Interviews
Dave Ryan, General Manager, Health & Life Sciences Business at Intel – Interview Series
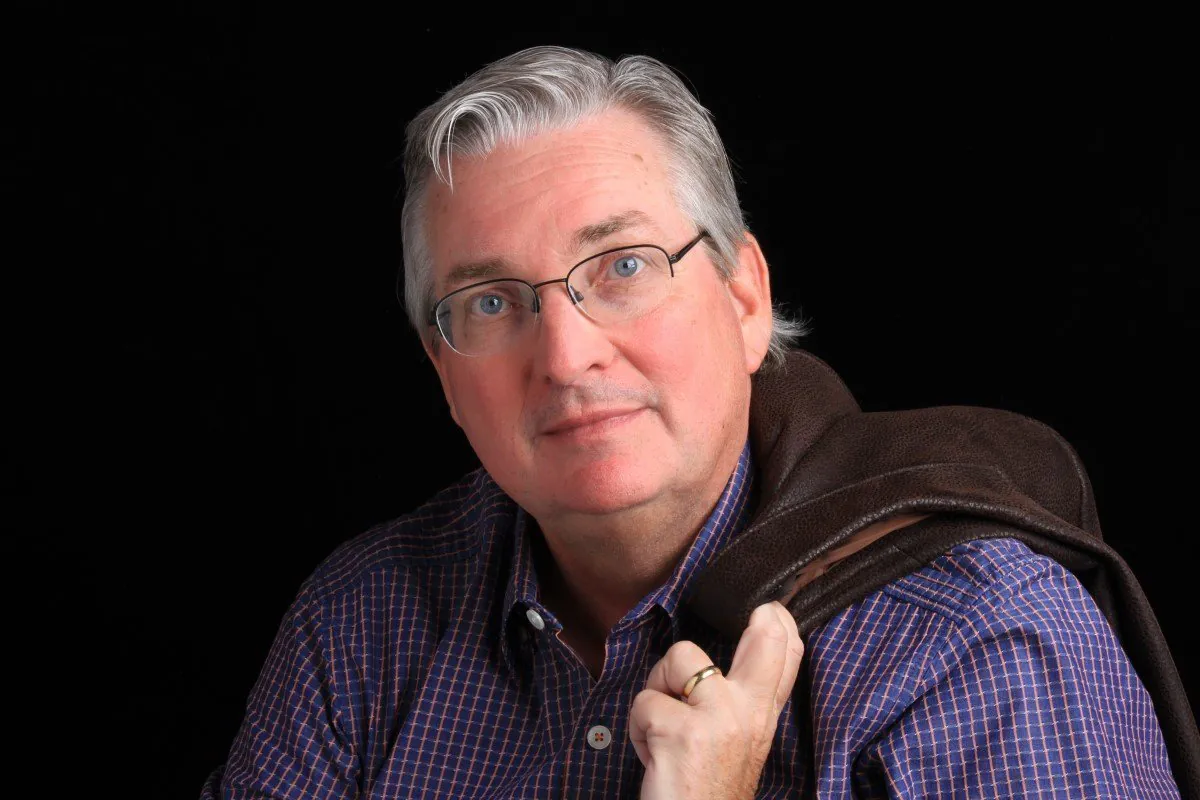
Dave Ryan leads the Global Health & Life Sciences business unit at Intel that focuses on digital transformation from edge-to-cloud in order to make precision, value-based care a reality. His customers are the manufacturers who build life sciences instruments, medical equipment, clinical systems, compute appliances and devices used by research centers, hospitals, clinics, residential care settings and the home. Dave has served on the boards of Consumer Technology Association Health & Fitness Division, HIMSS’ Personal Connected Health Alliance, the Global Coalition on Aging and the Alliance for Connected Care.
What is Intel’s Health & Life Sciences Business?
Intel’s Health & Life Sciences business helps customers create solutions in the areas of medical imaging, clinical systems, and lab and life sciences, enabling distributed, intelligent, and personalized care.
Intel’s Health business focuses on population health, medical imaging, clinical systems, and digital infrastructure.
- Population Health examines diverse patient data to give providers insights into risks for medical issues and improved treatments across cohorts. Optimized and tuned ML and AI helps “tier” groups, so payers and providers prioritize patients at most risk.
- Medical Imaging (e.g. MRI, CT), generate enormous data sets requiring accurate evaluation with no room for error. HPC and AI help more quickly scan image data and identify critical factors to assist radiologists in diagnosis.
- Clinical Systems use computer vision, AI, HPC and edge computing for patient monitoring, robotic surgery, and telehealth and many others. These intelligent systems reconcile diverse source data for a complete patient view and better diagnosis, with flexibility and scalability to support changing organizational needs.
- Digital Infrastructure integrates many technologies to enable novel approaches to patient interaction including anywhere anytime care where clinicians collaborate across space and time for condition management, surgery, and analytics.
Intel’s Lab and Life Sciences business is focused in 3 primary areas: Data Analytics, ‘Omics, and Pharma.
- Data Analytics uses AI to drive a cascade of discoveries and insights that help enable, among other things, precision medicine by ensuring that patients get the drugs that are most effective for them, and so reducing the risk of side effect profiles.
- ‘omics describes and quantifies biological molecule groups, using bioinformatics and computational biology. The massive data sets involved here require high-throughput processing to receive results within reasonable timeframes. With this throughput and new databases, toolkits, libraries, and code optimizations, ‘omics institutions can reduce time to results and development costs.
- Pharma is the study of drugs and how they interact with human biological systems, including at a molecular level where data science needs AI and ML to assist with lead generation and optimizations, target ID and preclinical research. This results in better clinical trials, smarter reaction insights and faster new drug discovery.
When did you personally initially become interested in using AI for the benefit of healthcare?
The proliferation of AI across many industries has largely been about automating those tasks routinely performed by humans. In healthcare, AI has become a tool through which we augment or assist, not replace, existing human expertise to deliver truly transformative approaches to diagnosis and treatment. And nowhere is this clearer than in medical imaging, in which data volume and complexity is both barrier and opportunity. Today, AI, and inferencing in particular, is able to perform more rapid and detailed scans of vast arrays of information than any human can and in so doing not only reveals insights previously hidden but also maximizes the valuable time of the radiologist to give reach a better diagnostic conclusion and for more patients. For example, AI solutions from customers help radiologists by analyzing data in X-rays which could indicate the presence of a collapsed lung (pneumothorax) or COVID. That is a truly remarkable achievement that is revolutionizing the efficacy of both medical imaging itself and how the human expertise is applied. Witnessing that kind of transformation in this one field naturally motivates one to seek out the next great leap in other health and life sciences pursuits where man and machine combine to produce a new whole so much greater the sum of the parts. Taking that a step further is the idea that AI can democratize knowledge across care disciplines and make scarce human expertise and experienced-based nuance go even further, raising the level of quality.
How important is AI to analyzing big data in a clinical setting?
The Health and Life Sciences industries generate more data with greater complexity than any other single industry in the world today. And unlike other industries, effectively managing and analyzing that data is a matter of life and death. Given these magnitudes, AI is now an indispensable enabler of a range of needs, both mundane and breakthrough, in both the clinical and lab settings to address the industry’s Triple Aim: Improve care quality and access while lowering costs.
For example, electronic health records (EHR) have enabled a digital revolution in the quality and efficiency of care delivery. Unfortunately, within these records is a messy mix of both unstructured and structured data which AI can help digitize into more unified and useful data sets. Optical character recognition (OCR) and natural language processing (NLP) are just two AI-enabled models that can convert the analogs of handwriting and voice into EHR data. And once digitized, AI can be applied across these data sets in many exciting use cases.
In other instances, data captured from medical devices and cameras is growing and, when combined with patient history data, analytics can help drive new insights to further personalize treatment. At a census level, many hospitals have already deployed algorithms that can predict sepsis onset for quicker intervention, and in ICUs, software can combine data across multiple isolated devices to create an impressively complete picture of that patient in near-real-time. Over time, all that captured and stored data can also be analyzed for better predictions in the future.
What are some of the more notable use cases that you are seeing for machine learning analyzing this data?
As mentioned above, NLP tools can help replace manual scribing or data entry to generate new documents, like patient visit summaries and detailed clinical notes. This enables clinicians to see more patients, and providers to improve documentation, workflow, and billing accuracy by entering orders and documentation sooner in the day.
More broadly, AI-enabled analytics help providers understand and manage a wide range of clinical applications that improve efficiency and lower costs. This allows hospitals to better manage resources and fine tune best practices, and care teams to collaborate on diagnoses and coordinate treatments and overall care they deliver to improve patient outcomes.
Clinicians can analyze for targeted abnormalities using appropriate ML approaches and filter out structured information from other raw data. This can lead to quicker and more accurate diagnosis and optimal treatments. For example, ML algorithms can convert the diagnostic system of medical images into automated decision-making by converting images to machine readable text. ML and pattern recognition techniques can also draw insights from massive volumes of clinical image data, unmanageable by human alone, to transform the diagnosis, treatment and monitoring of patients.
To assess and manage population health, ML algorithms can help predict future risk trajectories, identify risk drivers, and provide solutions for best outcomes. Deep learning modules integrated with AI technologies allow the researchers to interpret complex genomic data sets, to predict specific types of cancer (based on the gene expression profiles obtained from various large data sets) and identify multiple druggable targets.
Could you elaborate on how Intel is collaborating with the genomics community to transform large datasets into biomedical insights that accelerate personalized care?
Precision medicine supplies individual-level health data sources that enable better selection of disease targets and identification of patient populations that demonstrate improved clinical outcomes to novel preventative and therapeutic approaches.
Genomics is the cornerstone of this precision medicine. It provides the blueprint of who we are, and why and how we are unique which is critical for providers to understand as they combine this information with other data (images, clinical chemistry, medical history, cohort data, etc.). Clinicians use this information to develop and deliver patient-specific treatments that are lower risk and more effective.
Intel is collaborating with the genomics community by optimizing the most commonly used genetic analysis tools used in the industry to run best and across Intel architecture-based platforms and the processors that power them. For example, optimization of the Broad Institute’s industry leading genetic variant software, the Genomic Analysis Toolkit (GATK), on Intel hardware using OpenVINO to ease AI model development debug and scalable deployment, highlights our impact and commitment to this industry. The GATK toolkit provides benefits to biomedical research such as Genomics DB which efficiently stores files ~200GB in size (typical for genomic datasets) and the Genome Kernel Library running AVX512 which takes advantage of specific Intel architecture hardware instructions to accelerate genomic workloads and AI utilization.
Accelerating the speed and reducing the cost of genomic analysis while maintaining the accuracy of that analysis, continues to be compelling to biomedical and other life sciences researchers as they use Intel compute solutions to discover and harness new medical insights.
Could you discuss why you believe that remote healthcare is so important?
The Health industry has been working on various forms and aspects of remote care for many years. The reasons for this have been, up until recently, an intuitive and hoped for belief that remote care can be for many care delivery situations, as good as or better than traditional in-clinic models. Now spurred by the pandemic crisis and its impact, health care delivery systems around the world are forced to adopt telehealth or collapse. This sudden rush to implement is now proving those long held beliefs to be true and care at a distance to be both vital and highly viable.
Remote care has many benefits. Patient comfort and satisfaction with telehealth care delivery is rising rapidly. They are able to remain calmer and at ease in their home with less disruption and time/schedule impact. Providers like it because it allows them to see more patients, and better manage their own time and better allocate scare clinical resources. And of course, what has become the clearest and most compelling reason these past few months for everyone is the inherent ability of remote care to limit contagion and the need for in-person contact when a video chat with augmented device and compute telemetry can get most care delivery tasks done just as well.
Can you discuss some of the technologies that are currently being used for remote patient monitoring?
There are several critical technology elements. The most important is ease of use for the patient, quickly followed by security and privacy of the data, and the robustness of the application and the data it captures. For example, we need to prevent a user from deleting a monitoring app from her iPad by accident.
Another critical aspect for a care provider deploying across multiple patients is fleet management and the ability to send updates or tech support down the wire and tailored to each user or cohort of user. This requires:
- standardization of the data exchange and privacy with industry standards such as FHIR and Continua;
- secure and power-efficient compute platform to orchestrate the data and communicate it back to the clinician including appropriate software and encryption;
- connectivity through a cellular network to make the user devices stand-alone and not dependent on Wi-Fi at home that may be unreliable or even non-existent;
- cloud storage and analytics on the backend.
In addition, the ability to gather and aggregate the data flowing in from users is fundamental to enabling clinicians to do patient monitoring and support, and for the software and analytics to inform care teams of a nominal state or initiate an alarm notification for results that are out of tolerance.
We believe that AI will play a much larger role in patient monitoring moving forward, improving the patient experience through natural voice surveys (“How are you feeling today?”, “Your blood pressure seems a bit high”) and allowing care teams to better understand a patient’s health and identify appropriate treatments. Through the use of AI models, population health management will also progress with all patient data folding into ever larger data sets which improve accuracy of an iterative learning model. This is essential for remote monitoring at scale.
What are some of the problems that need to be overcome to increase the success rate of remote healthcare?
Many of the same issues that plague our current system of traditional care delivery are also factors in enhancing or inhibiting the success of remote care. These include societal sub-segment beliefs and stigmas surrounding healthcare, or socio-economic barriers stemming from lack of insurance, technology fluency, required devices, and connectivity. Data silos prevent maximizing value that larger shared data sets could produce especially now that our ability to harness learning programs is truly emerging.
But there are challenges that are unique to remote care:
- policy and payment issues, though much improved of late, must continue their positive momentum to expand with relaxed restrictions on what is allowable and reimbursable under via remote care modality;
- financial challenges and lack capital to invest in technology in health care requires a conversion from a CapEx model to an OpEx model. Rather than investing in facilities and capex equipment, providers can shift to a “pay as you go” model, foregoing the need for a lot of fixed infrastructure and, like phone service, pay for the minutes (or data) used;
- user experience, for both patient and provider, must continue to improve, ultimately to where the technology disappears into the background, and the capabilities are intuitive and seamless and the process compelling with equivalent or better outcomes and cost structures.
Ultimately, we want the technology to support the provision of care, not get in the way of it. If we are successful (and we believe we are and will continue to be), then the technology truly will allow a bridge to tomorrow’s better model of remote care delivery, making the best possible case for the normalization of remote care as standard of care delivery.
Thank you for the fantastic interview, I enjoyed learning more about Intel's health efforts. Reader's who wish to learn more should visit Intel's Global Health & Life Sciences business.
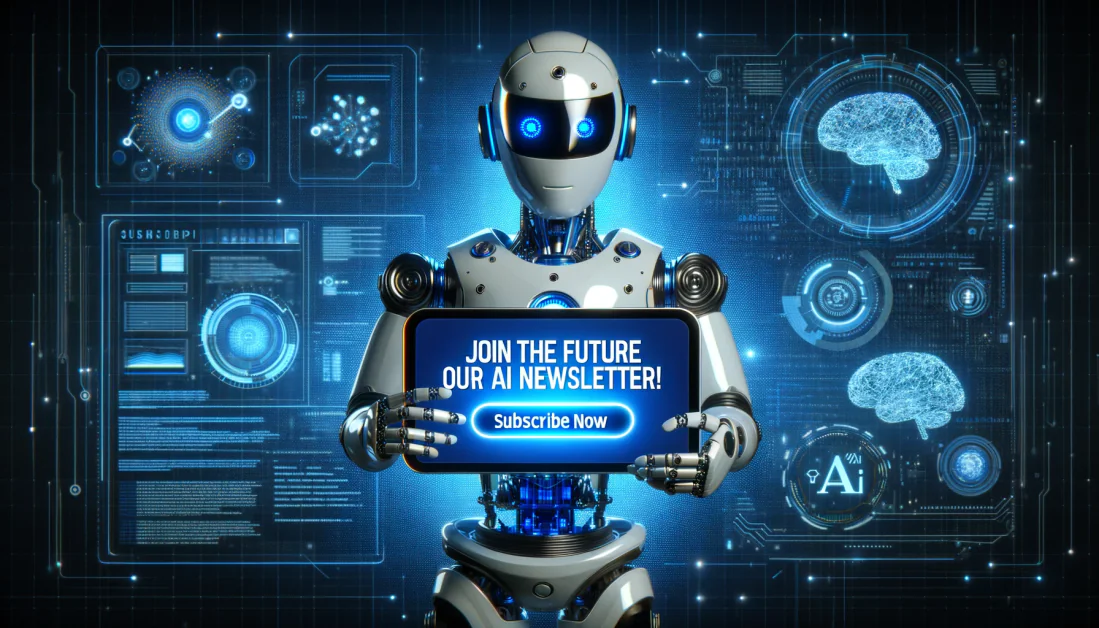
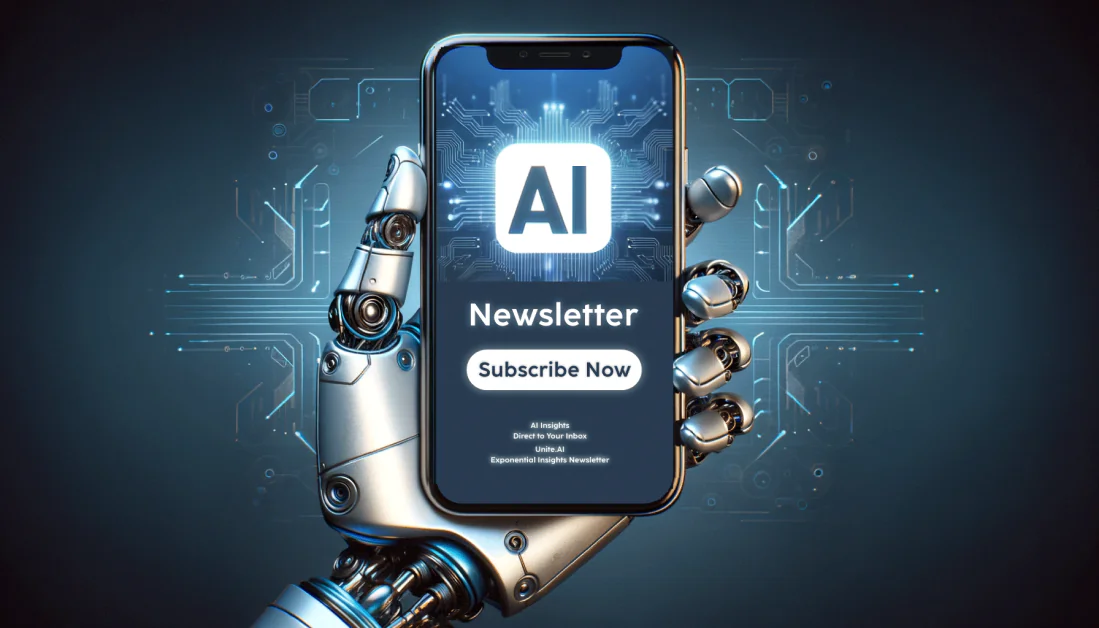