Interviews
Dr. Serafim Batzoglou, Chief Data Officer at Seer – Interview Series
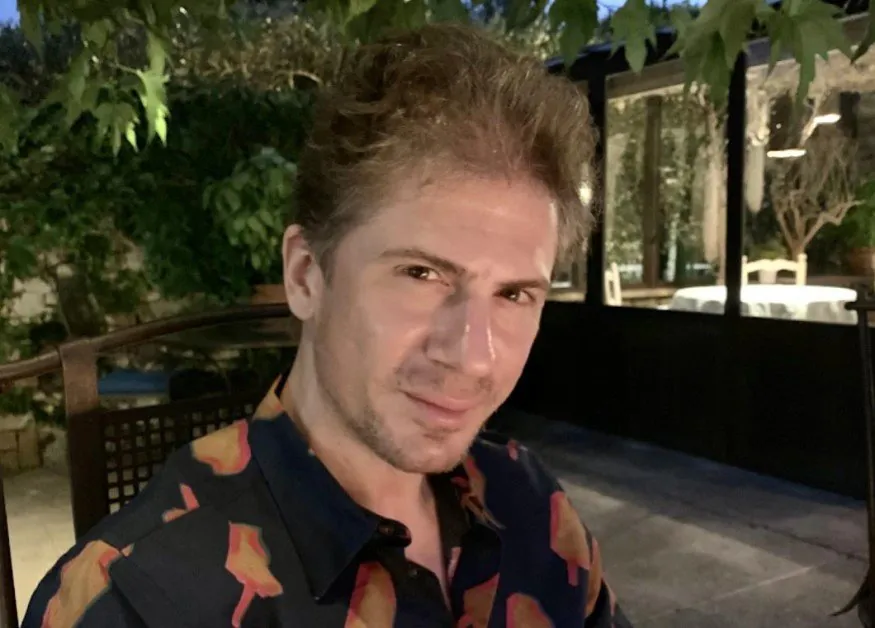
Serafim Batzoglou is Chief Data Officer at Seer. Prior to joining Seer, Serafim served as Chief Data Officer at Insitro, leading machine learning and data science in their approach to drug discovery. Prior to Insitro, he served as VP of Applied and Computational Biology at Illumina, leading research and technology development of AI and molecular assays for making genomic data more interpretable in human health.
What initially attracted you to the field of genomics?
I became interested in the field of computational biology at the start of my PhD in computer science at MIT, when I took a class on the topic taught by Bonnie Berger, who became my PhD advisor, and David Gifford. The human genome project was picking up pace during my PhD. Eric Lander, who was heading the Genome Center at MIT became my PhD co-advisor and involved me in the project. Motivated by the human genome project, I worked on whole-genome assembly and comparative genomics of human and mouse DNA.
I then moved to Stanford University as faculty at the Computer Science department where I spent 15 years, and was privileged to have advised about 30 incredibly talented PhD students and many postdoctoral researchers and undergraduates. My team’s focus has been the application of algorithms, machine learning and software tools building for the analysis of large-scale genomic and biomolecular data. I left Stanford in 2016 to lead a research and technology development team at Illumina. Since then, I have enjoyed leading R&D teams in industry. I find that teamwork, the business aspect, and a more direct impact to society are characteristic of industry compared to academia. I worked at innovative companies over my career: DNAnexus, which I co-founded in 2009, Illumina, insitro and now Seer. Computation and machine learning are essential across the technology chain in biotech, from technology development, to data acquisition, to biological data interpretation and translation to human health.
Over the last 20 years, sequencing the human genome has become vastly cheaper and faster. This led to dramatic growth in the genome sequencing market and broader adoption in the life sciences industry. We are now at the cusp of having population genomic, multi-omic and phenotypic data of sufficient size to meaningfully revolutionize healthcare including prevention, diagnosis, treatment and drug discovery. We can increasingly discover the molecular underpinnings of disease for individuals through computational analysis of genomic data, and patients have the chance to receive treatments that are personalized and targeted, especially in the areas of cancer and rare genetic disease. Beyond the obvious use in medicine, machine learning coupled with genomic information allows us to gain insights into other areas of our lives, such as our genealogy and nutrition. The next several years will see adoption of personalized, data-driven healthcare, first for select groups of people, such as rare disease patients, and increasingly for the broad public.
Prior to your current role you were Chief Data Officer at Insitro, leading machine learning and data science in their approach to drug discovery. What were some of your key takeaways from this time period with how machine learning can be used to accelerate drug discovery?
The conventional drug discovery and development “trial-and-error” paradigm is plagued with inefficiencies and extremely lengthy timelines. For one drug to get to market, it can take upwards of $1 billion and over a decade. By incorporating machine learning into these efforts, we can dramatically reduce costs and timeframes in several steps on the way. One step is target identification, where a gene or set of genes that modulate a disease phenotype or revert a disease cellular state to a more healthy state can be identified through large-scale genetic and chemical perturbations, and phenotypic readouts such as imaging and functional genomics. Another step is compound identification and optimization, where a small molecule or other modality can be designed by machine learning-driven in silico prediction as well as in vitro screening, and moreover desired properties of a drug such as solubility, permeability, specificity and non-toxicity can be optimized. The hardest as well as most important aspect is perhaps translation to humans. Here, choice of the right model—induced pluripotent stem cell-derived lines versus primary patient cell lines and tissue samples versus animal models—for the right disease poses an incredibly important set of tradeoffs that ultimately reflect on the ability of the resulting data plus machine learning to translate to patients.
Seer Bio is pioneering new ways to decode the secrets of the proteome to improve human health, for readers who are unfamiliar with this term what is the proteome?
The proteome is the changing set of proteins produced or modified by an organism over time and in response to environment, nutrition and health state. Proteomics is the study of the proteome within a given cell type or tissue sample. The genome of a human or other organisms is static: with the important exception of somatic mutations, the genome at birth is the genome one has their entire life, copied exactly in each cell of their body. The proteome is dynamic and changes in the time spans of years, days and even minutes. As such, proteomes are vastly closer to phenotype and ultimately to health status than are genomes, and consequently more informative for monitoring health and understanding disease.
At Seer, we have developed a new way to access the proteome that provides deeper insights into proteins and proteoforms in complex samples such as plasma, which is a highly accessible sample that unfortunately to-date has posed a great challenge for conventional mass spectrometry proteomics.
What is the Seer’s Proteograph™ platform and how does it offer a new view of the proteome?
Seer’s Proteograph platform leverages a library of proprietary engineered nanoparticles, powered by a simple, rapid, and automated workflow, enabling deep and scalable interrogation of the proteome.
The Proteograph platform shines in interrogating plasma and other complex samples that exhibit large dynamic range—many orders of magnitude difference in the abundance of various proteins in the sample—where conventional mass spectrometry methods are unable to detect the low abundance part of the proteome. Seer’s nanoparticles are engineered with tunable physiochemical properties that gather proteins across the dynamic range in an unbiased manner. In typical plasma samples, our technology enables detection of 5x to 8x more proteins than when processing neat plasma without using the Proteograph. As a result, from sample prep to instrumentation to data analysis, our Proteograph Product Suite helps scientists find proteome disease signatures that might otherwise be undetectable. We like to say that at Seer, we’re opening up a new gateway to the proteome.
Furthermore, we’re allowing scientists to easily perform large-scale proteogenomic studies. Proteogenomics is the combining of genomic data with proteomic data to identify and quantify protein variants, link genomic variants with protein abundance levels, and ultimately link the genome and the proteome to phenotype and disease, and start disentangling the causal and downstream genetic pathways associated with disease.
Can you discuss some of the machine learning technology that is currently used at Seer Bio?
Seer is leveraging machine learning at all steps from technology development to downstream data analysis. Those steps include: (1) design of our proprietary nanoparticles, where machine learning helps us determine which physicochemical properties and combinations of nanoparticles will work with specific product lines and assays; (2) detection and quantification of peptides, proteins, variants and proteoforms from the readout data produced from the MS instruments; (3) downstream proteomic and proteogenomic analyses in large-scale population cohorts.
Last year, we published a paper in Advanced Materials combining proteomics methods, nanoengineering and machine learning for improving our understanding of the mechanisms of protein corona formation. This paper uncovered nano-bio interactions and is informing Seer in the creation of improved future nanoparticles and products.
Beyond nanoparticle development, we have been developing novel algorithms to identify variant peptides and post-translational modifications (PTMs). We recently developed a method for detection of protein quantified trait loci (pQTLs) that is robust to protein variants, which is a known confounder for affinity-based proteomics. We are extending this work to directly identify these peptides from the raw spectra using deep learning-based de novo sequencing methods to allow search without inflating the size of spectral libraries.
Our team is also developing methods to enable scientists without deep expertise in machine learning to optimally tune and utilize machine learning models in their discovery work. This is accomplished via a Seer ML framework based on the AutoML tool, which allows efficient hyperparameter tuning via Bayesian optimization.
Finally, we are developing methods to reduce the batch effect and increase the quantitative accuracy of the mass spec readout by modeling the measured quantitative values to maximize expected metrics such as correlation of intensity values across peptides within a protein group.
Hallucinations are a common issue with LLMs, what are some of the solutions to prevent or mitigate this?
LLMs are generative methods that are given a large corpus and are trained to generate similar text. They capture the underlying statistical properties of the text they are trained on, from simple local properties such as how often certain combinations of words (or tokens) are found together, to higher level properties that emulate understanding of context and meaning.
However, LLMs are not primarily trained to be correct. Reinforcement learning with human feedback (RLHF) and other techniques help train them for desirable properties including correctness, but are not fully successful. Given a prompt, LLMs will generate text that most closely resembles the statistical properties of the training data. Often, this text is also correct. For example, if asked “when was Alexander the Great born,” the correct answer is 356 BC (or BCE), and an LLM is likely to give that answer because within the training data Alexander the Great’s birth appears often as this value. However, when asked “when was Empress Reginella born,” a fictional character not present in the training corpus, the LLM is likely to hallucinate and create a story of her birth. Similarly, when asked a question that the LLM may not retrieve a right answer for (either because the right answer does not exist, or for other statistical purposes), it is likely to hallucinate and answer as if it knows. This creates hallucinations that are an obvious problem for serious applications, such as “how can such and such cancer be treated.”
There are no perfect solutions yet for hallucinations. They are endemic to the design of the LLM. One partial solution is proper prompting, such as asking the LLM to “think carefully, step-by-step,” and so on. This increases the LLMs likelihood to not concoct stories. A more sophisticated approach that is being developed is the use of knowledge graphs. Knowledge graphs provide structured data: entities in a knowledge graph are connected to other entities in a predefined, logical manner. Constructing a knowledge graph for a given domain is of course a challenging task but doable with a combination of automated and statistical methods and curation. With a built-in knowledge graph, LLMs can cross-check the statements they generate against the structured set of known facts, and can be constrained to not generate a statement that contradicts or is not supported by the knowledge graph.
Because of the fundamental issue of hallucinations, and arguably because of their lack of sufficient reasoning and judgment abilities, LLMs are today powerful for retrieving, connecting and distilling information, but cannot replace human experts in serious applications such as medical diagnosis or legal advice. Still, they can tremendously enhance the efficiency and capability of human experts in these domains.
Can you share your vision for a future where biology is steered by data rather than hypotheses?
The traditional hypothesis-driven approach, which involves researchers finding patterns, developing hypotheses, performing experiments or studies to test them, and then refining theories based on the data, is becoming supplanted by a new paradigm based on data-driven modeling.
In this emerging paradigm, researchers start with hypothesis-free, large-scale data generation. Then, they train a machine learning model such as an LLM with the objective of accurate reconstruction of occluded data, strong regression or classification performance in a number of downstream tasks. Once the machine learning model can accurately predict the data, and achieves fidelity comparable to the similarity between experimental replicates, researchers can interrogate the model to extract insight about the biological system and discern the underlying biological principles.
LLMs are proving to be especially good in modeling biomolecular data, and are geared to fuel a shift from hypothesis-driven to data-driven biological discovery. This shift will become increasingly pronounced over the next 10 years and allow accurate modeling of biomolecular systems at a granularity that goes well beyond human capacity.
What is the potential impact for disease diagnosis and drug discovery?
I believe LLM and generative AI will lead to significant changes in the life sciences industry. One area that will benefit greatly from LLMs is clinical diagnosis, specifically for rare, difficult-to-diagnose diseases and cancer subtypes. There are tremendous amounts of comprehensive patient information that we can tap into – from genomic profiles, treatment responses, medical records and family history – to drive accurate and timely diagnosis. If we can find a way to compile all this data such that they are easily accessible, and not siloed by individual health organizations, we can dramatically improve diagnostic precision. This is not to imply that the machine learning models, including LLMs, will be able to autonomously operate in diagnosis. Due to their technical limitations, in the foreseeable future they will not be autonomous, but instead they will augment human experts. They will be powerful tools to help the doctor provide superbly informed assessments and diagnoses in a fraction of the time needed to date, and to properly document and communicate their diagnoses to the patient as well as to the entire network of health providers connected through the machine learning system.
The industry is already leveraging machine learning for drug discovery and development, touting its ability to reduce costs and timelines compared to the traditional paradigm. LLMs further add to the available toolbox, and are providing excellent frameworks for modeling large-scale biomolecular data including genomes, proteomes, functional genomic and epigenomic data, single-cell data, and more. In the foreseeable future, foundation LLMs will undoubtedly connect across all these data modalities and across large cohorts of individuals whose genomic, proteomic and health information is collected. Such LLMs will aid in generation of promising drug targets, identify likely pockets of activity of proteins associated with biological function and disease, or suggest pathways and more complex cellular functions that can be modulated in a specific way with small molecules or other drug modalities. We can also tap into LLMs to identify drug responders and non-responders based on genetic susceptibility, or to repurpose drugs in other disease indications. Many of the existing innovative AI-based drug discovery companies are undoubtedly already starting to think and develop in this direction, and we should expect to see the formation of additional companies as well as public efforts aimed at the deployment of LLMs in human health and drug discovery.
Thank you for the detailed interview, readers who wish to learn more should visit Seer.
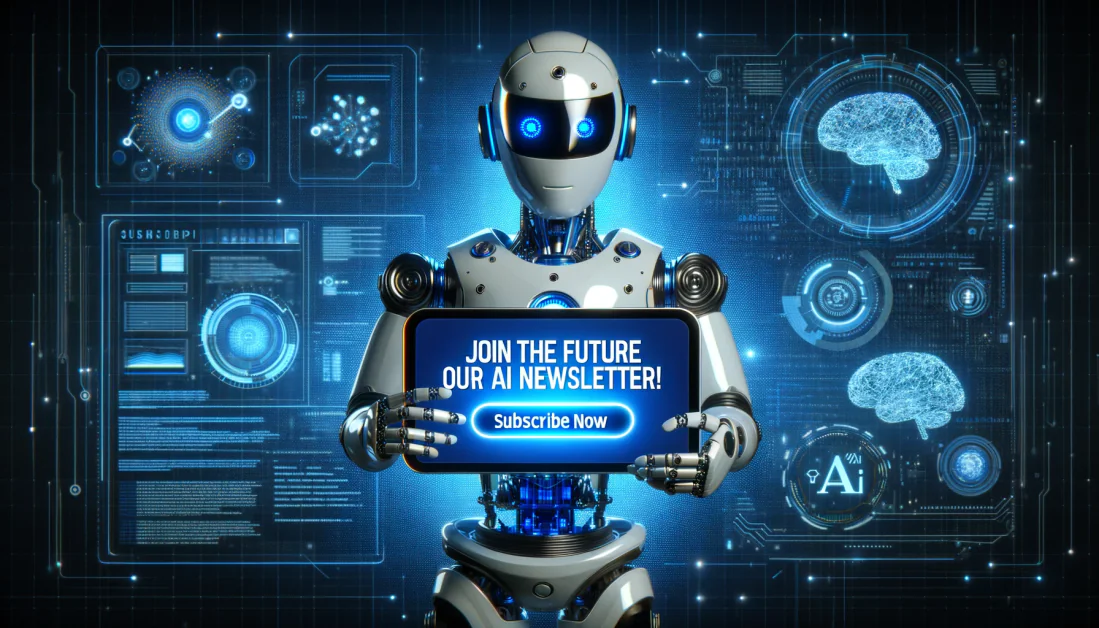
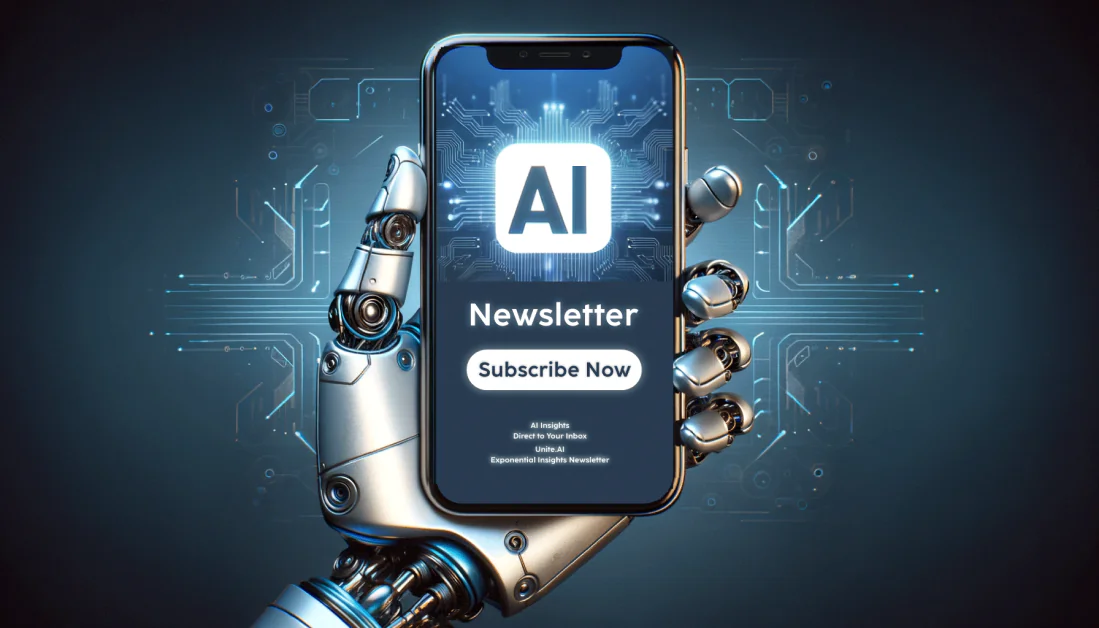