Artificial Intelligence
Everything You Need to Know About Llama 3 | Most Powerful Open-Source Model Yet | Concepts to Usage
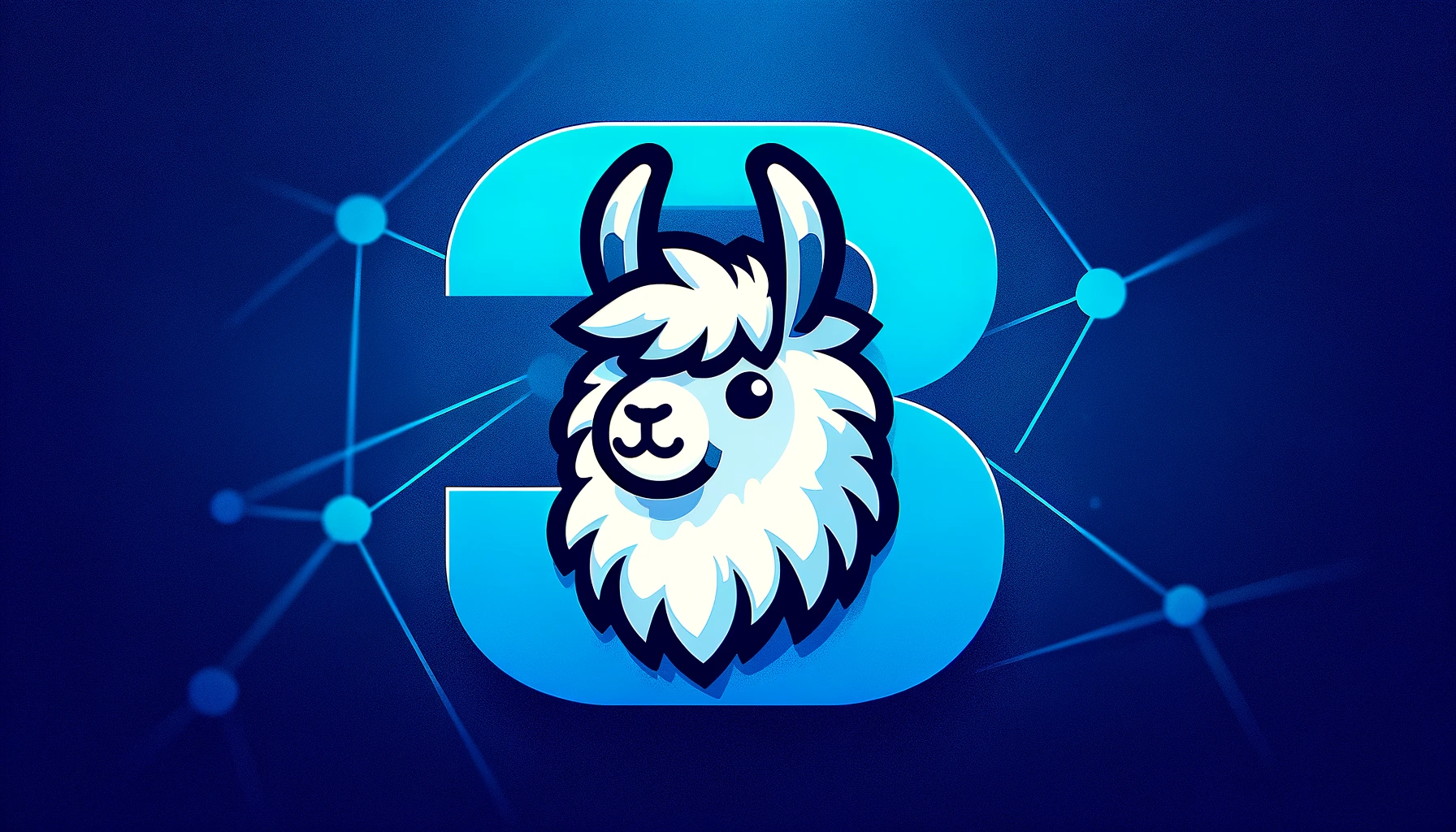
Meta has recently released Llama 3, the next generation of its state-of-the-art open source large language model (LLM). Building on the foundations set by its predecessor, Llama 3 aims to enhance the capabilities that positioned Llama 2 as a significant open-source competitor to ChatGPT, as outlined in the comprehensive review in the article Llama 2: A Deep Dive into the Open-Source Challenger to ChatGPT.
In this article we will discuss the core concepts behind Llama 3, explore its innovative architecture and training process, and provide practical guidance on how to access, use, and deploy this groundbreaking model responsibly. Whether you are a researcher, developer, or AI enthusiast, this post will equip you with the knowledge and resources needed to harness the power of Llama 3 for your projects and applications.
The Evolution of Llama: From Llama 2 to Llama 3
Meta's CEO, Mark Zuckerberg, announced the debut of Llama 3, the latest AI model developed by Meta AI. This state-of-the-art model, now open-sourced, is set to enhance Meta's various products, including Messenger and Instagram. Zuckerberg highlighted that Llama 3 positions Meta AI as the most advanced freely available AI assistant.
Before we talk about the specifics of Llama 3, let's briefly revisit its predecessor, Llama 2. Introduced in 2022, Llama 2 was a significant milestone in the open-source LLM landscape, offering a powerful and efficient model that could be run on consumer hardware.
However, while Llama 2 was a notable achievement, it had its limitations. Users reported issues with false refusals (the model refusing to answer benign prompts), limited helpfulness, and room for improvement in areas like reasoning and code generation.
Enter Llama 3: Meta's response to these challenges and the community's feedback. With Llama 3, Meta has set out to build the best open-source models on par with the top proprietary models available today, while also prioritizing responsible development and deployment practices.
Llama 3: Architecture and Training
One of the key innovations in Llama 3 is its tokenizer, which features a significantly expanded vocabulary of 128,256 tokens (up from 32,000 in Llama 2). This larger vocabulary allows for more efficient encoding of text, both for input and output, potentially leading to stronger multilingualism and overall performance improvements.
Llama 3 also incorporates Grouped-Query Attention (GQA), an efficient representation technique that enhances scalability and helps the model handle longer contexts more effectively. The 8B version of Llama 3 utilizes GQA, while both the 8B and 70B models can process sequences up to 8,192 tokens.
Training Data and Scaling
The training data used for Llama 3 is a crucial factor in its improved performance. Meta curated a massive dataset of over 15 trillion tokens from publicly available online sources, seven times larger than the dataset used for Llama 2. This dataset also includes a significant portion (over 5%) of high-quality non-English data, covering more than 30 languages, in preparation for future multilingual applications.
To ensure data quality, Meta employed advanced filtering techniques, including heuristic filters, NSFW filters, semantic deduplication, and text classifiers trained on Llama 2 to predict data quality. The team also conducted extensive experiments to determine the optimal mix of data sources for pretraining, ensuring that Llama 3 performs well across a wide range of use cases, including trivia, STEM, coding, and historical knowledge.
Scaling up pretraining was another critical aspect of Llama 3's development. Meta developed scaling laws that enabled them to predict the performance of its largest models on key tasks, such as code generation, before actually training them. This informed the decisions on data mix and compute allocation, ultimately leading to more efficient and effective training.
Llama 3's largest models were trained on two custom-built 24,000 GPU clusters, leveraging a combination of data parallelization, model parallelization, and pipeline parallelization techniques. Meta's advanced training stack automated error detection, handling, and maintenance, maximizing GPU uptime and increasing training efficiency by approximately three times compared to Llama 2.
Instruction Fine-tuning and Performance
To unlock Llama 3's full potential for chat and dialogue applications, Meta innovated its approach to instruction fine-tuning. Its method combines supervised fine-tuning (SFT), rejection sampling, proximal policy optimization (PPO), and direct preference optimization (DPO).
The quality of the prompts used in SFT and the preference rankings used in PPO and DPO played a crucial role in the performance of the aligned models. Meta's team carefully curated this data and performed multiple rounds of quality assurance on annotations provided by human annotators.
Training on preference rankings via PPO and DPO also significantly improved Llama 3's performance on reasoning and coding tasks. Meta found that even when a model struggles to answer a reasoning question directly, it may still produce the correct reasoning trace. Training on preference rankings enabled the model to learn how to select the correct answer from these traces.
The results speak for themselves: Llama 3 outperforms many available open-source chat models on common industry benchmarks, establishing new state-of-the-art performance for LLMs at the 8B and 70B parameter scales.
Responsible Development and Safety Considerations
While pursuing cutting-edge performance, Meta also prioritized responsible development and deployment practices for Llama 3. The company adopted a system-level approach, envisioning Llama 3 models as part of a broader ecosystem that puts developers in the driver's seat, allowing them to design and customize the models for their specific use cases and safety requirements.
Meta conducted extensive red-teaming exercises, performed adversarial evaluations, and implemented safety mitigation techniques to lower residual risks in its instruction-tuned models. However, the company acknowledges that residual risks will likely remain and recommends that developers assess these risks in the context of their specific use cases.
To support responsible deployment, Meta has updated its Responsible Use Guide, providing a comprehensive resource for developers to implement model and system-level safety best practices for their applications. The guide covers topics such as content moderation, risk assessment, and the use of safety tools like Llama Guard 2 and Code Shield.
Llama Guard 2, built on the MLCommons taxonomy, is designed to classify LLM inputs (prompts) and responses, detecting content that may be considered unsafe or harmful. CyberSecEval 2 expands on its predecessor by adding measures to prevent abuse of the model's code interpreter, offensive cybersecurity capabilities, and susceptibility to prompt injection attacks.
Code Shield, a new introduction with Llama 3, adds inference-time filtering of insecure code produced by LLMs, mitigating risks associated with insecure code suggestions, code interpreter abuse, and secure command execution.
Accessing and Using Llama 3
Following the launch of Meta AI's Llama 3, several open-source tools have been made available for local deployment on various operating systems, including Mac, Windows, and Linux. This section details three notable tools: Ollama, Open WebUI, and LM Studio, each offering unique features for leveraging Llama 3's capabilities on personal devices.
Ollama: Available for Mac, Linux, and Windows, Ollama simplifies the operation of Llama 3 and other large language models on personal computers, even those with less robust hardware. It includes a package manager for easy model management and supports commands across platforms for downloading and running models.
Open WebUI with Docker: This tool provides a user-friendly, Docker-based interface compatible with Mac, Linux, and Windows. It integrates seamlessly with models from the Ollama registry, allowing users to deploy and interact with models like Llama 3 within a local web interface.
LM Studio: Targeting users on Mac, Linux, and Windows, LM Studio supports a range of models and is built on the llama.cpp project. It provides a chat interface and facilitates direct interaction with various models, including the Llama 3 8B Instruct model.
These tools ensure that users can efficiently utilize Llama 3 on their personal devices, accommodating a range of technical skills and requirements. Each platform offers step-by-step processes for setup and model interaction, making advanced AI more accessible to developers and enthusiasts.
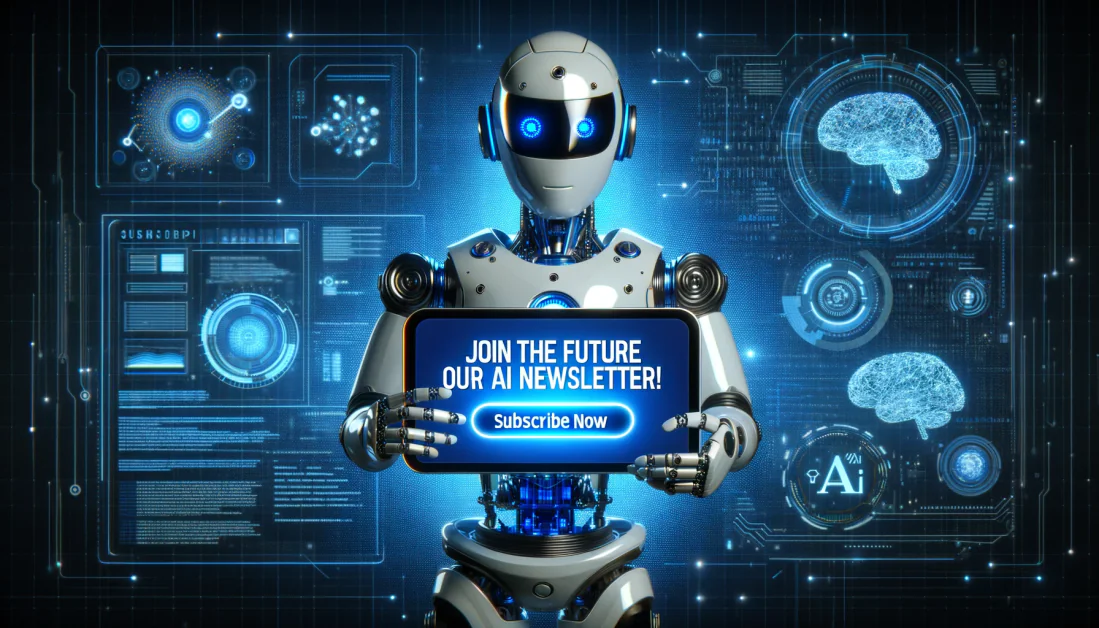
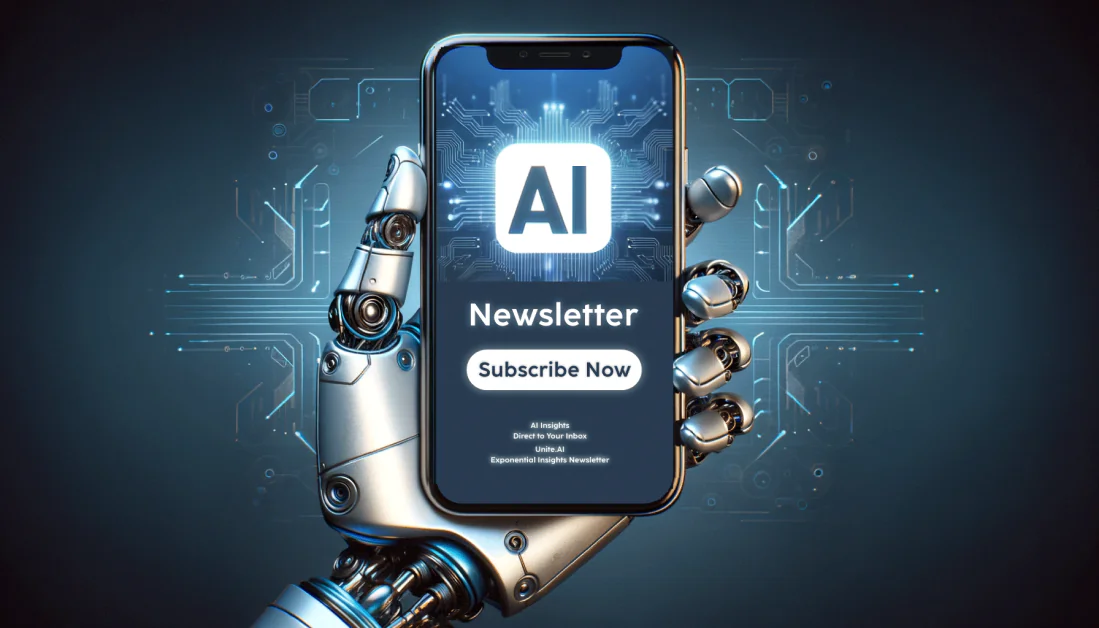