Interviews
Dave Excell, Founder of Featurespace – Interview Series
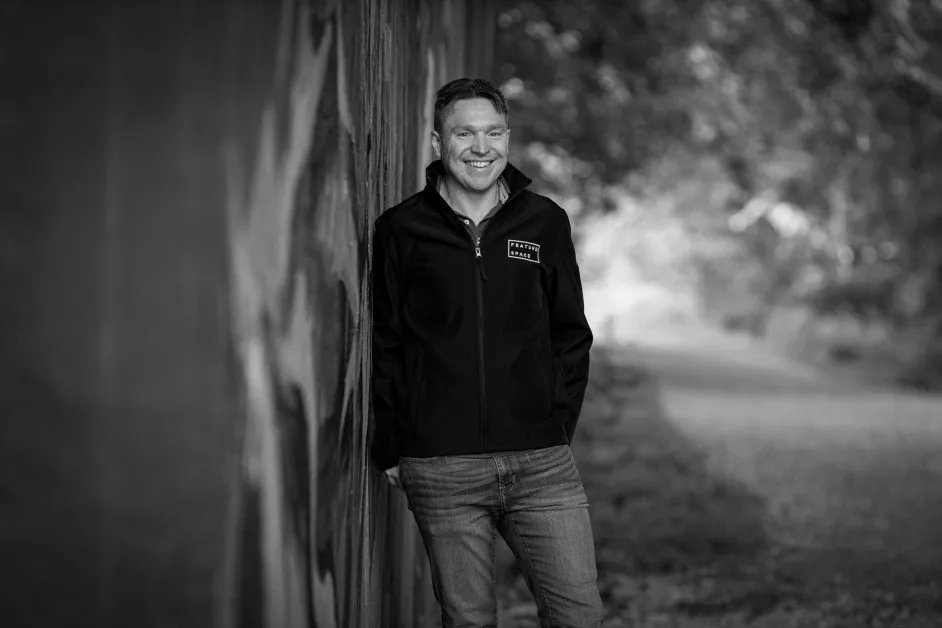
Dave Excell is the Founder of Featurespace, Dave founded Featurespace following his invention of Adaptive Behavioral Analytics, which uses explainable AI to help banks recognize and flag suspicious consumer behavior. Even in recent times as consumer behavior shifts, this advanced AI has been able to curb fraud and help authorities tackle money laundering and other organized financial crime while bringing trust back to fintech.
Could you share with us the story of how in collaboration with Professor Bill Fitzgerald you came up with the concept of Adaptive Behavioral Analytics?
While pursuing my PhD, I worked with Professor Bill Fitzgerald at the University of Cambridge to apply machine learning and statistical techniques to understand human behavior. During my time there, organizations would come to us seeking novel solutions to various challenges they had automating effective decision making from data they captured or to improve efficiency in manual processes. I began to notice a pattern: organizations across industries struggled with understanding the underlying behavior or ‘intent’ behind the data they captured, especially when trying to identify bad actors. For example, with one organization we modeled the decision making of players within a computer game to understand if they were genuine players or robots cheating the system. The more projects we did, the more I saw this need for machine learning that would adapt as the behavior (and data) behind the outcome (e.g. cheating or fraudulent activity) would change to avoid detection. This is actually how I first came up with the concept of Adaptive Behavioral Analytics, which later became the first fundamental technology within Featurespace.
Could you share the genesis story of how this concept then led to the launch of Featurespace?
Although I do enjoy researching and finding solutions, I don’t enjoy research just for research’s sake. I’m motivated by applying technology to practical problems, then finding ways to deliver commercial value and deploying the technology to make a positive impact on the world that we live in. That is how I ended up founding Featurespace and we’ve been on a mission since to make the world a safer place to transact.
Could you discuss the existing techniques that are applied towards fraud and financial crime prevention, and why these techniques fall short?
There have been various tech applications in the space for a while–in fact, the first uses of AI to fight financial fraud date back to the early 1990s. However, that primitive version of AI assumed that fraud behaviors would stay the same. The algorithms were built to recognize the same fraudulent behavior over and over. This same theory is broadly applied in anti-fraud technology to this day. But fraud isn't static. Fraudsters are constantly adapting their methods to stay ahead of anti-fraud technology. That’s why at Featurespace, we created the world’s first adaptive AI model to fight fraud. We stay three steps ahead of fraudsters without requiring any human intervention.
Why is Adaptive Behavioral Analytics so impactful compared to these legacy fraud prevention techniques?
Our proprietary Adaptive Behavioral Analytics are so impactful compared to legacy fraud prevention techniques because legacy players rely on static fraud patterns–but fraud is never static. Legacy players learn what different types of known bad behavior looks like, then set out to detect those bad behaviors among millions of transactions. The problem is that those models can only take into account bad behaviors that have been seen before, and fraudsters are constantly adapting their methods to stay ahead of fraud prevention. Instead, our Adaptive Behavior Analytics model learns what good behavior looks like, then detects changes against those good behaviors. There is much more good behavior being done in the world than bad, giving us more to learn from good behavior. There is a much smaller set of fraudulent behaviors, and they are constantly changing. To try to detect only known fraudulent behaviors is a losing game.
What are the different types of machine learning algorithms that are used?
Featurespace’s Adaptive Behavioral Analytics uses a combination of unsupervised and supervised machine learning techniques. Unsupervised techniques are used to identify changes in behavior to indicate likely risk. Supervised techniques are subsequently used to optimize the accuracy of our models to prevent and detect fraud and financial crime. Last year Featurespace launched Automated Deep Behavioral Network models that utilize a novel Recurrent Neural Network architecture. Featurespace Research developed Automated Deep Behavioral Networks to automate feature discovery and introduce memory cells with native understanding of the significance of time in transaction flows, improving upon the market-leading performance of our existing Adaptive Behavioral Analytics.
How adaptive are the models to learning new consumer behavior and optimizing customer profiles?
Our Adaptive Behavioral Analytics models are exactly as adaptable as they need to be–even in the face of unprecedented change. For example, during the initial COVID-19 lockdowns in 2020, consumer purchasing behavior changed literally overnight. By April 29, 2020, Mastercard saw a 40% increase in contactless payments. Non-adaptive fraud prevention AI models were thrown for a loop, blocking legitimate payments being made by people ordered to stay at home. Our models adapted automatically, without human intervention. This is most evident through the TSYS Foresight Score, a fraud- and risk-management decisioning scoring tool for payments issuers, built by TSYS and Featurespace. From January-June 2020, the TSYS Foresight Score with Featurespace consistently delivered stable score distributions on a weekly basis, enabling consumers ordered to stay home to continue purchasing groceries and other essentials with no interruption.
What are the biggest use cases for this technology?
This technology is specifically geared toward banks, financial institutions and payments processors. For example, payments processing company Worldpay was recently recognized for its FraudSight product powered by Featurespace for its ability to mitigate fraud while increasing merchants’ approval rates and protecting consumers.
Is there anything else that you would like to share about Featurespace?
Scams are one of the fastest growing fraud categories in the world. Regulators are recognizing this and attempting to put protections in place. For example, the UK government launched a reform of the Online Safety Bill in March 2022 in an effort to prevent scams and increase consumer confidence in online transactions. Similarly in the US, the Consumer Financial Protection Bureau (CFPB) is considering taking action to protect consumers against scams by putting more responsibility on banks and credit unions. By preventing scams before they happen, Featurespace can save banks money and keep their customers safe, automatically without human intervention.
An example of this is NatWest, the fourth largest UK bank in terms of total assets, with around 19 million customers. NatWest saw an increase in the value of fraud and scams detected, including an immediate decrease in false positive rates (genuine customer activity declined), within just 24 hours of deploying Featurespace’s ARIC Risk Hub. As a result of our partnership, they’ve cited Featurespace as a “strong partner” to their investors.
Thank you for the great interview, readers who wish to learn more should visit Featurespace.
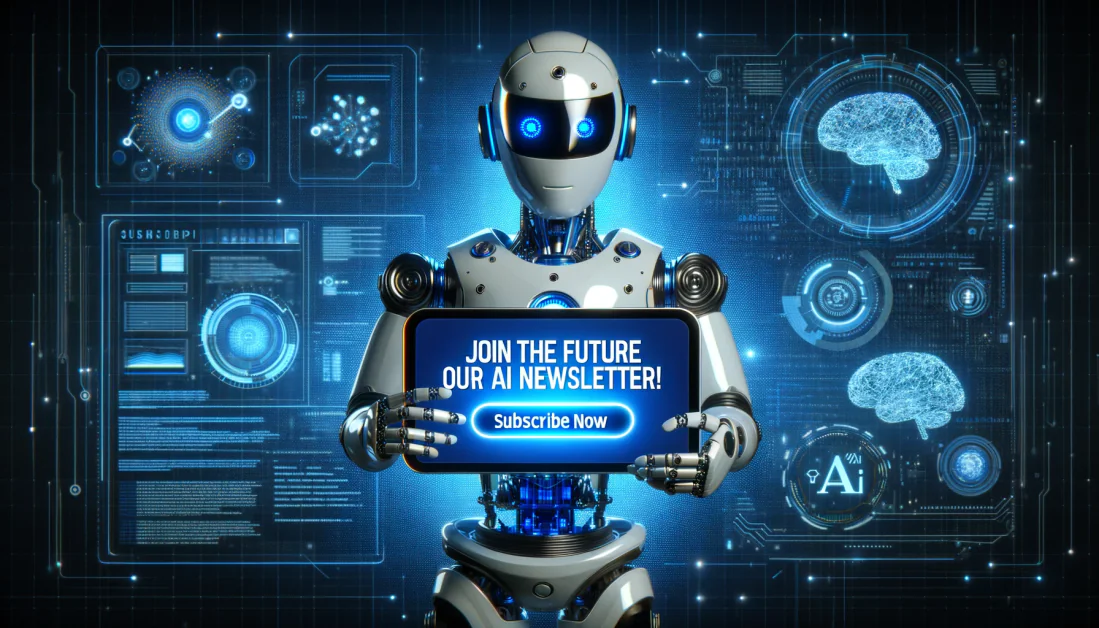
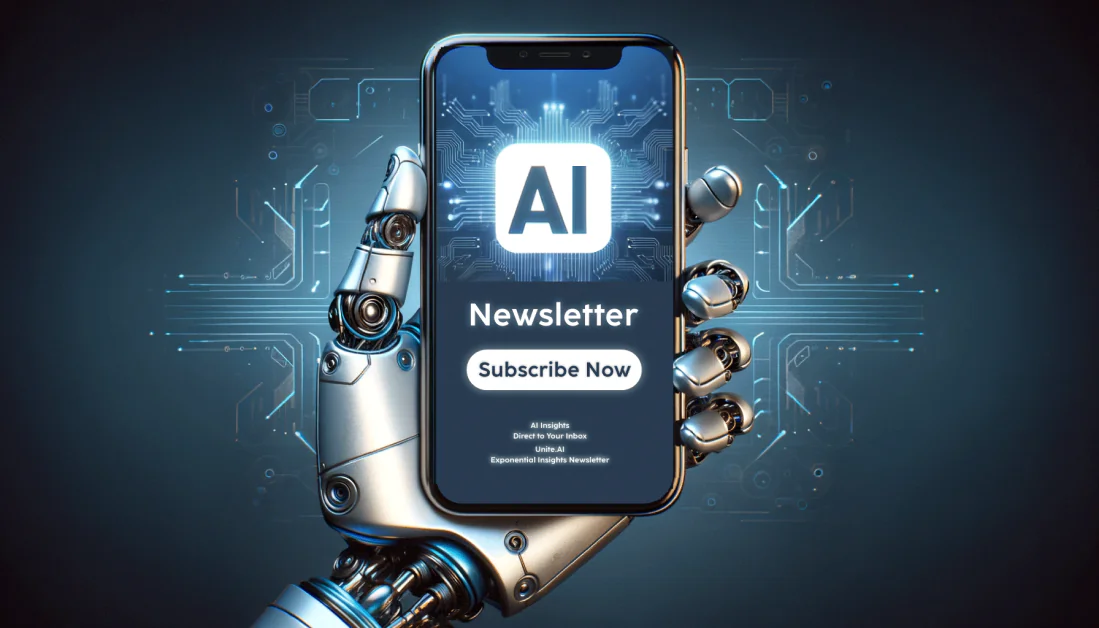