Thought Leaders
Garbage In, Garbage Out: The Crucial Role of Data Quality in AI
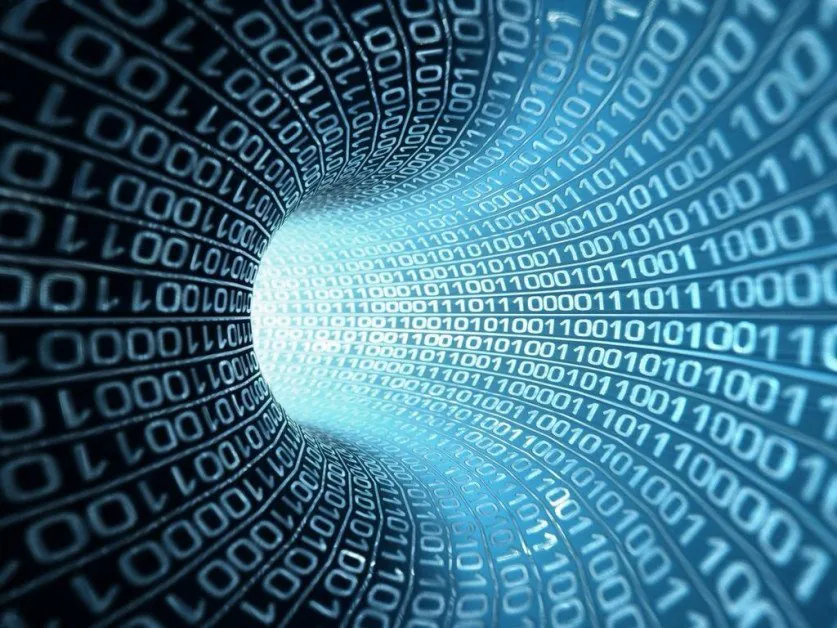
The world is buzzing with chatter about artificial intelligence (AI). From self-driving cars to personalized customer experiences, the promise of AI seems limitless. However, behind these marvels of technology lies a less glamorous – but critically important – factor: high-quality training data. Without this, even the most advanced AI systems can fall flat.
The Importance of Quality Data
Clean data serves as the foundation for any successful AI application. AI algorithms learn from data; they identify patterns, make decisions, and generate predictions based on the information they're fed. Consequently, the quality of this training data is paramount.
Poor data quality can come in various forms, from incomplete data with missing fields and inconsistent data with mismatched formats to irrelevant data that does not align with the business's objectives. When such data is fed into an AI system, the consequences can range from mild inaccuracies to severe operational disasters. Incorrect predictions could lead to flawed strategic decisions, while biased algorithms could result in reputational damage and legal issues. Therefore, prioritizing strategies for creating clean training data is crucial for organizations to harness the full potential of AI technology.
AI's Role in Improving Data Quality
While the problem of data quality may seem daunting, there is hope. The very technology affected by data quality, AI, can also play a pivotal role in enhancing it. AI-powered automated data cleaning tools can detect and rectify anomalies in the data. These tools can identify missing data, spot inconsistencies, and effortlessly remove redundant entries, providing a single, accurate view of each data point. Furthermore, they excel in data unification, seamlessly merging and reconciling data from disparate sources into a cohesive, user-friendly format. AI transforms data cleaning from a daunting task into a streamlined, automated process.
Human review of the data surfaced by AI’s advanced algorithms is crucial in creating quality training data. Human intelligence effectively guides AI in curating data for optimal output. The partnership between AI and human expertise ensures that the training data fed into AI models is of the utmost quality, resulting in more robust and accurate AI systems. By embracing AI with human feedback in their data management strategy, organizations can maintain high-quality data, substantially boosting their AI systems’ performance.
Data Products: Ensuring Data Quality from the Get-Go
The best way to avoid the pitfalls of poor data is to ensure its quality from the outset. This is where data products come in. But there's often confusion surrounding the term ‘data product,' leading to various interpretations of the definition. To bring some clarity to the discourse, a data product is a consumption-ready set of high-quality, trustworthy, and accessible data that people across an organization can use to solve business challenges. Organized by business entities and governed by domain, data products are the best version of data. They are comprehensive, clean, curated, continuously-updated data sets, aligned to key entities such as customers, vendors, or patients, that humans and machines can consume broadly and securely across an enterprise. Data products, powered by AI-driven efficiency with human oversight to provide feedback, play a crucial role in the collection and management of data, guaranteeing its quality and reliability.
At the heart of the AI revolution, data quality becomes the master key that unlocks AI's full potential. In the pursuit of data quality, AI-powered data products emerge as the solution, ensuring accuracy and reliability. Investment in data quality isn't a discretionary business decision—it's an essential commitment to the future of AI-enabled innovation. The key to avoiding the trap of ‘garbage in, garbage out' lies not in the sophistication of your AI, but in the quality of your data.
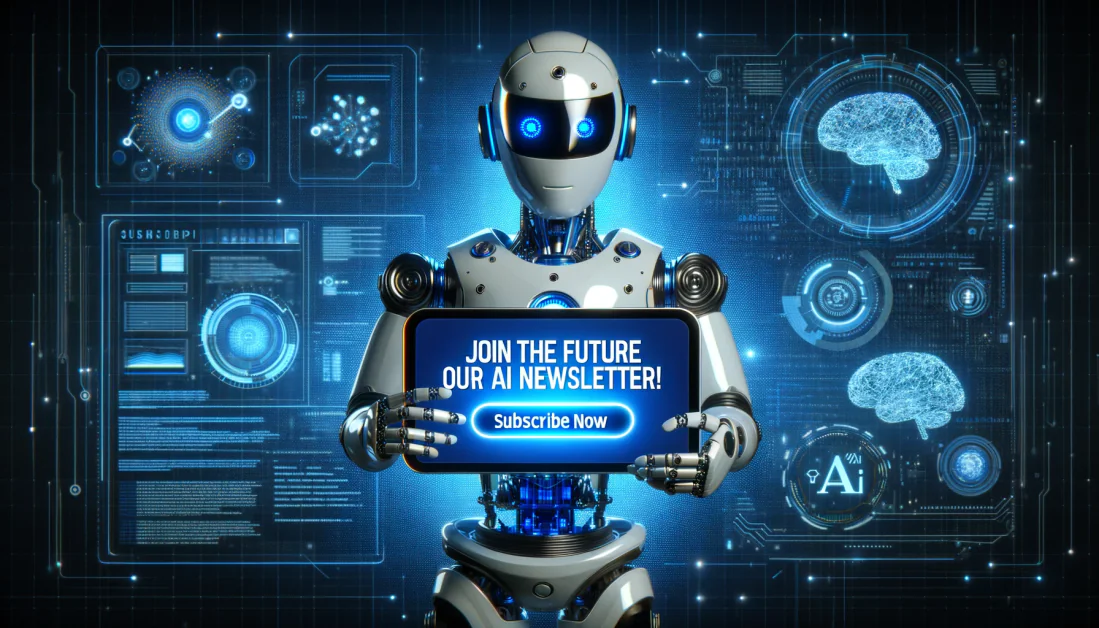
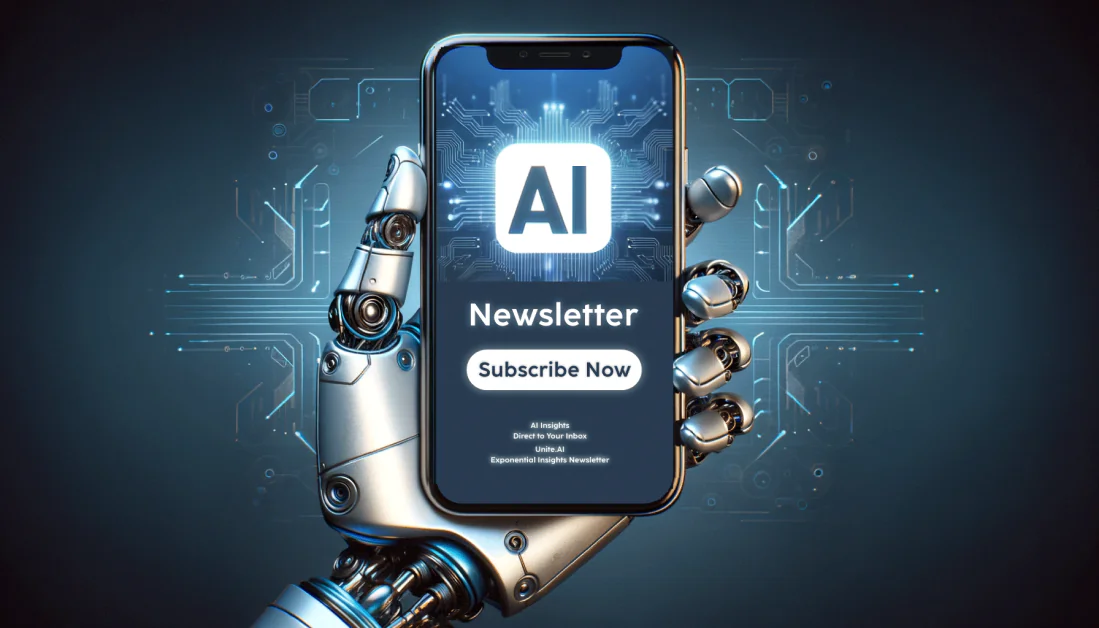