Healthcare
Deep Neural Network Can Screen for Skin Disease on Laptop
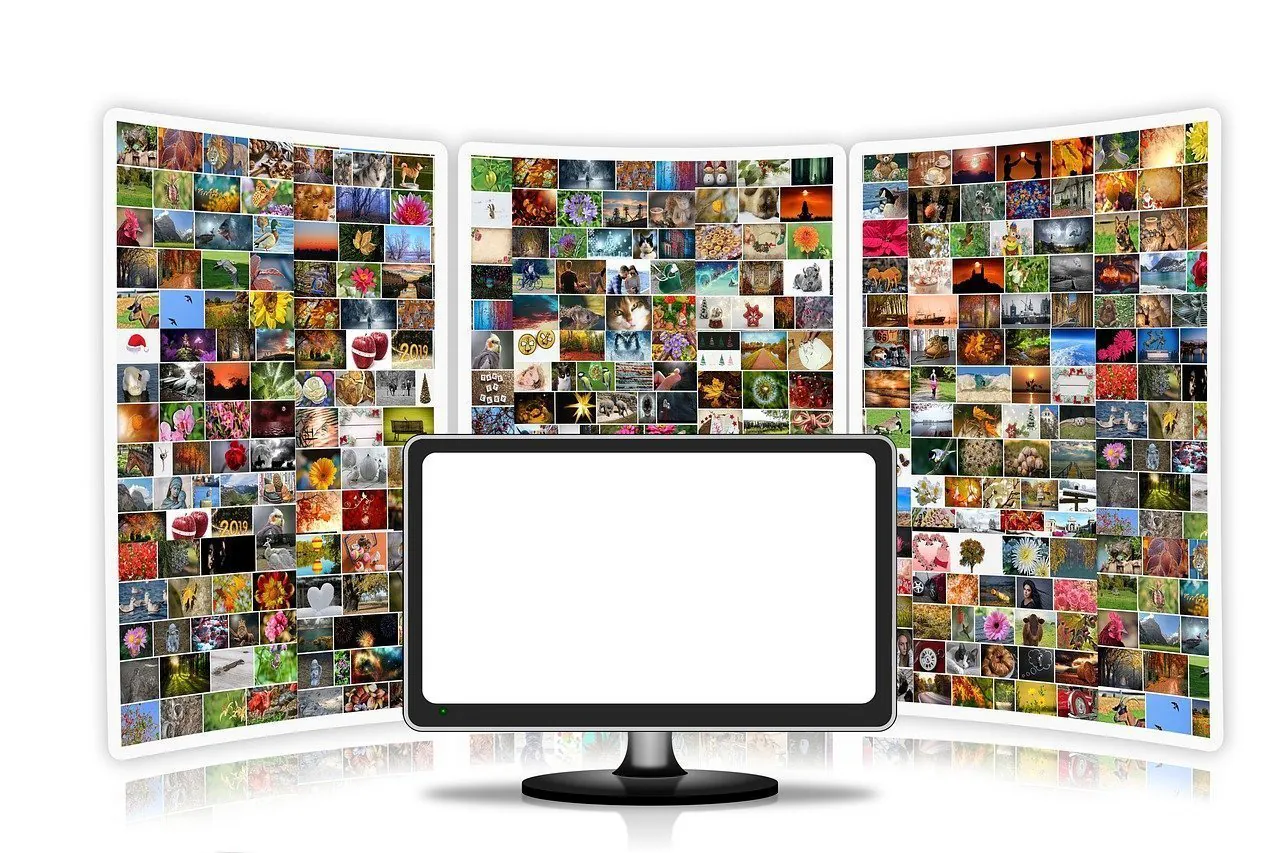
A new deep neural network architecture that can provide early diagnosis of systemic sclerosis (SSc) is being reported by the founding chair of the Biomedical Engineering Department at the University of Houston. SSc is a rare autoimmune disease that causes hardened or fibrous skin and internal organs.
The proposed network is implemented with a standard laptop computer, and it can immediately recognize the differences between images of healthy skin and skin with SSc.
The research was published in IEEE Open Journal of Engineering in Medicine and Biology.
Metin Akay is a John S. Dunn Endowed Chair Professor of biomedical engineering.
“Our preliminary study, intended to show the efficacy of the proposed network architecture, holds promise in the characterization of SSc,” Akay says.
“We believe that the proposed network architecture could easily be implemented in a clinical setting, providing a simple, inexpensive and accurate screening tool for SSc.”
SSc and Early Diagnosis
It is extremely important for SSc to be diagnosed early, but this is often difficult to achieve. Various studies demonstrate that organ involvement could take place much sooner than previously expected, occurring in the early phase of the disease.
Because it is so challenging for even physicians at expert centers to diagnose early and determine the extent of the disease progression, there are often long delays in therapy and treatment.
Training the System
Deep learning puts algorithms into layers, called the artificial neural network, which can make its own decisions. The researchers set out to speed up the learning process, so they trained the new network using the parameters of MobileNetV2, which is a mobile vision application. It is pre-trained with 1.4 million images from the ImageNet dataset. The training time only lasted less than five hours.
“By scanning the images, the network learns from the existing images and decides which new image is normal or in an early or late stage of disease,” said Akay.
Convolutional Neural Networks (CNNs), which are among deep learning networks, are often relied on in engineering, biology, and medicine. However, they still have not reached a high level of success in biomedical applications, as their use has been limited due to the size of training sets and networks available.
Akay, along with partner Yasemin Akay, combined the UNet, which is a modified CNN architecture, with added layers to overcome this challenge. They then developed a mobile training module, and the results demonstrated that the proposed deep learning architecture is more efficient and better than CNNs when it comes to classifying SSc images.
Yasemin Akay is a UH instructional associate professor of biomedical engineering.
“After fine tuning, our results showed the proposed network reached 100% accuracy on the training image set, 96.8% accuracy on the validation image set, and 95.2% on the testing image set,” said Akay.
The paper’s co-authors included Yong Du, Cheryl Shersen, Ting Chen, and Chanfra Mohan from the University of Houston. It also involved Minghua Wu and Shervin Assassi of the University of Texas Health Science Center (UT Health).
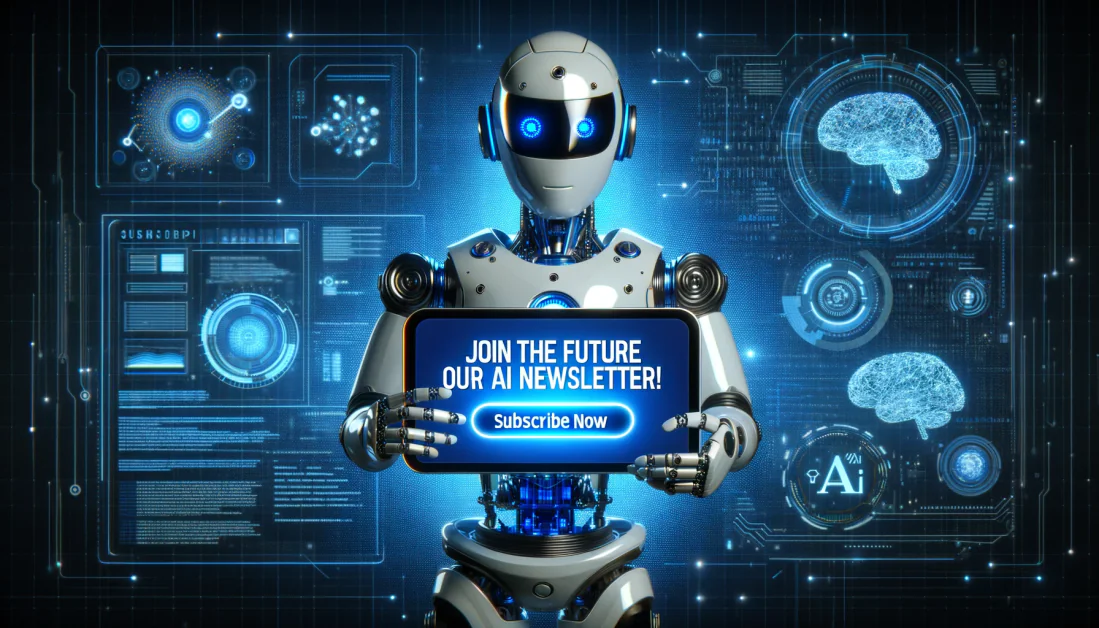
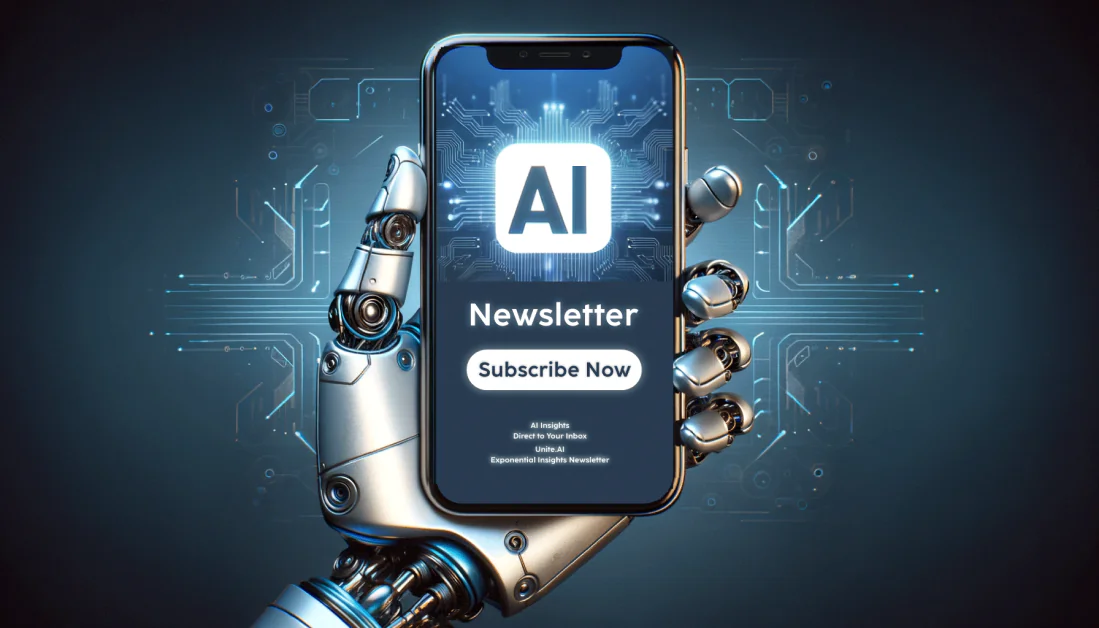