Artificial Intelligence
GOTCHA– A CAPTCHA System for Live Deepfakes
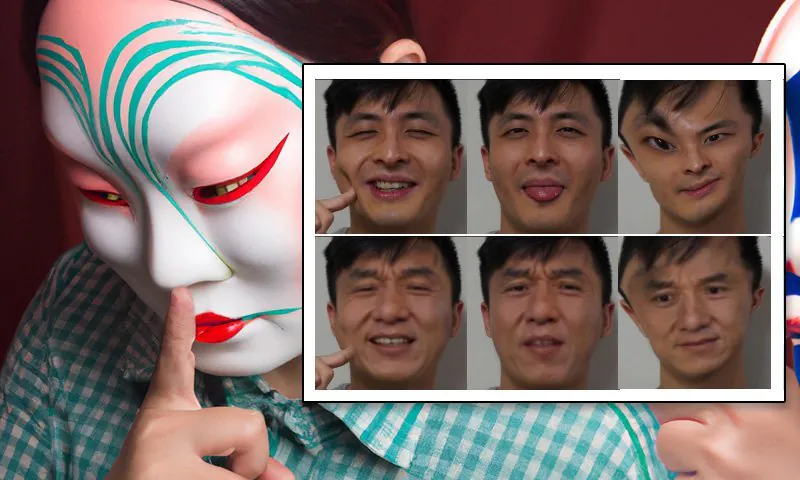
New research from New York University adds to the growing indications that we may soon have to take the deepfake equivalent of a ‘drunk test' in order to authenticate ourselves, before commencing a sensitive video call – such as a work-related videoconference, or any other sensitive scenario that may attract fraudsters using real-time deepfake streaming software.
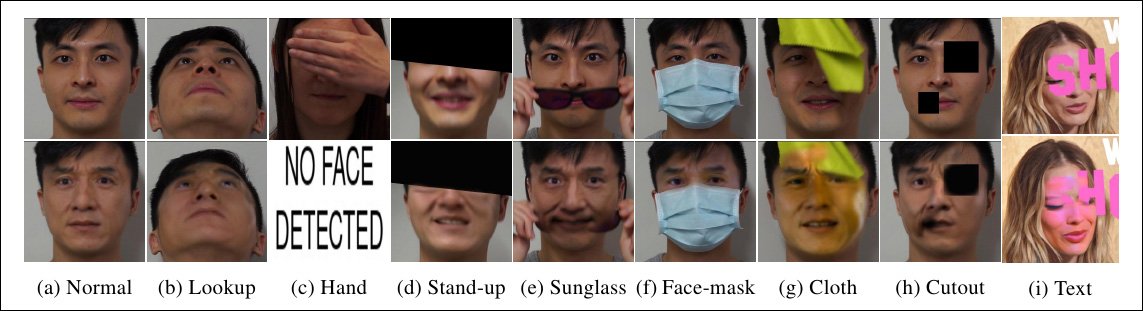
Some of the active and passive challenges applied to video-call scenarios in GOTCHA. The user must comply with and pass the challenges, while additional ‘passive' methods (such as attempting to overload a potential deepfake system) are used over which the participant has no influence. Source: http://export.arxiv.org/pdf/2210.06186
The proposed system is titled GOTCHA – a tribute to the CAPTCHA systems that have become an increasing obstacle to web-browsing over the last 10-15 years, wherein automated systems require the user to perform tasks that machines are bad at, such as identifying animals or deciphering garbled text (and, ironically, these challenges often turn the user into a free AMT-style outsourced annotator).
In essence, GOTCHA extends the August 2022 DF-Captcha paper from Ben-Gurion University, which was the first to propose making the person at the other end of the call jump through a few visually semantic hoops in order to prove their authenticity.
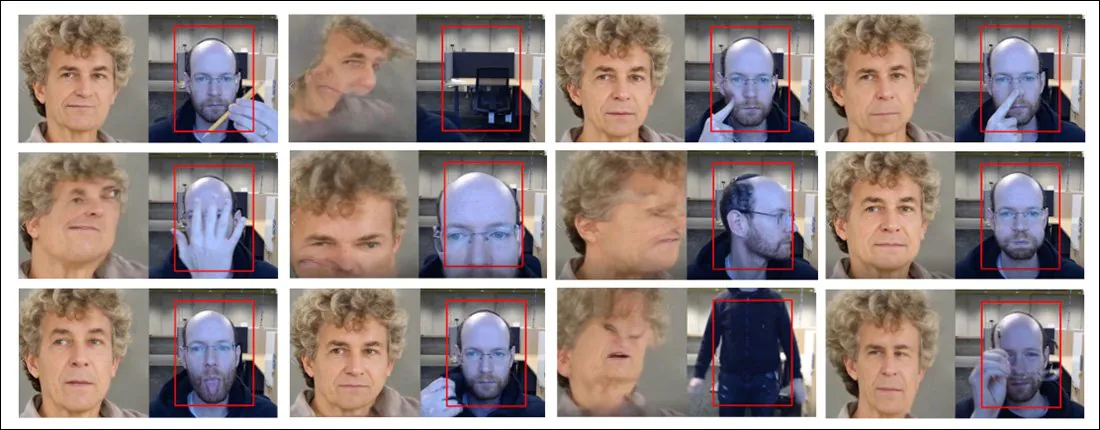
The August 2022 paper from Ben Gurion University first proposed a range of interactive tests for a user, including occluding their face, or even depressing their skin – tasks which even well-trained live deepfake systems may not have anticipated or be able to cope with photorealistically. Source: https://arxiv.org/pdf/2208.08524.pdf
Notably, GOTCHA adds ‘passive' methodologies to a ‘cascade' of proposed tests, including the automatic superimposition of unreal elements over the user's face, and the ‘overloading' of frames going through the source system. However, only the user-responsive tasks can be evaluated without special permissions to access the user's local system – which, presumably, would come in the form of local modules or add-ons to popular systems such as Skype and Zoom, or even in the form of dedicated proprietary software specifically tasked with weeding out fakers.
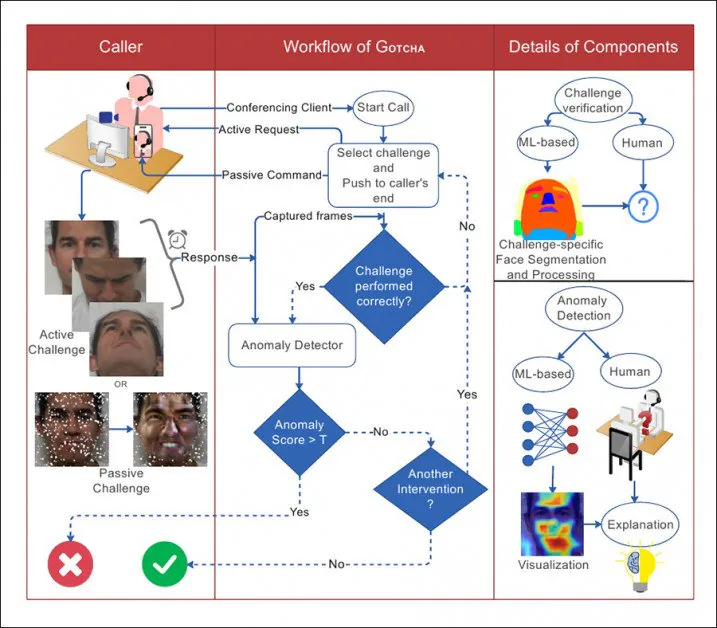
From the paper, an illustration of the interaction between the caller and the system in GOTCHA, with dotted lines as decision flows.
The researchers validated the system on a new dataset containing over 2.5m video-frames from 47 participants, each undertaking 13 challenges from GOTCHA. They claim that the framework induces ‘consistent and measurable' reduction in deepfake content quality for fraudulent users, straining the local system until evident artifacts make the deception clear to the naked human eye (though GOTCHA also contains some more subtle algorithmic analysis methods).
The new paper is titled Gotcha: A Challenge-Response System for Real-Time Deepfake Detection (the system's name is capitalized in the body but not the title of the publication, though it is not an acronym).
A Range of Challenges
Mostly in accordance with the Ben Gurion paper, the actual user-facing challenges are divided into several types of task.
For occlusion, the user is required either to obscure their face with their hand, or with other objects, or to present their face at an angle that is not likely to have been trained into a deepfake model (usually because of a lack of training data for ‘odd' poses – see range of images in the first illustration above).
Besides actions that the user may perform themselves in accordance with instructions, GOTCHA can superimpose random facial cutouts, stickers and augmented reality filters, in order to ‘corrupt' the face-stream that a local trained deepfake model may be expecting, causing it to fail. As indicated before, though this is a ‘passive' process for the user, it is an intrusive one for the software, which needs to be able to intervene directly in the end-correspondent's stream.
Next, the user may be required to pose their face into unusual facial expressions that are likely to either be absent or under-represented in any training dataset, causing a lowering of quality of the deepfaked output (image ‘b', second column from left, in the first illustration above).
As part of this strand of tests, the user may be required to read out text or make conversation that is designed to challenge a local live deepfaking system, which may not have trained an adequate range of phonemes or other types of mouth data to a level where it can reconstruct accurate lip movement under such scrutiny.
Finally (and this one would seem to challenge the acting talents of the end correspondent), in this category, the user may be asked to perform a micro-expression' – a short and involuntary facial expression that belies an emotion. Of this, the paper says ‘[it] usually lasts 0.5-4.0 seconds, and is difficult to fake'.
Though the paper does not describe how to extract a micro-expression, logic suggests that the only way to do it is to create an apposite emotion in the end user, perhaps with some kind of startling content presented to them as part of the test's routine.
Facial Distortion, Lighting, and Unexpected Guests
Additionally, in line with the suggestions from the August paper, the new work proposes asking the end-user to perform unusual facial distortions and manipulations, such as pressing their finger into their cheek, interacting with their face and/or hair, and performing other motions that no current live deepfake system is likely to be able to handle well, since these are marginal actions – even if they were present in the training dataset, their reproduction would likely be of low quality, in line with other ‘outlier' data.
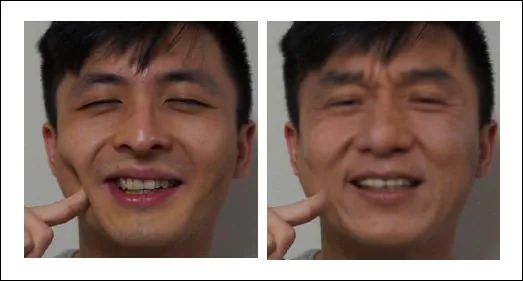
A smile, but this ‘depressed face' is not translated well by a local live deepfake system.
An additional challenge lies in changing the illumination conditions in which the end-user is situated, since it's possible that the training of a deepfake model has been optimized to standard videoconferencing lighting situations, or even the exact lighting conditions that the call is taking place in.
Thus the user may be asked to shine the torch on their mobile phone onto their face, or in some other way alter the lighting (and it’s worth noting that this tack is the central proposition of another live deepfake detection paper that came out this summer).
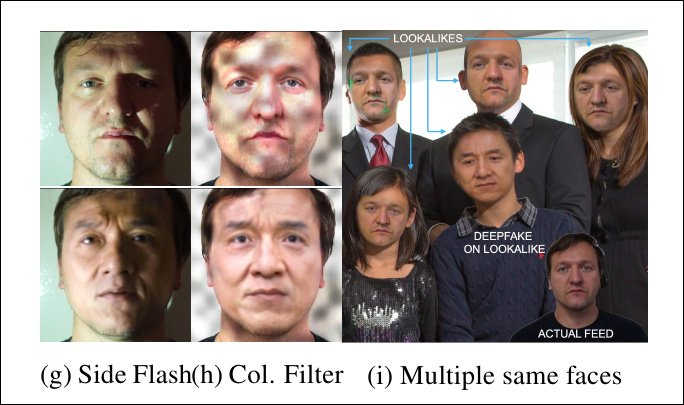
Live deepfake systems are challenged by unexpected lighting – and even by multiple people in the stream, where it was expecting only a single individual.
In the case of the proposed system having the ability to interpose into the local user-stream (which is suspected of harboring a deepfake middleman), adding unexpected patterns (see middle column in image above) can compromise the deepfake algorithm's ability to maintain a simulation.
Additionally, though it is unreasonable to expect a correspondent to have additional people on hand to help authenticate them, the system can interject additional faces (right-most image above), and see if any local deepfake system makes the mistake of switching attention – or even trying to deepfake all of them (autoencoder deepfake systems have no ‘identity recognition' capabilities that could keep attention focused on one individual in this scenario).
Steganography and Overloading
GOTCHA also incorporates an approach first proposed by UC San Diego in April this year, and which uses steganography to encrypt a message into the user's local video stream. Deepfake routines will completely destroy this message, leading to an authentication failure.
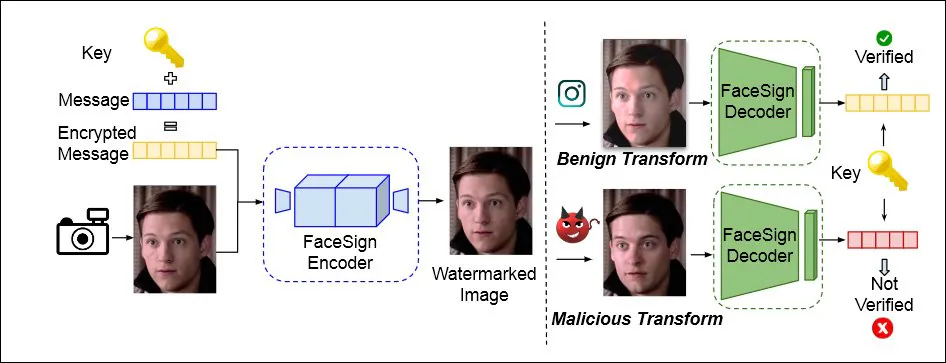
From an April 2022 paper from the University of California San Diego, and San Diego State University, a method of determining authentic identity by seeing if a steganographic signal sent into a user's video stream survives the local loop intact – if it does not, deepfaking chicanery may be at hand. Source: https://arxiv.org/pdf/2204.01960.pdf
Additionally, GOTCHA is capable of overloading the local system (given access and permission), by duplicating a stream and presenting ‘excessive' data to any local system, designed to cause replication failure in a local deepfake system.
The system contains further tests (see the paper for details), including a challenge, in the case of a smartphone-based correspondent, of turning their phone upside down, which will distort a local deepfake system:
Again, this kind of thing would only work with a compelling use case, where the user is forced to grant local access to the stream, and can't be implemented by simple passive evaluation of user video, unlike the interactive tests (such as pressing a finger into one's face).
Practicality
The paper touches briefly on the extent to which tests of this nature may annoy the end user, or else in some way inconvenience them – for example, by obliging the user to have at hand a number of objects that may be needed for the tests, such as sunglasses.
It also acknowledges that it may be difficult to get powerful correspondents to comply with the testing routines. In regard to the case of a video-call with a CEO, the authors state:
‘Usability may be key here, so informal or frivolous challenges (such as facial distortions or expressions) may not be appropriate. Challenges using external physical articles may not be desirable. The context here is appropriately modified and GOTCHA adapts its suite of challenges accordingly.'
Data and Tests
GOTCHA was tested against four strains of local live deepfake system, including two variations on the very popular autoencoder deepfakes creator DeepFaceLab (‘DFL', though, surprisingly, the paper does not mention DeepFaceLive, which has been, since August of 2021, DeepFaceLab's ‘live' implementation, and seems the likeliest initial resource for a potential faker).
The four systems were DFL trained ‘lightly' on a non-famous person participating in tests, and a paired celebrity; DFL trained more fully, to 2m+ iterations or steps, wherein one would expect a much more performant model; Latent Image Animator (LIA); and Face Swapping Generative Adversarial Network (FSGAN).
For the data, the researchers captured and curated the aforementioned video clips, featuring 47 users performing 13 active challenges, with each user outputting around 5-6 minutes of 1080p video at 60fps. The authors state also that this data will eventually be publicly released.
Anomaly detection can be performed either by a human observer or algorithmically. For the latter option, the system was trained on 600 faces from the FaceForensics dataset. The regression loss function was the powerful Learned Perceptual Image Patch Similarity (LPIPS), while binary cross-entropy was used to train the classifier. EigenCam was used to visualize the detector's weights.
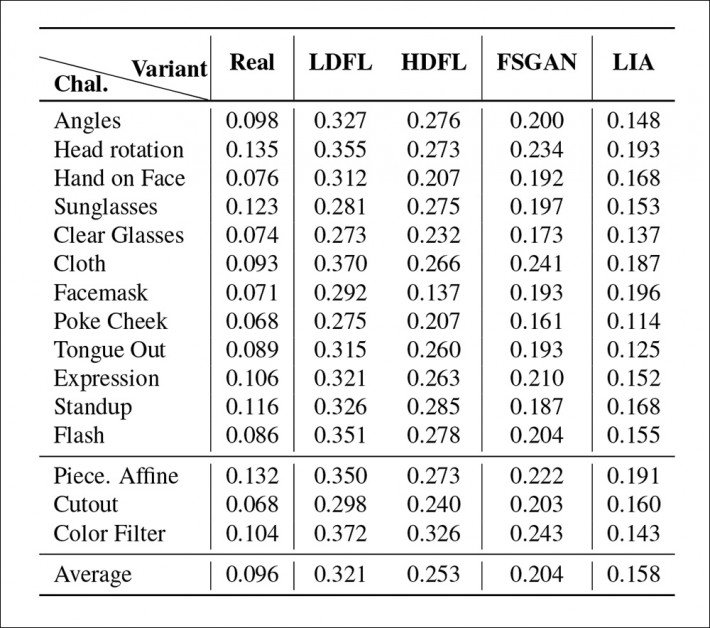
Primary results from the tests for GOTCHA.
The researchers found that for the full cascade of tests across the four systems, the lowest number and severity of anomalies (i.e., artifacts that would reveal the presence of a deepfake system) were obtained by the higher-trained DFL distribution. The lesser-trained version struggled in particular to recreate complex lip movements (which occupy very little of the frame, but which receive high human attention), while FSGAN occupied the middle ground between the two DFL versions, and LIA proved completely inadequate to the task, with the researchers opining that LIA would fail in a real deployment.
First published 17th October 2022.
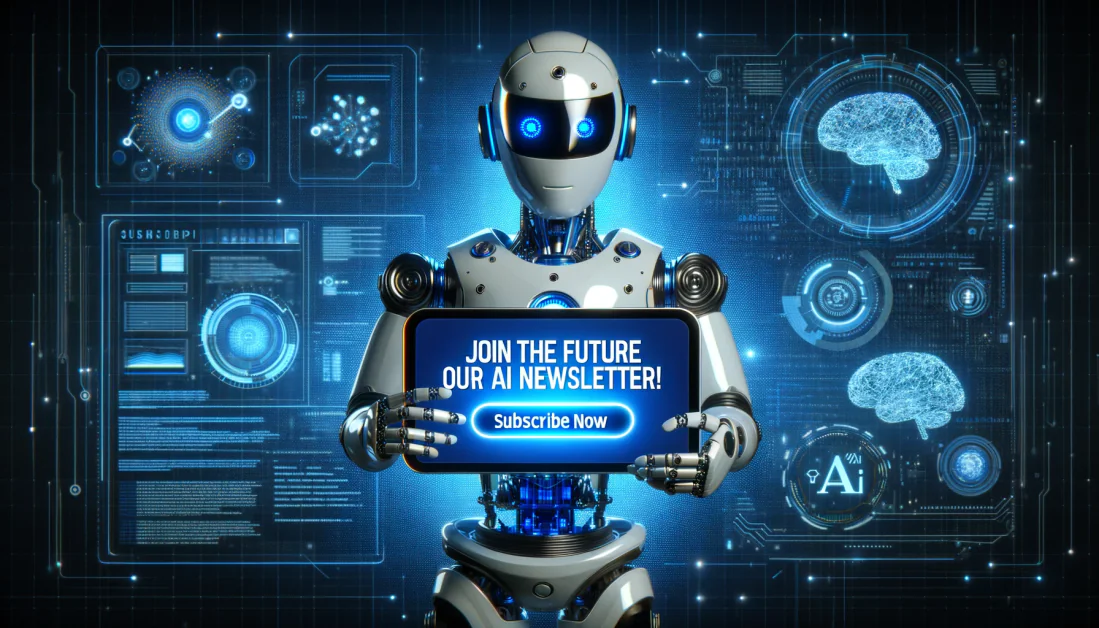
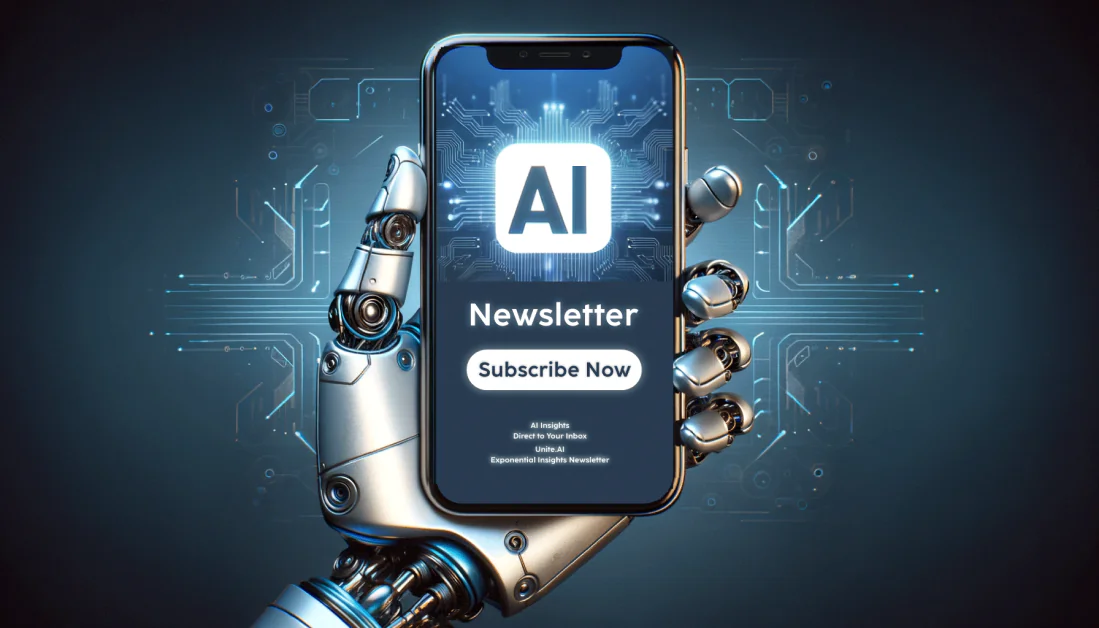