Cybersecurity
DeepTeeth: A Biometric ID System That Uses Teeth
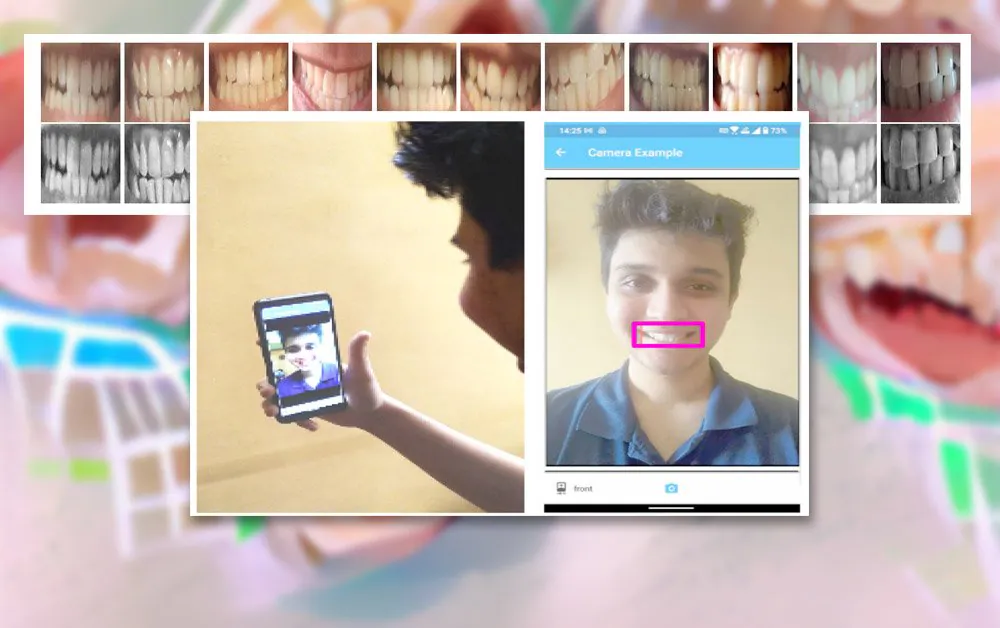
Researchers from India have proposed a biometric system for using teeth as an authentication token for secure systems on mobile devices. Called DeepTeeth, the system overcomes stumbling blocks that previous efforts towards this goal have encountered, such as excessive training time or high or unrealistic data training requirements, to achieve a reported accuracy rate of 100%.
It is also specifically aimed at frugal mobile environments and casual user authentications scenarios, rather than the more common use of such techniques in an expensive forensic analysis context.
The new pre-print, from researchers at the Birla Institute of Technology and Science Pilani at Rajasthan, utilizes a data image size of only 75×75 pixels, is an end-to-end few-shot framework, and has minimal local resource needs compared to prior attempts for tooth-based machine learning authentication systems.
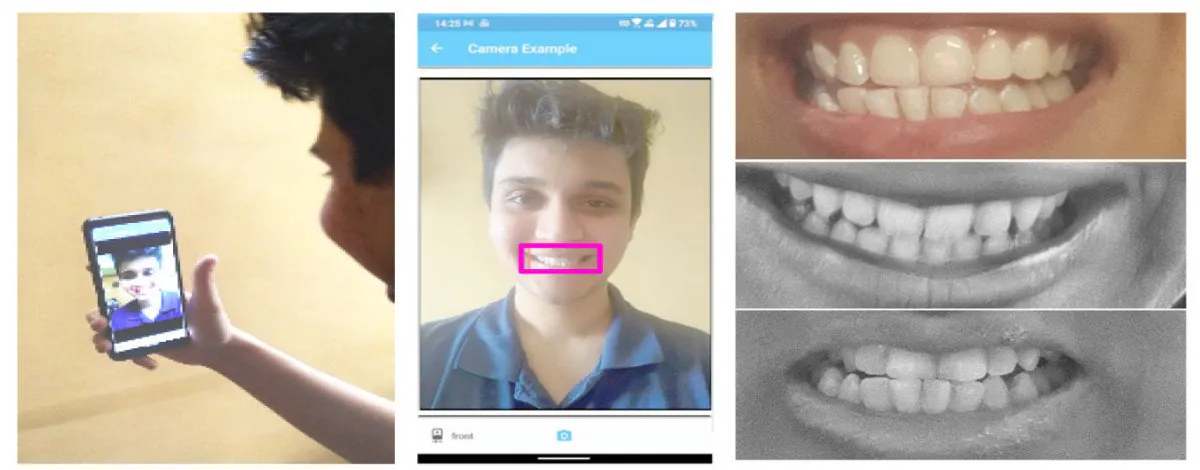
The proposed usage for DeepTeeth-based authentication. Source: https://arxiv.org/pdf/2107.13217.pdf
Teeth as ID Signifiers
Though teeth might be considered the most volatile of facial features, due to the frequency of surgical intervention compared to other types of cosmetic or facial reconstruction surgery, the paper notes that in the long term and on average, they remain the most consistent of our facial identification characteristics
Perhaps most famously, the resilience of our dental configurations is exemplified in how frequently they are used for post-mortem identification, where all other tissue has fallen victim to fire or other extreme forms of trauma. Additionally, teeth are the very last component of the body to degrade after death.
Whereas datasets for this type of forensic dentistry are specialized, and require custom scanning equipment (usually with an x-ray component), DeepTeeth requires only a series of facile ‘dental selfies' to establish a baseline ID.
Furthermore, the paper's researchers found that their teeth-based ID framework is resistant to the kind of spoof attacks that have been effectively leveled at fingerprint and facial ID authentication methods.

Normalized Region of Interest (RoI) images, and their corresponding enhancements in the automated DeepTeeth work-flow.
Capture, Processing and Training
The DeepTeeth system operates in an Android app, with the subject providing multiple captures. Photos of the teeth can be taken from various angles and in a variety of lighting conditions, and are processed locally for later inference at authentication time.
To generate the core training database, the researchers collected teeth images from 51 volunteers. The volunteers used a beta of the Android app to obtain the images themselves. The app identifies and localizes the teeth area that it's seeking to acquire. Each user submitted four example teeth images within a space of 3-4 days.
The data was tested in a Siamese network where itwas also run against a competing, older method – Google's 2015 FaceNet. The training used a batch size of 16 on an Adam optimizer. The model was trained on a Dell Inspiron-15-5577 using a Nvidia GTX 1050 GPU, with the training taking slightly less than 25 minutes to generate a 256-dimensional feature vector.
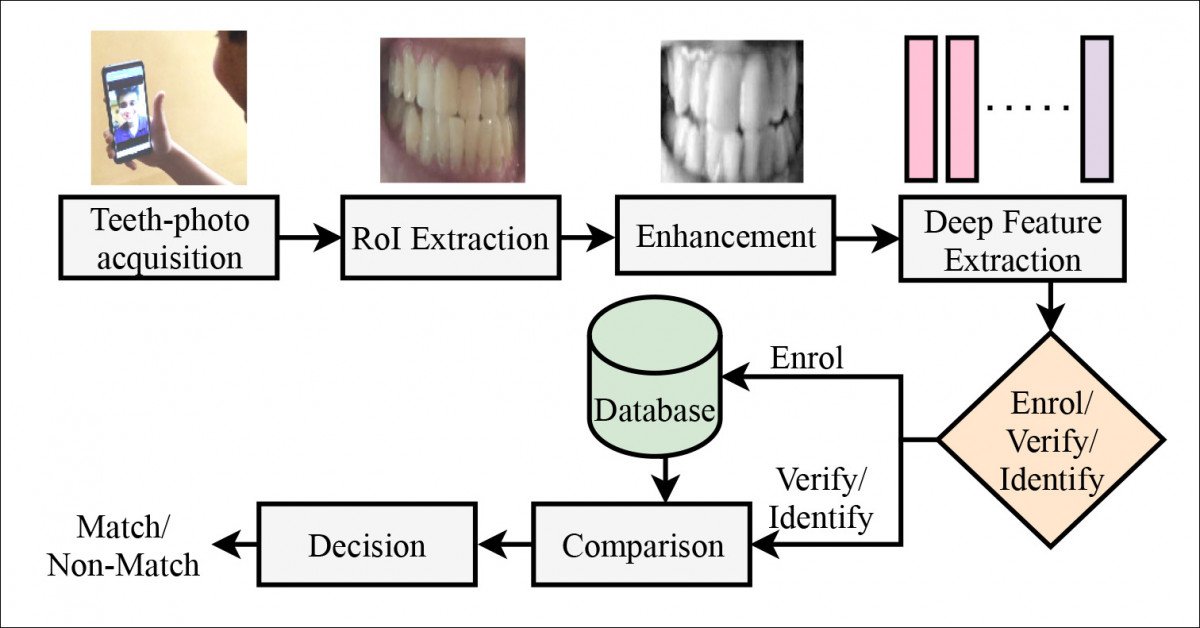
The DeepTeeth approach passes cropped raw user-taken images through an enhancement framework for subsequent feature extraction before on-device processing through a generic pre-trained local network.
Though the initially captured and cropped teeth section measure 1416 x 510 pixels, an unwieldy size even for server-based machine learning training, the smaller grey scale images derived from these captures are what run through the system, with the larger data discarded.
The loss function used for training the classification network is SoftMax, which is lightweight and resilient enough for the target operating environment.
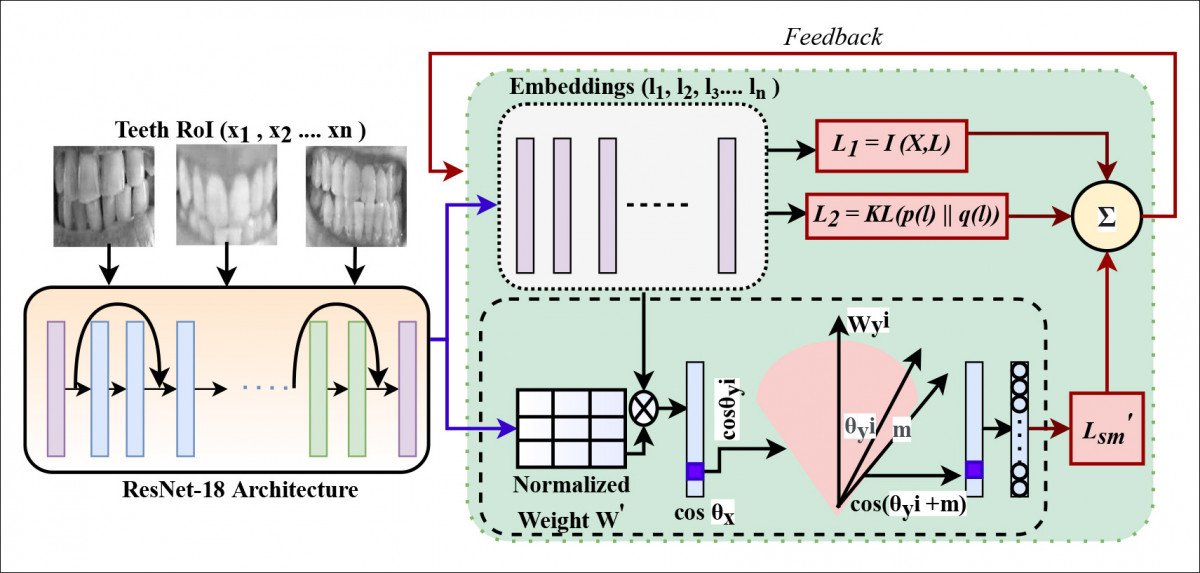
The loss function architecture of DeepTeeth.
Results
The researchers used five separate performance parameters to evaluate DeepTeeth, and found that the system operates optimally with a meager input size of 75 pixels squared, achieving a 100% success rate.
Previous attempts to use teeth as a biometric indicator include the 2008 study Multimodal biometric authentication using teeth image and voice in mobile environment, which essentially added teeth as a back-up method for voice-based identification.
Another contender, from 2020, was the SmileAuth framework proposed by researchers at Hunan University in China, a collaboration with Michigan State University and the University of Massachusetts. Experimental results at the time of the paper's publication suggested that the SmileAuth system could achieve an accuracy rate up to 99.74%. The system used Random Forest for feature extraction.
The researchers contend that DeepTeeth improves on all previous attempts in this niche area of biometrics, and takes dental recognition beyond the forensic sphere as a viable potential avenue for face-based ID authentication.
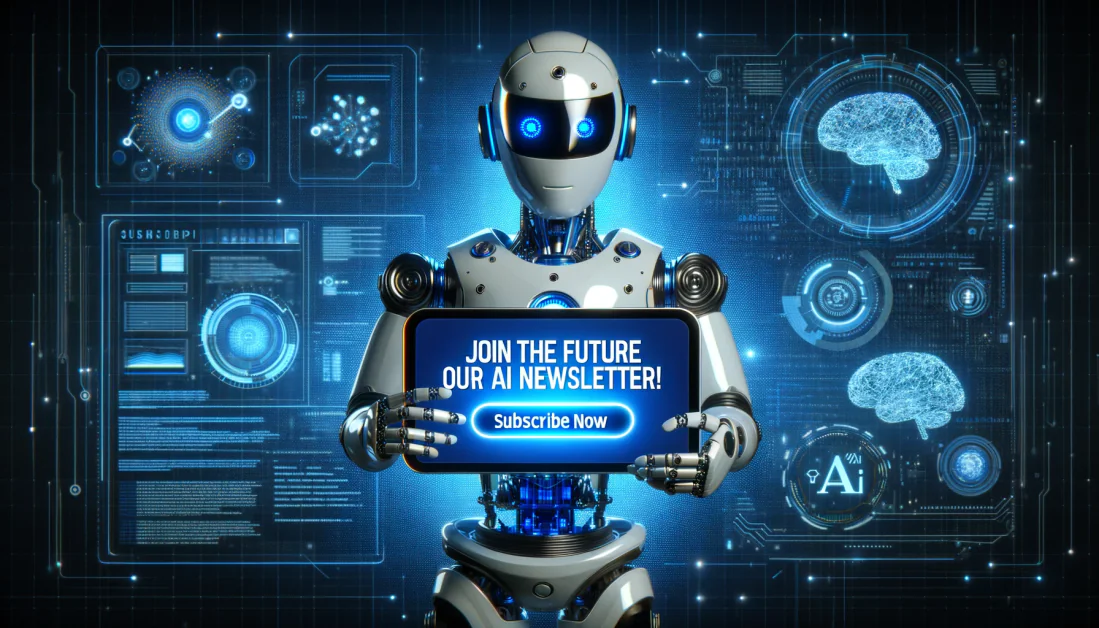
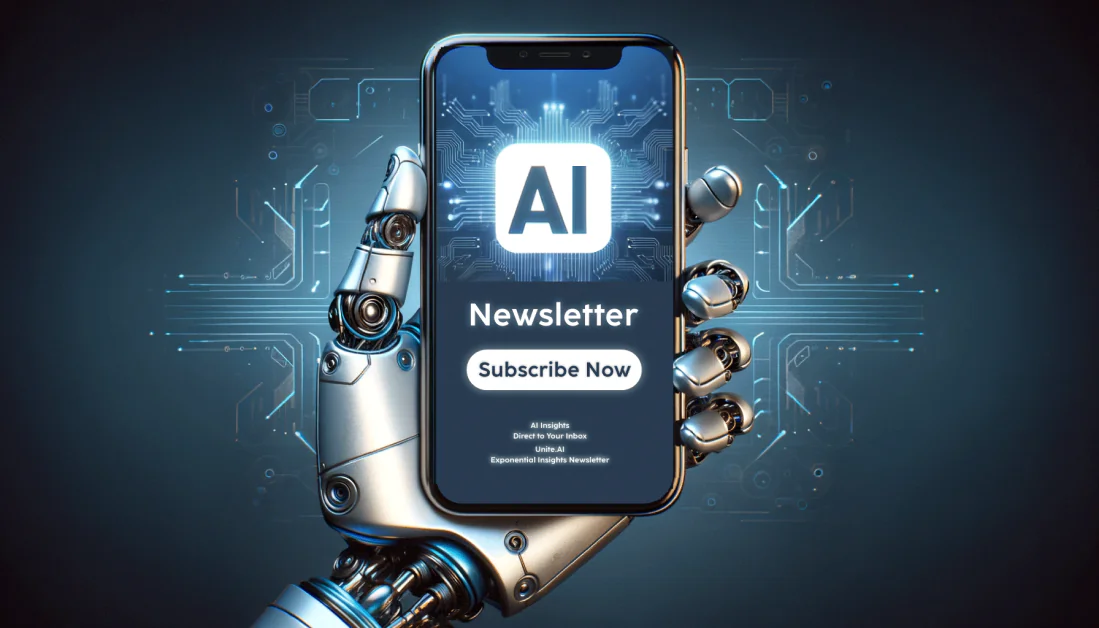