Thought Leaders
CPGs – It’s Time to Look More Closely at Your Data
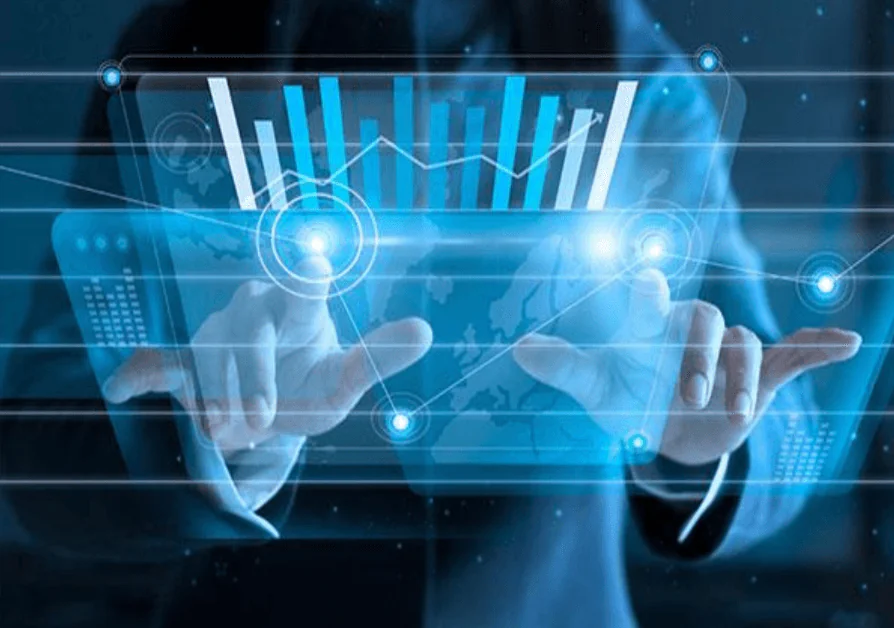
What do traditional demand planning and the advertising cookie have in common? They are both getting replaced by something more sophisticated. Why? Because in each case, they are missing the right data and analytics which will mislead you on the way to understanding your consumer.
Demand planning and the reliance on historical sales data
Traditional demand management starts with historical sales and shipment data across all customers over some time period for a baseline revenue and unit forecast. The forecast is done at different levels of aggregation depending on the stock keeping unit (SKU) and the number of distribution centers (DCs) and factories involved with that SKU. This forecast is then massaged between sales, marketing and finance functions to arrive at what is thought to be a reasonable, achievable growth target based on past performance. This is used to create manufacturing plans, often on a monthly schedule (e.g., SKU by factory by month). The production plan, ideally in combination with DC-level forecasts, then drives replenishment planning for deliveries from a factory to a DC. All to deliver a reasonable, consensus plan.
However, demand planners have come to recognize that historical sales data is not enough to see what consumers need today or tomorrow. The impact of the pandemic, and the supply chain challenges that followed, made this very apparent. In addition, direct to consumer purchasing options have only made the planning process more complicated. Past performance data does not reflect near-term changes in consumer behavior, nor can it keep up with rapid shifts in consumer behavior and supplier disruptions.
But where’s the analogy to the advertising cookie?
For over 20 years, CPG advertisers leaned on third-party cookies to achieve advertising scale and to practice a kind of performance-driven marketing that guided their advertising spend. Rather than negotiate deals with media sites one-by-one, without any data to confirm the value of one site over another, cookies and programmatic marketing evolved advertising into something that promised to be more easily quantifiable and justifiable. According to Matt Naeger, who heads US strategy for the performance marketing agency Merkle, “We became a little bit dependent on third-party cookies because it was easier, faster, and required less planning and integration [than traditional marketing].”
However, even before the rise of privacy rules and ad blockers, the accuracy of cookie data came into question. Consumers were given the option to clear their cookie caches, which served to undercount what might have been true consumer interest. And in the opposite direction, the prevalence of bots that could fabricate gobs of phony ad traffic seriously overcounted real interest.
The lack of actionable results and over-reliance on cookies to gauge interest led Stephen Pretorius, CTO at UK-based ad agency WPP, to state “I’m not particularly sad about the demise of third-party cookies because they were never really that accurate, never really that useful, and in fact I think this whole thing has helped us all to rethink what data matters.”
As far as the cookie goes, new and more sophisticated approaches – that protect personal identity but still identify potential buyers – are coming to the fore. Approaches like browser-based cohort assignments, activity-versus-personal-identity-assigned IDs, and first-party data – are being actively explored. In addition, AI and machine learning models can now provide insights that help make the ads themselves measurably more effective – attracting, rather than tracking consumers.
For demand planning, the analogy is similar. The heart of demand planning is predicting consumer demand and deciphering every demand driver that shapes consumer demand. Historical data and shipments were never a great source of information, and inherent bias between sales, marketing, and finance wouldn’t generate a better forecast. Overconfidence in these beliefs failed to capture the shifts and impact of near-term and local conditions. And just like the cookie, more data, better sources, and new processes combined with AI and machine learning provide a better path forward.
What’s the resolution for demand planning?
Demand planners are taking a closer look at the accuracy of their planning forecasts as a result of the volatility and complexity in today’s markets. Three areas in particular stand out as contributors to forecast inaccuracy:
- the absence of models that use real-time point-of-sale (POS) and other data sources that would better tune the forecast to current demand-impacting factors;
- machine learning that uncovers the right level and/or grouping at which to execute a forecast for greatest aggregate accuracy; and
- the AI modeling methodologies to account for the lost sales that didn’t make it into future demand consideration at all.
Once again, better data and data science can address these challenges. CPG firms are creating a new layer of forecasting expertise that augments their planning process with AI and machine learning insights based on a wider range of data and advanced modeling techniques.
Areas of investment include:
- Demand Sensing – leveraging near-term data – including order status, recent sell-through data, retailer inventory, promotion execution, retail pricing, product and location-specific attributes, social media sentiment, and inventory cost factors – in models that are more frequently run to improve short-term demand response with recent insights and frequent actions;
- Inventory Optimization – sophisticated machine learning approaches to assess probabilities of lost or excess sales in forecast data and consideration of those insights in order to maximize fill-rate; and
- Dynamic Aggregation – an AI approach that overcomes the high level of data variability at more disaggregated levels that can impact forecast accuracy at higher aggregated levels.
These new approaches, and more, are serving to make demand forecasts more accurate, more easily reactive, and considerably less volatile. In addition, they contribute to the demand planning business process by automating what would be laborious manual spreadsheet tasks, easily scaling to incorporate a greater range of internal and external data, and, due to the built-in learning aspect of these models, contributing to continuous improvement over time.
There’s no escaping it: a world where attracting customers can be accomplished via one tracking mechanism – and supplying what they need tomorrow can be based simply on what was bought in the past – is disappearing. The opportunity for greater and more permanent sales growth lies in digging deeper into the data to learn what the consumer is all about. For more information about AI-powered forecasting, planning and pricing solutions, click here.
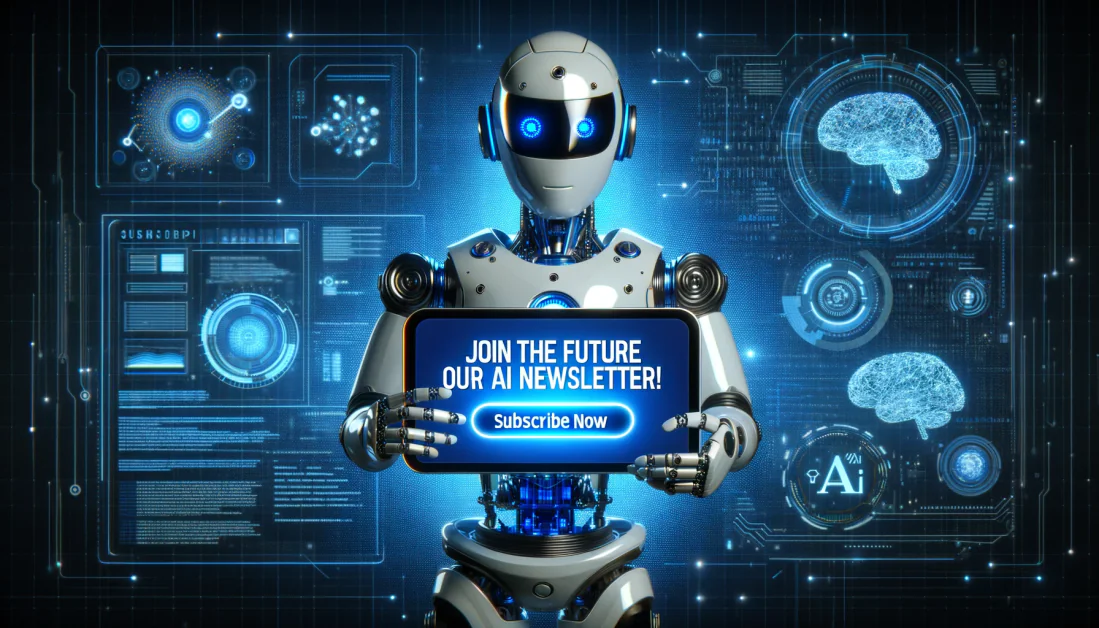
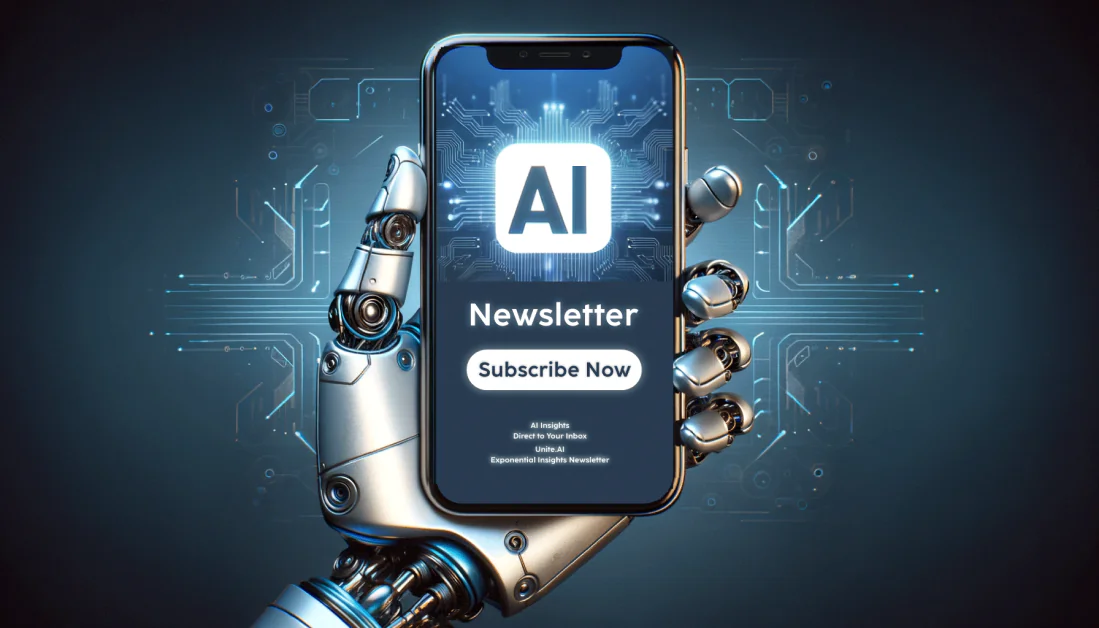