Thought Leaders
Enabling Real-World AI Deployments at Scale
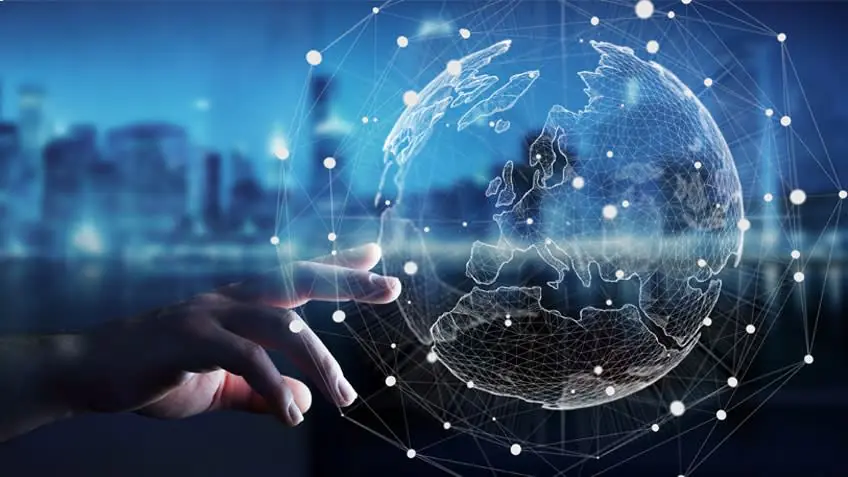
By Brad King, field CTO, Scality
The tools of AI/ML and big data have a common thread – they need data, and they need a lot of it. Conventional wisdom says the more, the better. Analysts predict global data creation will grow to more than 180 zettabytes by 2025 – and in 2020, the amount of data created and replicated hit a new high of 64.2 zettabytes.
That data is extremely valuable – often irreplaceable and sometimes representing one-time or once-in-a-lifetime events. This data needs to be stored safely and securely; and while it’s estimated that just a small percentage of this newly created data is retained, the demand for storage capacity continues to grow. In fact, the installed base of storage capacity is forecast to grow at a compound annual growth rate of 19.2% between 2020 and 2025, according to researchers at Statista.
With more data being created – particularly by these AI/ML workloads – organizations need more storage, but not all storage solutions can handle these intensive and massive workloads. What’s needed is a new approach to storage. Let’s look at how organizations are overcoming these challenges through the lens of three use cases.
The travel industry
While many of us are just getting used to traveling again after more than a year of lockdowns, the travel industry is looking to get back to pre-pandemic times in a major way. And this is making the importance of data – specifically, the relevant application and use of that data – even more important.
Imagine what you could do with the knowledge of where the majority of the world’s airline travelers are going to travel next or where they’re going tomorrow. For a travel agency, for instance, that would be huge.
But these travel organizations are dealing with so much data that sorting through it to figure out what’s meaningful is an overwhelming prospect. About a petabyte of data is generated each day, and some of the data is duplicated by sites like Kayak. This data is time-sensitive, and travel companies need to quickly discover which data is meaningful. They need a tool to be able to manage this level of scale more effectively.
The automobile industry
Another example comes from the automobile industry, which is certainly one of the most talked-about use cases. The industry has been hard at work for a long time with assistance tools like lane minders, collision avoidance and the like. All these sensors are bringing in great quantities of data. And, of course, they are developing, testing and verifying self-driving algorithms.
What the industry needs is a better way to make sense of this stored data so they can use it to analyze incidents where something went wrong, curate sensor outputs as a test case, test algorithms against sensor data and more. They need QA testing to avoid regressions, and they need to document cases that fail.
Digital pathology
Another interesting use case for AI/ML that’s also grappling with the data deluge and the need to make better use of data is digital pathology. Just like the other examples, what they really need is the ability to make better use of this data so they can do things like automatically detect pathologies in tissue samples, perform remote diagnostics and so on.
But storage today is limiting usage. Images with useful resolution are too large to store economically. However, fast object storage will enable new abilities – like image banks that can be used as a key training resource and the use of space-filling curves to name/store and retrieve multiresolution images in an object store. It also enables extensible and flexible metadata tagging, which makes it easier to search for and make sense of this information.
AI workloads require a new approach
As we’ve seen in the three cases above, it’s critical to be able to aggregate and orchestrate vast amounts of data related to AI/ML workloads. Data sets often reach multi-petabyte scale, with performance demands that could saturate the whole infrastructure. When dealing with such large-scale training and test data sets, overcoming storage bottlenecks (latency and/or throughput issues) and capacity limitations/barriers are key elements for success.
AI/ML/DL workloads require a storage architecture that can keep data flowing through the pipeline, with both excellent raw I/O performance and capacity scaling capability. The storage infrastructure must keep pace with increasingly demanding requirements across all stages of the AI/ML/DL pipeline. The solution is a storage infrastructure specifically built for speed and limitless scale.
Extracting value
Not a week goes by without stories about the potential of AI and ML to change business processes and everyday lives. There are many use cases that clearly demonstrate the benefits of using these technologies. The reality of AI in the enterprise today, though, is one of overwhelmingly large data sets and storage solutions that can’t manage these massive workloads. Innovations in automobiles, healthcare and many more industries can’t go forward until the storage issue is resolved. Fast object storage overcomes the challenge of retaining big data so organizations can extract the value from this data to move their businesses forward.
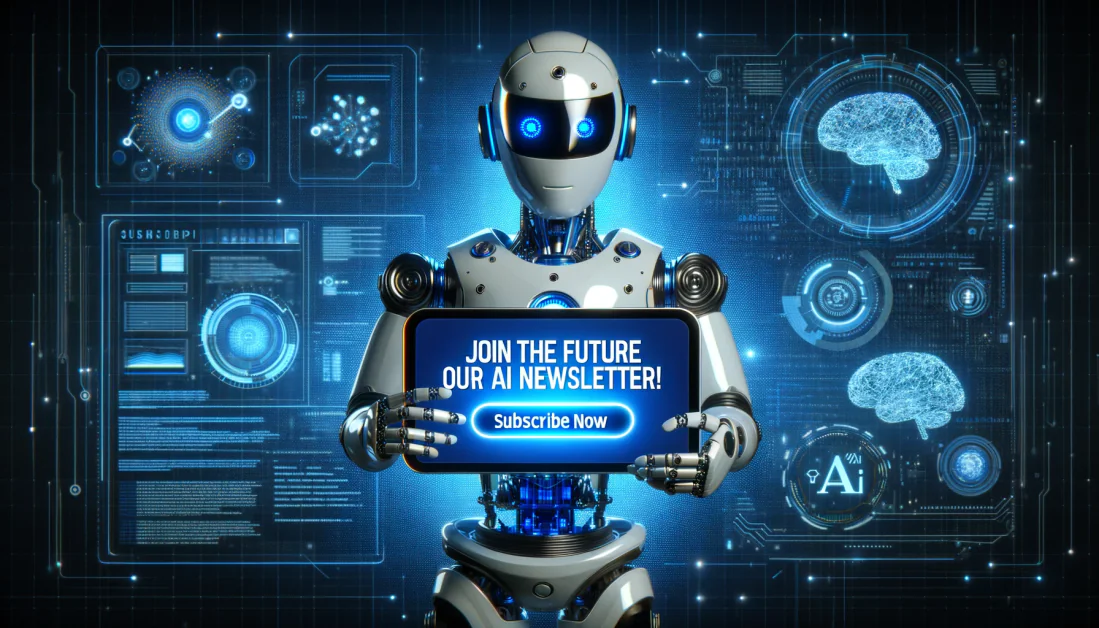
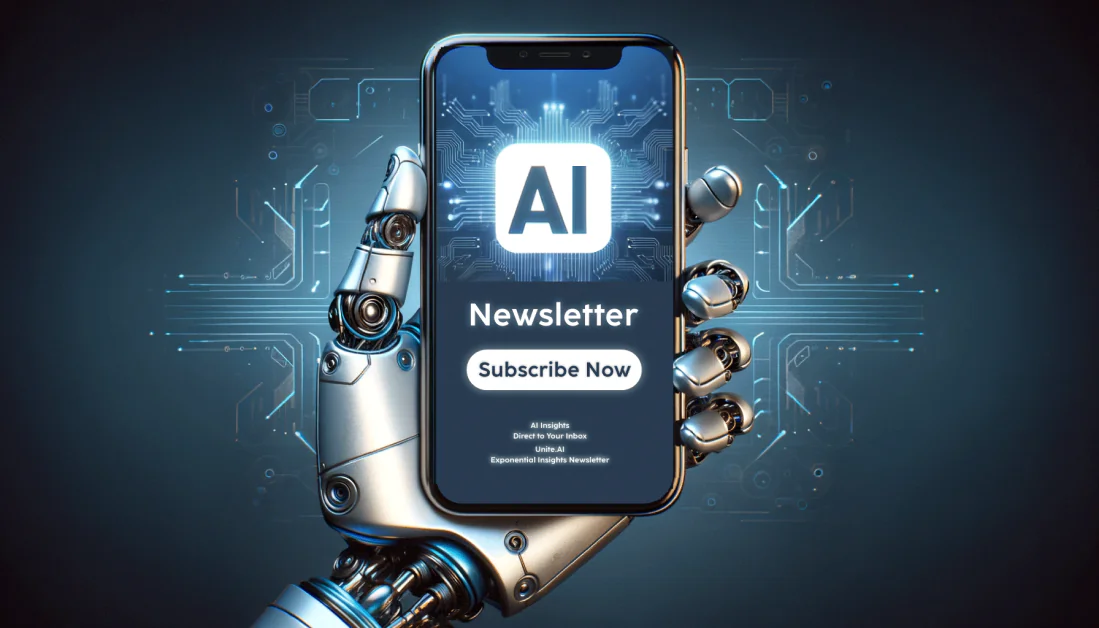