Artificial Intelligence
Determining Gender Through Walking Styles With Machine Learning
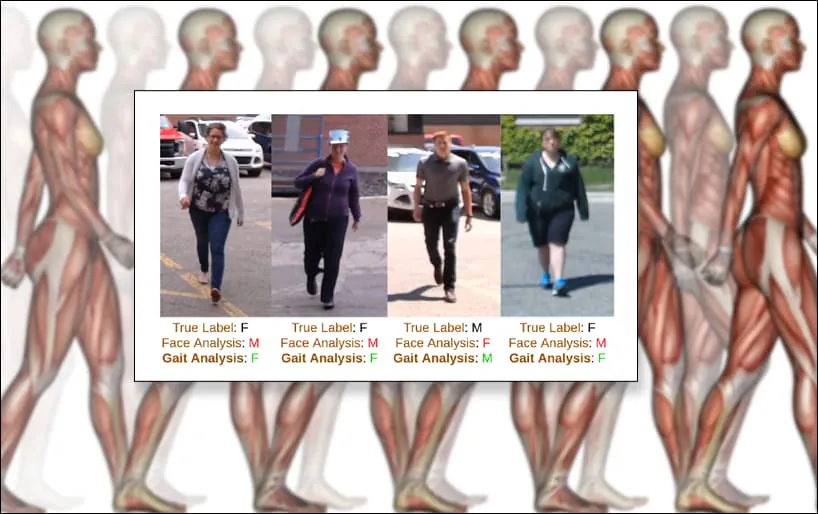
Researchers from Romania have developed a machine learning system capable of identifying the gender of a person from the way they walk, without the need to analyze facial components (which may be hidden or obscured), and without relying on silhouette analysis or other body-worn clues to gender (which can be ‘spoofed' by members of another gender).
Rather, the new system uses existing labeling systems based on these ephemeral (and changeable) signals to identify the core characteristics that distinguish the gait of men and women, resulting in a system that effectively identifies gender only from the ‘skeletal' movements of a person walking.
Effectively, this new approach quantifies the different ways that men and women walk without recourse to other signals; but since it uses other characteristics (such as face information) to initially label gait styles, the research leaves open the question of which particular traits distinguish the sexes as they walk.
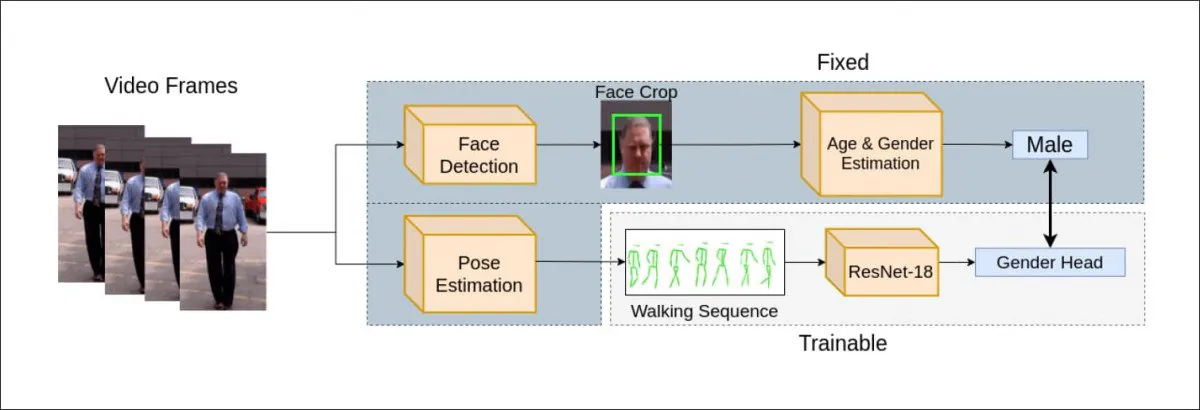
The new method derives gender identity from facial analysis models which operate under constraints (such as limited usable angle, and the need for dataset curation). The system then assigns skeletal movement characteristics as male or female, and distills characteristic walking signatures for each, ignoring facial, clothing and other unreliable sources of data. Source: https://arxiv.org/pdf/2111.00538.pdf
The new paper is titled From Face to Gait: Weakly-Supervised Learning of Gender Information from Walking Patterns, and comes from researchers at the University Politehnica at Bucharest.
The system performs on a par with facial analysis models, and frequently exceeds those standards, with an F1 score of up to 91%, and offers a high level of generalization to novel scenarios, including a variety of viewpoints and circumstances that will typically block the effectiveness of face-based or similar gender recognition systems. These include face-obscured viewpoints, non-frontal angles and the very typical scenario of low resolution imagery, or the surveilling of people who are distant within the image, where only movement-style remains as a potential reliable indicator of gender.
Gender Gap
As the researchers conclude, such a system has great potential for demographic-gathering frameworks that are currently hindered not only by mask adoption under COVID, but also by the eccentricities of fashion and happenstance that make clothing and silhouette analysis an unreliable method of identifying gender from surveillance footage.
In terms of surveillance, being able to discount all potential targets that do not fit the gender of a target subject could cut pre-processing and the need for human and machine attention by as much as half – because current identification systems often struggle to correctly assign gender to a surveilled individual.
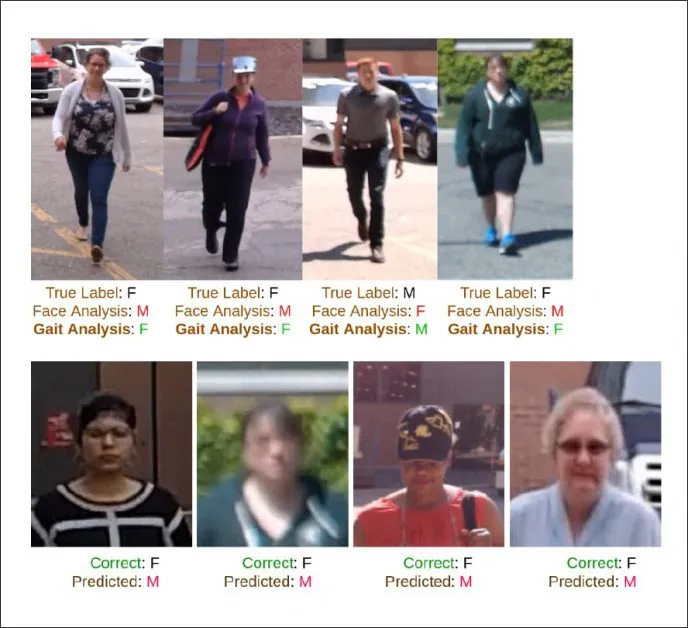
From the new paper: various examples where gender recognition systems fail. In the above row, we see the researchers' new gait-analysis system correctly matching the true label for the image (M or F), whereas face analysis has failed in the same instance. In the row below we see instances where the labeling tools used by the researchers have produced ‘noisy' (i.e. incorrect) gender labels. To counter this, the researchers used PENCIL (‘Probabilistic End-to-end Noise Correction for Learning with Noisy Labels'), among other methods.
Naturally, the possibility for reliable gender recognition through gait analysis is likely to increase current interest in gait-spoofing approaches.
Gender Determination by Proxy
It is theoretically possible to have arrived at the same functionality achieved by the new project through rigorous analysis of hand-curated skeletal movement data. Had this been done, it is likely that the new project might have deeper insights into which movement characteristics best define gender. However, such an approach signifies a large commitment of resources, and the researchers have instead used existing (less resilient) systems to generate the labels necessary.
These ‘pseudo-labels' offer no outright insight into gender-based walking traits, but make it possible to filter walking patterns by gender in a highly generalizable way that can be achieved within resource constraints.
Initially the researchers used the 2019 Front View Gait (FVG) dataset, which addresses the challenge of gait recognition from a frontal angle, which offers fewer clues than lateral views. The dataset contains walking samples with many obstacles, such as varied walking speed, cluttered background, varying resolution and differences in clothing.
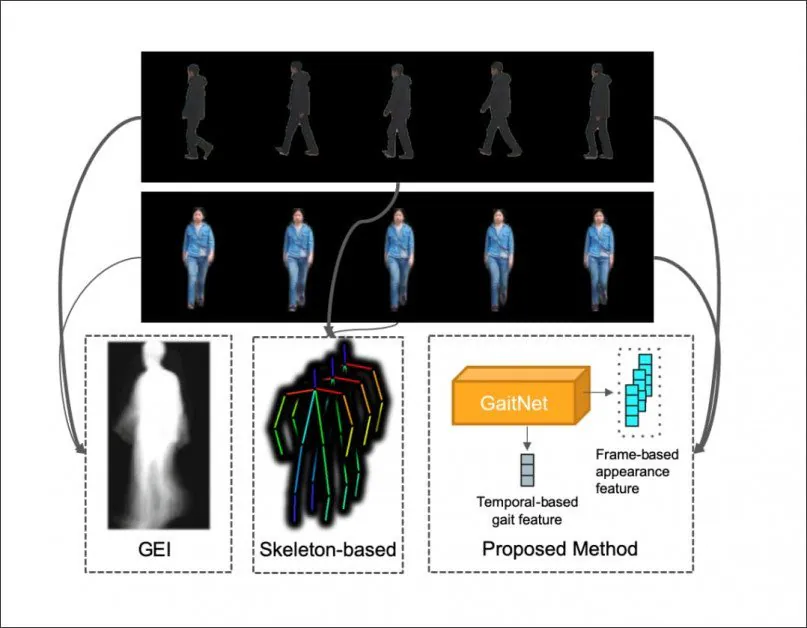
From the 2019 FVG paper, GaitNet automatically learns the essentialized gait features from ‘walking video', based on front view footage, a frequent viewing scenario in public-facing cameras. Source: https://arxiv.org/pdf/1904.04925.pdf
Since FVG is not oriented towards gender identification, the authors manually annotated 226 subjects in the dataset with gender information in order to develop ground truth for the framework.
Facial detection was facilitated through MTCNN, and demographic attributes determined by the IMDB-WIKI dataset. Since gait analysis is potentially far more effective at long distance than face-based inference, the final labels were obtained by a weighted average of gender confidence derived from the area of the facial bounding box relative to the dimensions of the frame. Skeletons were extracted with AlphaPose, which removes any potential ‘giveaways', such as the objective height of the subject (which can't be evaluated for certain in ad hoc public camera scenarios).
Testing
The system was tested against the CASIA-B gait database, undersampling the over-represented males in the dataset to ensure parity of testing, with the data split for 80% training and 20% validation.
The researchers used their own prior work, a WildGait network (see image below), to calculate similarity between walking sequences. The gender IDs, already established are now effectively ushered through to this stage of the framework process.
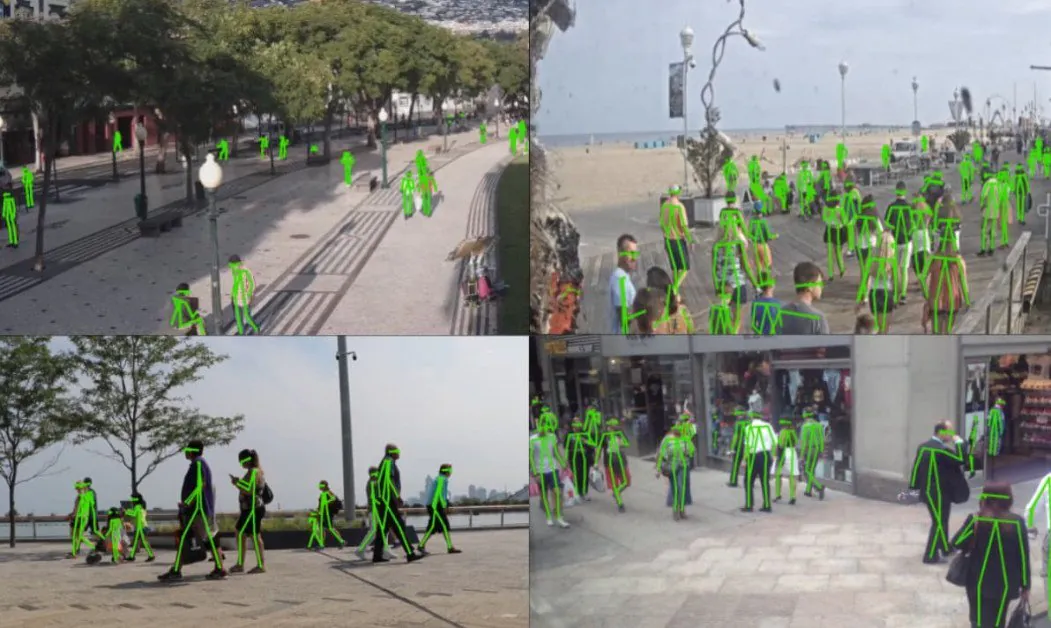
WildGait is a Spatio-Temporal Graph Convolutional Network trained on high volume, automatically annotated skeleton sequences derived from real-world, surveillance streams. Source: https://arxiv.org/pdf/2105.05528.pdf
In concluding, the authors state that the system matches state-of-the-art face-based systems in terms of accuracy in determining gender. Since there are so many possible angles that could occur in source walking video, the results are distributed across a range of those possible viewpoints:
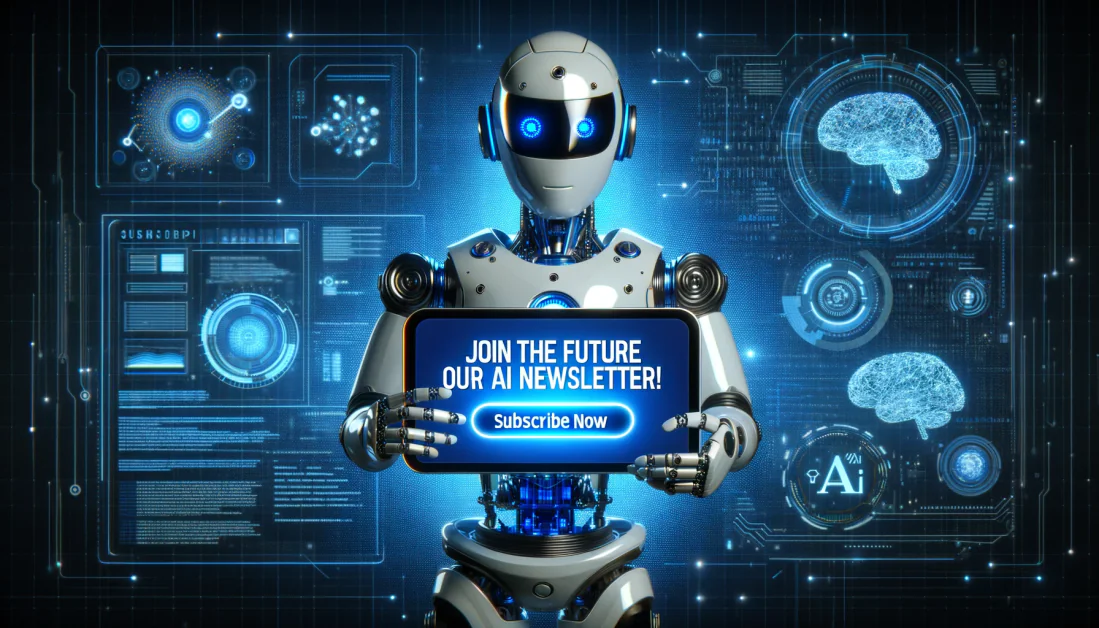
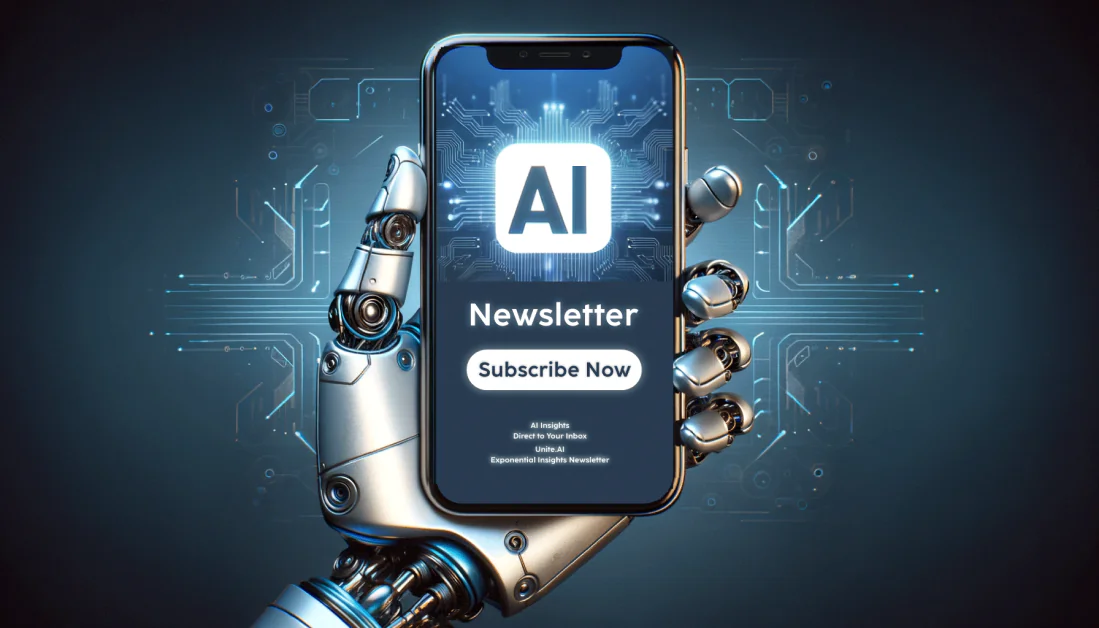