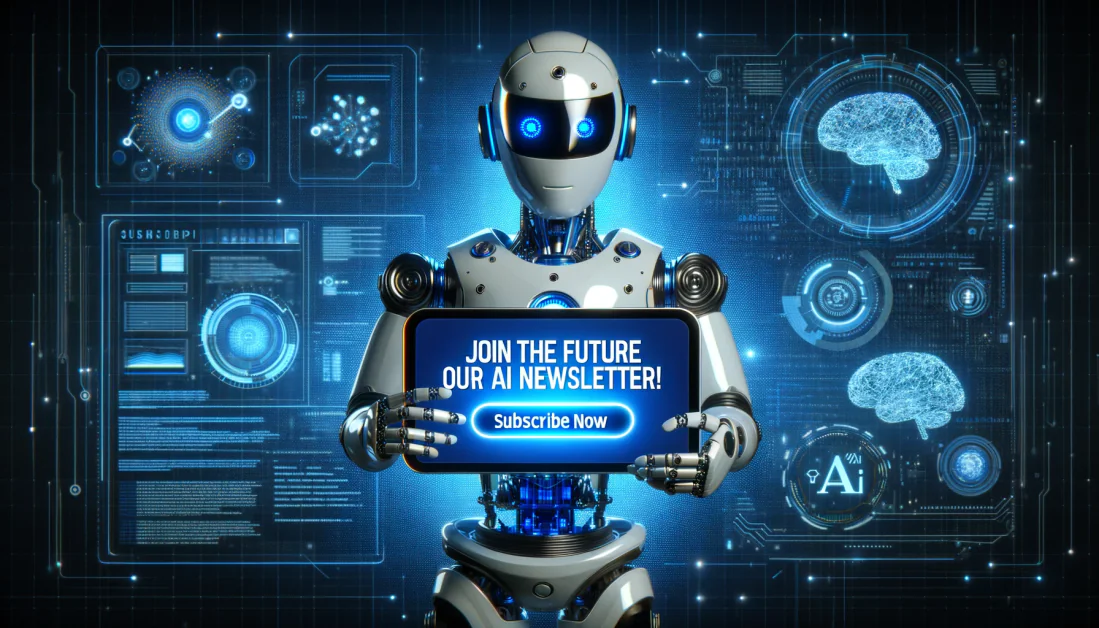
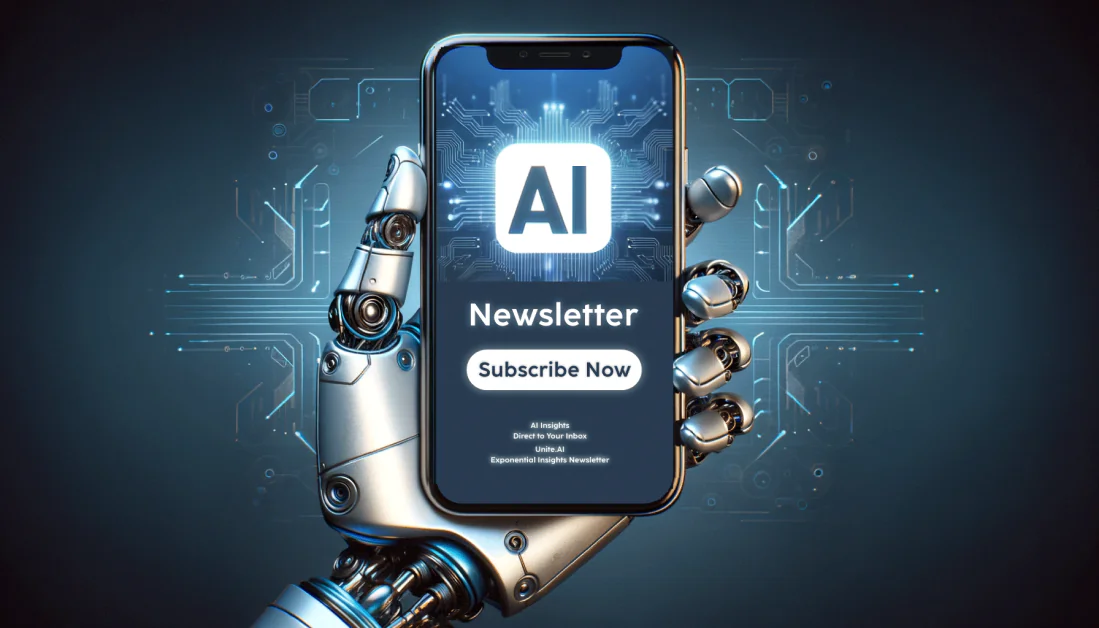