Artificial Intelligence
Computer Able to Identify 200 Species of Birds from One Photo
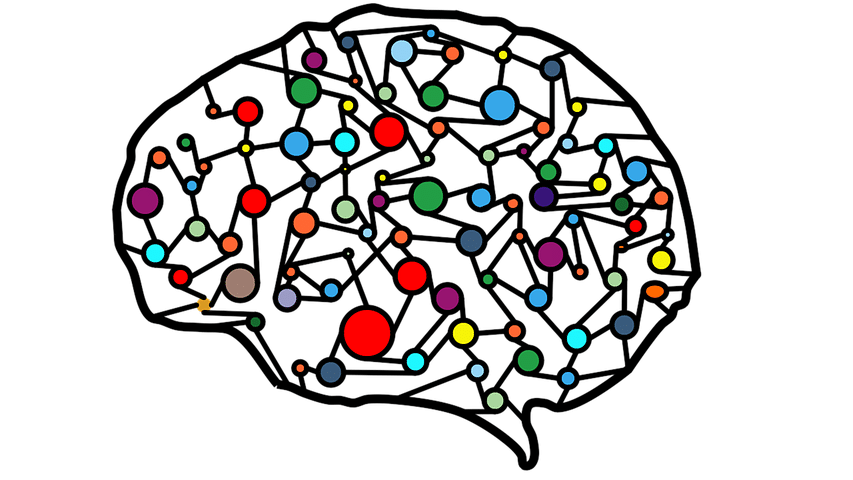
Researchers from Duke University used machine learning in order to train a computer to identify up to 200 different species of birds. The computer only requires one photo to complete the identification process. For a human, years of birdwatching is often required to be able to identify different species from each other.
The research was led by Duke computer science Ph.D. student Chaofan Chen, along with undergraduate Oscar Li. It was also worked on by other team members of the Prediction Analysis Lab directed by Duke professor Cynthia Rudin.
A.I. Showing Its Thinking
While the identification process is impressive, there is a more important aspect of the developments. The A.I. is able to show its thinking, allowing even an inexperienced bird watcher to understand the process.
The deep neural network, or algorithms that are based on the workings of the brain, were trained with 11,788 photos. The photos included 200 different species of birds, including everything from ducks to hummingbirds.
The team of researchers did not have to specifically train the network to identify beaks or wing feathers. Instead, the network is able to take a photo of a bird and identify certain patterns in the image. It can then take those patterns and identify previous patterns that it already encountered in typical species traits.
According to the team, the network then creates a series of heat maps that identify certain traits. For example, it can tell the difference between an ordinary warbler and a hooded warbler, along with the different features like a masked head and yellow belly. It then shows that these features are what led to the identification.
Unlike Other Systems
The neural network was able to identify the correct species up to 84% of the time. This is similar to some of the best-performing systems. The difference is that those systems don’t explain the thinking process like this one.
According to Rudin, this project’s most revolutionary aspect is that it provides visualization for what deep neural networks see when they look at an image.
This technology is also currently used on social media sites, to identify suspected criminals in surveillance cameras, and help autonomous vehicles identify traffic lights and pedestrians.
Deep learning software often does not require to be explicitly programmed in order to learn from data, which is not the case for traditional software. However, the process is not always clear or shown, so it is often difficult to explain how the algorithms “think” when classifying an image.
In the Future
Rudin and others are currently working on new deep learning models for A.I., pushing the field forward. The new models can explain their reasoning and identification process. This helps the researchers see from start to finish, and it makes it easier to identify the reason behind a mistake or problem.
Rudin and her team will work on using the algorithm in the medical field. It could identify certain problem areas within medical images like mammograms. This would help medical professionals detect lumps, calcifications, and other signs of breast cancer.
According to Rudin, the network mimics the way doctors make a diagnosis.
“It’s cased-based reasoning,” Rudin said. “We’re hoping we can better explain to physicians or patients why their image was classified by the network as either malignant or benign.”
The team will present a paper including their research at the Thirty-third Conference on Neural Information Processing Systems (NeurlIPS2019) in Vancouver on December 12.
The study also includes authors Daniel Tao and Alina Barnerr of Duke and Jonathan Su at MIT Lincoln Laboratory.
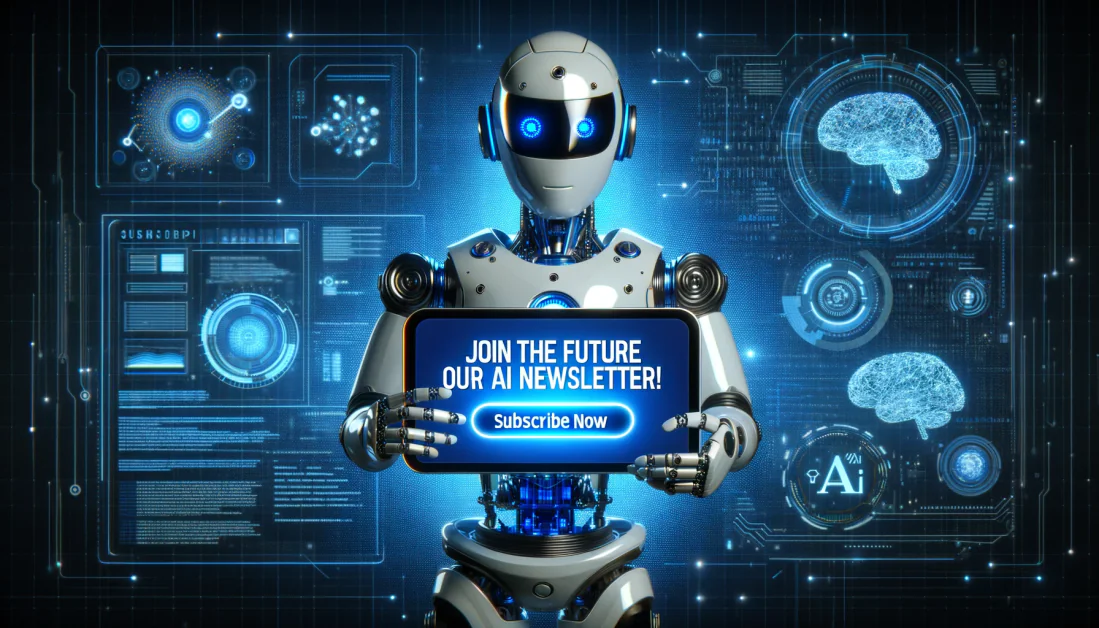
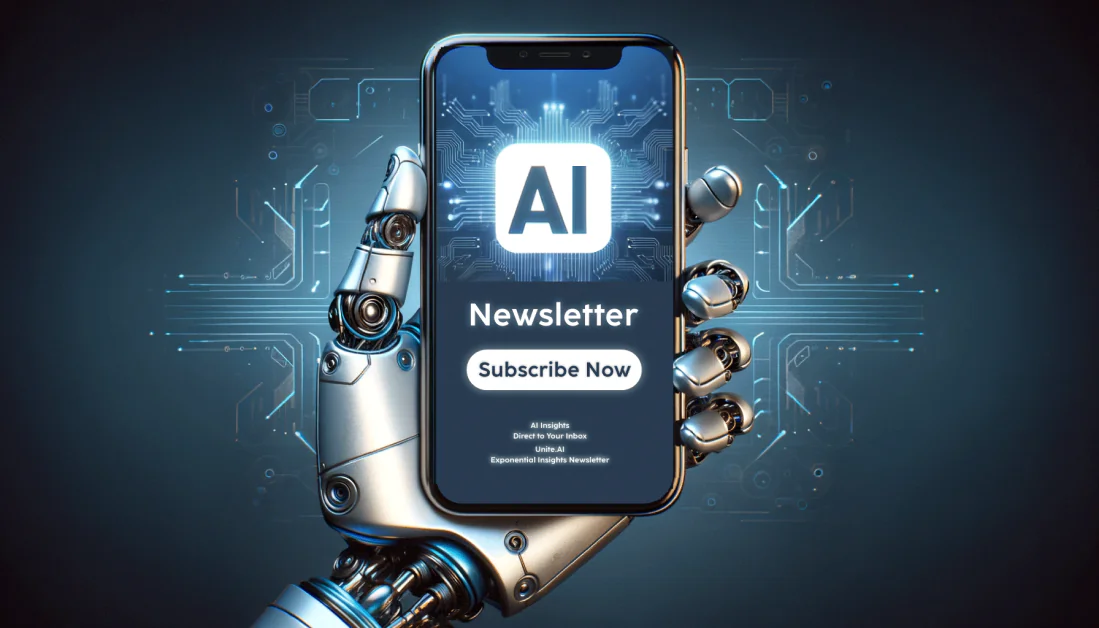