Thought Leaders
How AI and ML Are Scaling Data Collection to Transform Medical Monitoring
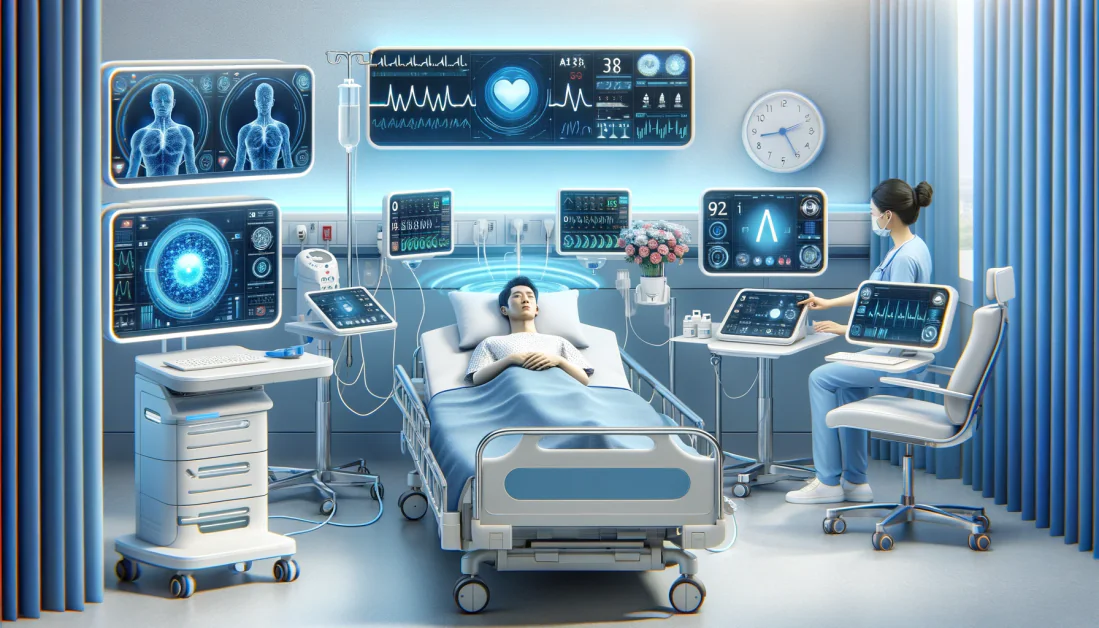
Artificial intelligence (AI) and machine learning (ML) can be found in nearly every industry, driving what some consider a new age of innovation – particularly in healthcare, where it is estimated the role of AI will grow at a 50% rate annually by 2025. ML is increasingly playing a vital role in assisting with diagnoses, imaging, predictive health, and more.
With new medical devices and wearables in the market, ML has the capability to transform medical monitoring by collecting, analyzing, and delivering easily accessible information for people to better manage their own health – improving the likelihood for the early detection or prevention of chronic diseases. There are several factors researchers should keep in mind when developing these novel technologies to ensure they are collecting the highest quality data and building scalable, accurate, and equitable ML algorithms fit for real-world use cases.
Using ML to scale clinical research and data analysis
Over the last 25 years, the development of medical devices has accelerated, especially during the COVID-19 pandemic. We’re starting to see more consumer devices such as fitness trackers and wearables commoditize, and development shift to medical diagnostic devices. As these devices are brought to market, their capabilities continue to evolve. More medical devices means more continuous data and larger, more diverse data sets that need to be analyzed. This processing can be tedious and inefficient when done manually. ML enables extensive datasets to be analyzed faster and with more accuracy, identifying patterns that can lead to transformative insights.
With all this data now at our fingertips, we must ensure first and foremost that we are processing the right data. Data shapes and informs the technology that we use, but not all data provides the same benefit. We need high-quality, continuous, unbiased data, with the right data collection methods supported by gold-standard medical references as a comparative baseline. This ensures we are building safe, equitable, and accurate ML algorithms.
Ensuring equitable system development in the medical device space
When developing algorithms, researchers and developers must consider their intended populations more broadly. It’s not uncommon for most companies to conduct studies and clinical trials in a singular, ideal, non-real-world instance. However, it is critical that developers consider all real-world use cases for the device, and all the possible interactions their intended population could have with the technology on a day-to-day basis. We ask: who is the intended population for the device, and are we factoring in the entire population? Does everyone in the targeted audience have equitable access to the technology? How will they interact with the technology? Will they be interacting with the technology 24/7 or intermittently?
When developing medical devices that are going to integrate into someone’s daily life, or potentially intervene with daily behaviors, we also need to factor in the whole person – mind, body, and environment – and how these components may change over time. Every human presents a unique opportunity, with variations at different points throughout the day. Understanding time as a component in data collection allows us to amplify the insights we generate.
By factoring in these elements and understanding all components of physiology, psychology, background, demographics, and environmental data, researchers and developers can ensure they are collecting high-resolution, continuous data that enables them to build accurate and strong models for human health applications.
How ML can transform diabetes management
These ML best practices will be particularly transformative in the diabetes management space. The diabetes epidemic is rapidly growing around the globe: 537M people worldwide live with Type 1 and Type 2 diabetes and that number is expected to grow to 643M by 2030. With so many impacted, it’s imperative that patients have access to a solution that shows them what is happening inside their own body and allows them to effectively manage their conditions.
In recent years, in response to the epidemic, researchers and developers have begun exploring non-invasive methods of measuring blood glucose, such as optical sensing techniques. These methods, however, have known limitations due to varying human factors such as melanin levels, BMI levels, or skin thickness.
Radiofrequency (RF) sensing technology overcomes the limitations of optical sensing and has the potential to transform the way people with diabetes and prediabetes manage their health. This technology offers a more reliable solution when it comes to non-invasively measuring blood glucose due to its ability to generate large amounts of data and safely measure through the full tissue stack.
RF sensor technology allows for data collection across several hundred thousand frequencies, resulting in billions of data observations to process and requiring powerful algorithms to manage and interpret such large and novel datasets. ML is essential in processing and interpreting the massive amount of novel data generated from this type of sensor technology, enabling faster and more accurate algorithm development – critical to building an effective non-invasive glucose monitor that improves health outcomes across all intended use cases.
In the diabetes space, we’re also seeing a shift from intermittent to continuous data. Finger pricking, for example, provides insights into blood glucose levels at select points throughout the day, but a continuous glucose monitor (CGM) provides insights in more frequent, yet non-continuous increments. These solutions, however, still require puncturing the skin, often resulting in pain and skin sensitivity. A non-invasive blood glucose monitoring solution enables us to capture high-quality continuous data from a broader population with ease and without a lag time in measurement. Overall, this solution would provide an unquestionably better user experience and lower cost over time.
In addition, the high volume of continuous data contributes to the development of more equitable and accurate algorithms. As more time series data is collected, in combination with high resolution data, developers can continue to build better algorithms to increase accuracy in detecting blood glucose over time. This data can fuel continued algorithm improvement as it includes various factors that reflect how people change day-to-day (and throughout a single day), yielding a highly accurate solution. Non-invasive solutions that monitor different vitals can transform the medical monitoring industry and provide a deeper look into how the human body works through continuous data from diverse patient populations.
Medical devices creating an interconnected system
As technology advances and medical device systems achieve even higher levels of accuracy, patients and consumers are seeing more and more opportunities to take control of their own daily health through advanced and multi-modal data from a variety of products. But in order to see the most impact from medical device and wearables data, there needs to be an interconnected system to create a smooth exchange of data across multiple devices in order to provide a holistic view of an individual’s health.
Prioritizing medical device interoperability will unlock the full capability of these devices to help manage chronic conditions, such as diabetes. A seamless flow and exchange of information between devices such as insulin pumps and CGMs will allow individuals to have a better understanding of their diabetes management system.
High-fidelity data has the potential to transform the healthcare industry when collected and used correctly. With the help of AI and ML, medical devices can make measurable developments within remote patient monitoring by treating individuals as individuals, and understanding a person’s health on a deeper level. ML is the key to unlocking insights from data to inform predictive and preventative health management protocols and empower patients with access to information on their own health, transforming the way data is used.
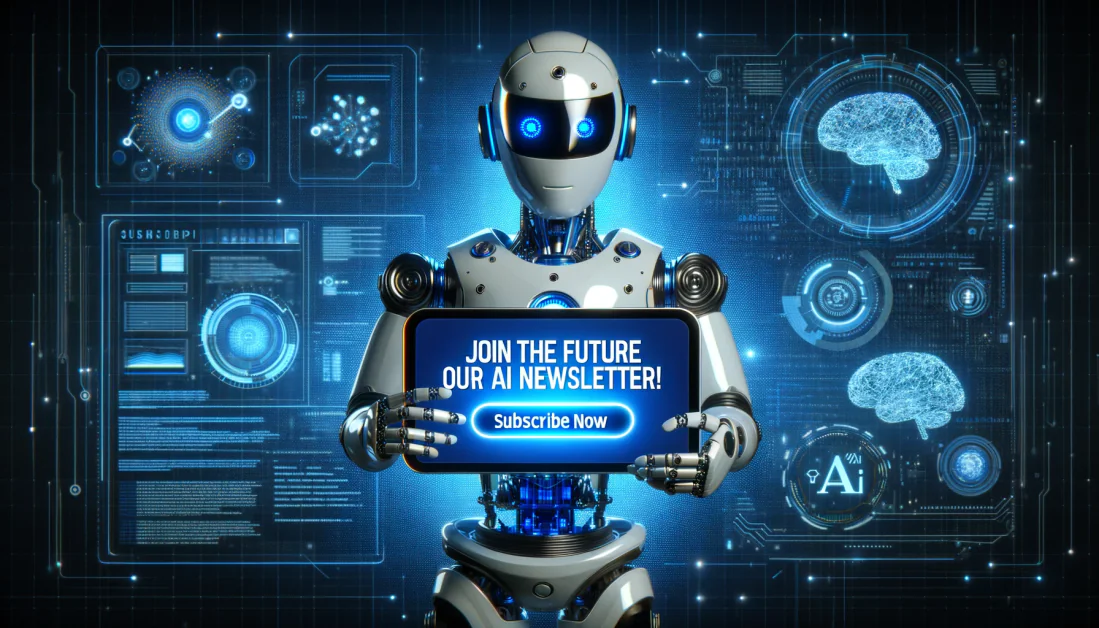
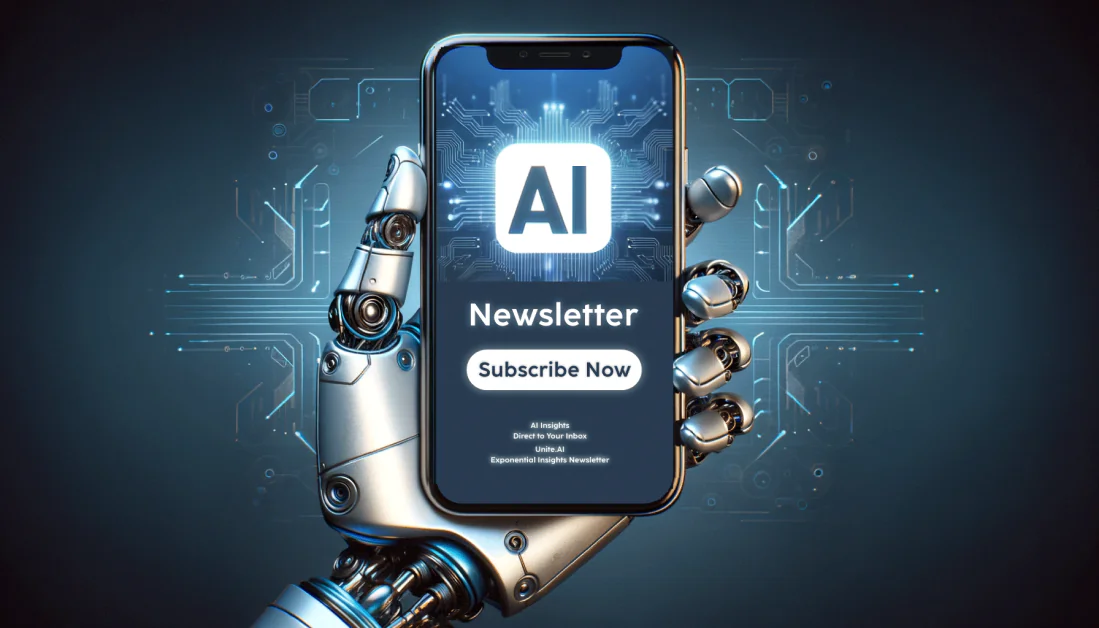