Thought Leaders
How Bias Will Kill Your AI/ML Strategy and What to Do About It
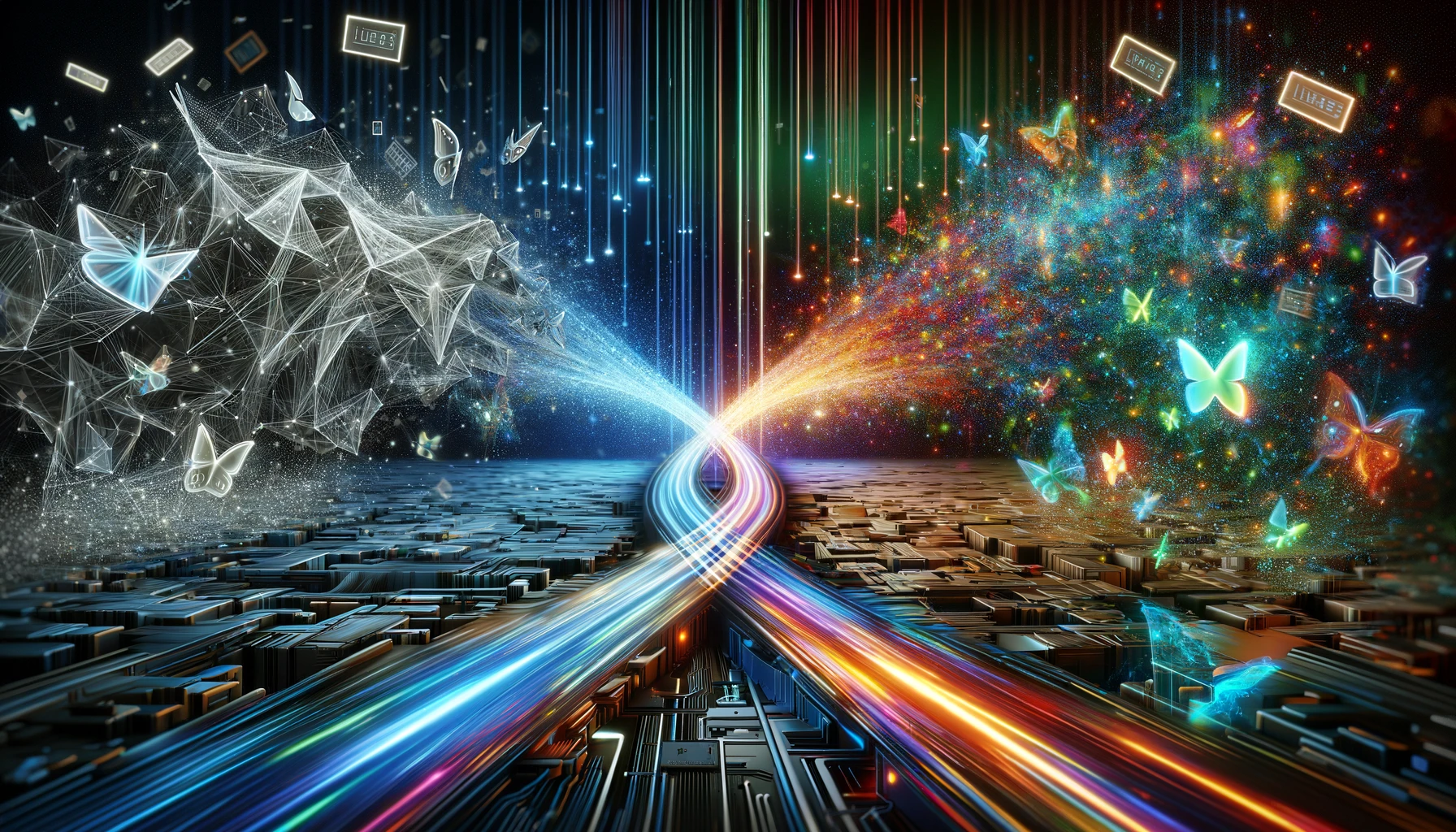
‘Bias’ in models of any type describes a situation in which the model responds inaccurately to prompts or input data because it hasn’t been trained with enough high-quality, diverse data to provide an accurate response. One example would be Apple’s facial recognition phone unlock feature, which failed at a significantly higher rate for people with darker skin complexions as opposed to lighter tones. The model hadn’t been trained on enough images of darker-skinned people. This was a relatively low-risk example of bias but is exactly why the EU AI Act has put forth requirements to prove model efficacy (and controls) before going to market. Models with outputs that impact business, financial, health, or personal situations must be trusted, or they won’t be used.
Tackling Bias with Data
Large Volumes of High-Quality Data
Among many important data management practices, a key component to overcoming and minimizing bias in AI/ML models is to acquire large volumes of high-quality, diverse data. This requires collaboration with multiple organizations that have such data. Traditionally, data acquisition and collaborations are challenged by privacy and/or IP protection concerns–sensitive data can't be sent to the model owner, and the model owner can’t risk leaking their IP to a data owner. A common workaround is to work with mock or synthetic data, which can be useful but also have limitations compared to using real, full-context data. This is where privacy-enhancing technologies (PETs) provide much-needed answers.
Synthetic Data: Close, but not Quite
Synthetic data is artificially generated to mimic real data. This is hard to do but becoming slightly easier with AI tools. Good quality synthetic data should have the same feature distances as real data, or it won’t be useful. Quality synthetic data can be used to effectively boost the diversity of training data by filling in gaps for smaller, marginalized populations, or for populations that the AI provider simply doesn’t have enough data. Synthetic data can also be used to address edge cases that might be difficult to find in adequate volumes in the real world. Additionally, organizations can generate a synthetic data set to satisfy data residency and privacy requirements that block access to the real data. This sounds great; however, synthetic data is just a piece of the puzzle, not the solution.
One of the obvious limitations of synthetic data is the disconnect from the real world. For example, autonomous vehicles trained solely on synthetic data will struggle with real, unforeseen road conditions. Additionally, synthetic data inherits bias from the real-world data used to generate it–pretty much defeating the purpose of our discussion. In conclusion, synthetic data is a useful option for fine tuning and addressing edge cases, but significant improvements in model efficacy and minimization of bias still rely upon accessing real world data.
A Better Way: Real Data via PETs-enabled Workflows
PETs protect data while in use. When it comes to AI/ML models, they can also protect the IP of the model being run–”two birds, one stone.” Solutions utilizing PETs provide the option to train models on real, sensitive datasets that weren't previously accessible due to data privacy and security concerns. This unlocking of dataflows to real data is the best option to reduce bias. But how would it actually work?
For now, the leading options start with a confidential computing environment. Then, an integration with a PETs-based software solution that makes it ready to use out of the box while addressing the data governance and security requirements that aren’t included in a standard trusted execution environment (TEE). With this solution, the models and data are all encrypted before being sent to a secured computing environment. The environment can be hosted anywhere, which is important when addressing certain data localization requirements. This means that both the model IP and the security of input data are maintained during computation–not even the provider of the trusted execution environment has access to the models or data inside of it. The encrypted results are then sent back for review and logs are available for review.
This flow unlocks the best quality data no matter where it is or who has it, creating a path to bias minimization and high-efficacy models we can trust. This flow is also what the EU AI Act was describing in their requirements for an AI regulatory sandbox.
Facilitating Ethical and Legal Compliance
Acquiring good quality, real data is tough. Data privacy and localization requirements immediately limit the datasets that organizations can access. For innovation and growth to occur, data must flow to those who can extract the value from it.
Art 54 of the EU AI Act provides requirements for “high-risk” model types in terms of what must be proven before they can be taken to market. In short, teams will need to use real world data inside of an AI Regulatory Sandbox to show sufficient model efficacy and compliance with all the controls detailed in Title III Chapter 2. The controls include monitoring, transparency, explainability, data security, data protection, data minimization, and model protection–think DevSecOps + Data Ops.
The first challenge will be to find a real-world data set to use–as this is inherently sensitive data for such model types. Without technical guarantees, many organizations may hesitate to trust the model provider with their data or won’t be allowed to do so. In addition, the way the act defines an “AI Regulatory Sandbox” is a challenge in and of itself. Some of the requirements include a guarantee that the data is removed from the system after the model has been run as well as the governance controls, enforcement, and reporting to prove it.
Many organizations have tried using out-of-the-box data clean rooms (DCRs) and trusted execution environments (TEEs). But, on their own, these technologies require significant expertise and work to operationalize and meet data and AI regulatory requirements.
DCRs are simpler to use, but not yet useful for more robust AI/ML needs. TEEs are secured servers and still need an integrated collaboration platform to be useful, quickly. This, however, identifies an opportunity for privacy enhancing technology platforms to integrate with TEEs to remove that work, trivializing the setup and use of an AI regulatory sandbox, and therefore, acquisition and use of sensitive data.
By enabling the use of more diverse and comprehensive datasets in a privacy-preserving manner, these technologies help ensure that AI and ML practices comply with ethical standards and legal requirements related to data privacy (e.g., GDPR and EU AI Act in Europe). In summary, while requirements are often met with audible grunts and sighs, these requirements are simply guiding us to building better models that we can trust and rely upon for important data-driven decision making while protecting the privacy of the data subjects used for model development and customization.
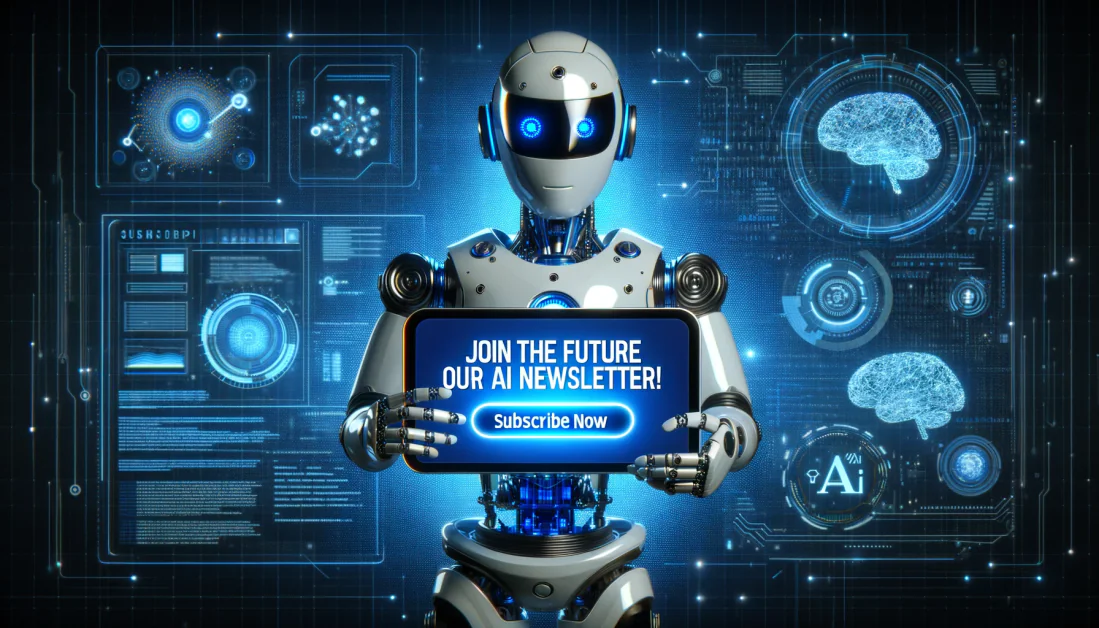
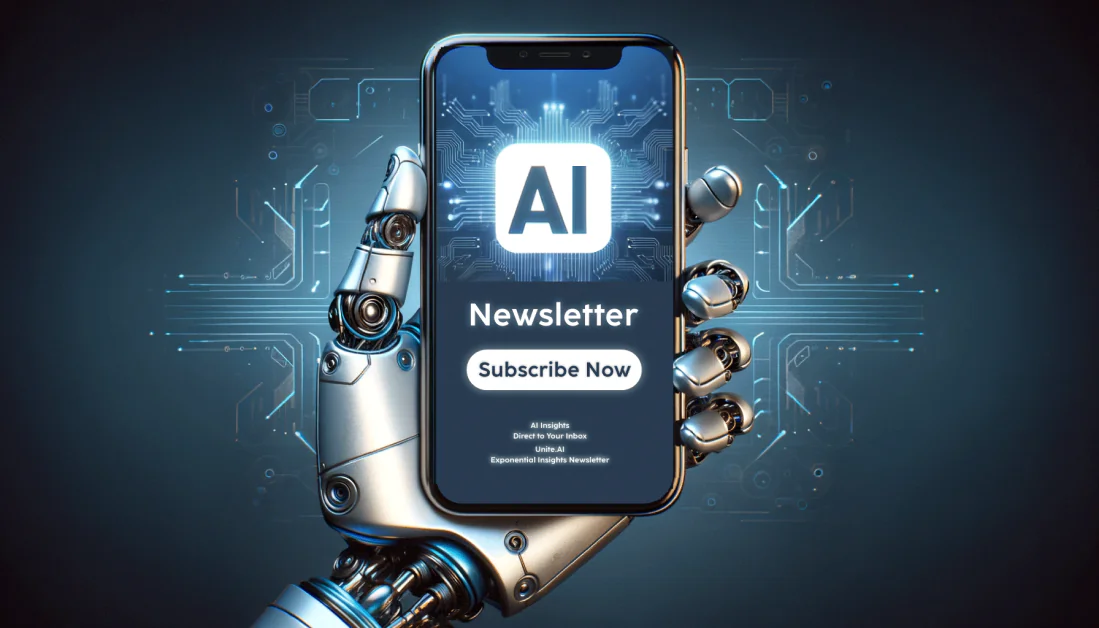