Healthcare
AI Model Detects Parkinson’s From Breathing Patterns
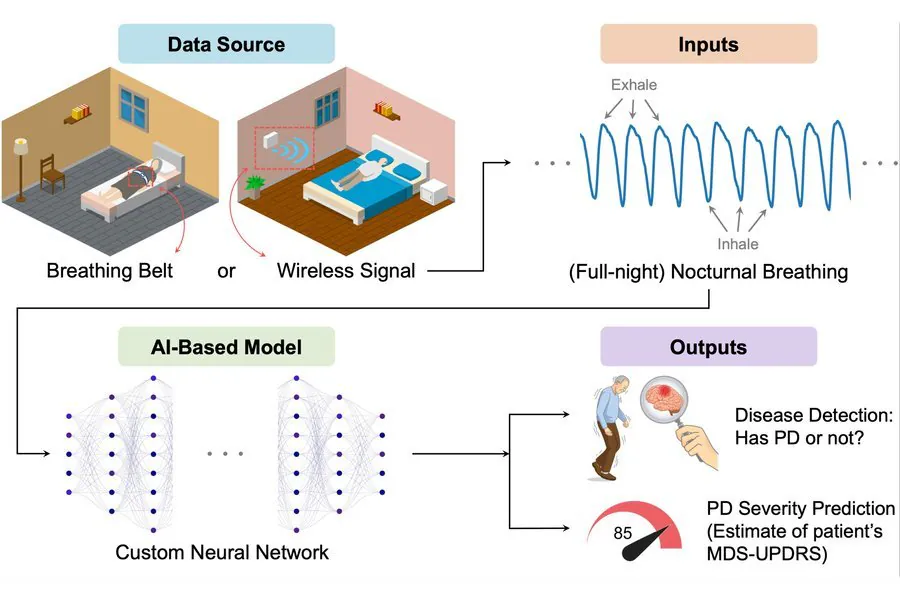
A team of researchers at MIT has developed an artificial intelligence (AI) model that can detect Parkinson’s from reading a person’s breathing patterns.
The neural network is able to assess a person’s nocturnal breathing, or sleeping breathing patterns, to determine whether or not they have Parkinson’s. It was trained by MIT PhD student Yuzhe Yang and postdoc Yuan Tuan, and it can determine the severity of someone’s Parkinson’s disease while tracking its progression over time.
Yang is the first author of the new research paper, which was published in Nature Medicine.
The entire team included Dina Katabi, the Thuan and Nicole Pham Professor in the Department of Electrical Engineering and Computer Science (EECS), and principal investigator at MIT Jameel Clinic.
Katabi, who is senior author, is also an affiliate of the MIT Computer Science and Artificial Intelligence Laboratory and director of the Center for Wireless Networks and Mobile Computing.
Researchers have been consistently investigating the potential of detecting Parkinson’s with cerebrospinal fluid and neuroimaging, but these methods are invasive and costly. They also require access to specialized medical centers.
AI Assessment Every Night
The team of researchers set out to overcome these challenges and demonstrated that the AI assessment of Parkinson's can be carried out every night at home. The person can even be asleep without touching their body.
The researchers developed a device that looks like a home Wi-Fi router, and it emits radio signals, analyzes their reflections off the surrounding environment, and extracts the subject’s breathing patterns without any bodily contact. The breathing signal is fed to the neural network to assess Parkinson’s, with zero effort form the patient and caregiver.
“A relationship between Parkinson's and breathing was noted as early as 1817, in the work of Dr. James Parkinson. This motivated us to consider the potential of detecting the disease from one's breathing without looking at movements,” Katabi says. “Some medical studies have shown that respiratory symptoms manifest years before motor symptoms, meaning that breathing attributes could be promising for risk assessment prior to Parkinson's diagnosis.”
According to Katabi, the study has important implications for drug development and clinical care.
“In terms of drug development, the results can enable clinical trials with a significantly shorter duration and fewer participants, ultimately accelerating the development of new therapies. In terms of clinical care, the approach can help in the assessment of Parkinson's patients in traditionally underserved communities, including those who live in rural areas and those with difficulty leaving home due to limited mobility or cognitive impairment,” she says.
Ray Dorsey is a professor of neurology at the University of Rochester and co-author of the paper. He is a Parkinson's specialist and says that the study is likely one of the largest sleep studies ever carried out on Parkinson’s.
“We've had no therapeutic breakthroughs this century, suggesting that our current approaches to evaluating new treatments is suboptimal,” says Dorsey. “We have very limited information about manifestations of the disease in their natural environment and [Katabi's] device allows you to get objective, real-world assessments of how people are doing at home. The analogy I like to draw [of current Parkinson's assessments] is a street lamp at night, and what we see from the street lamp is a very small segment … [Katabi's] entirely contactless sensor helps us illuminate the darkness.”
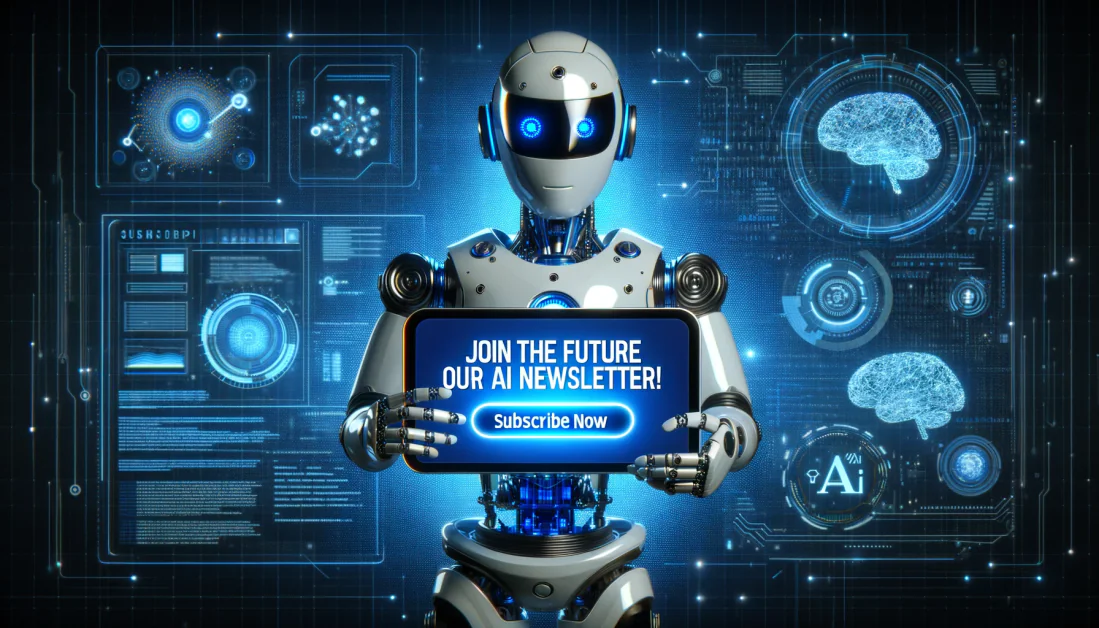
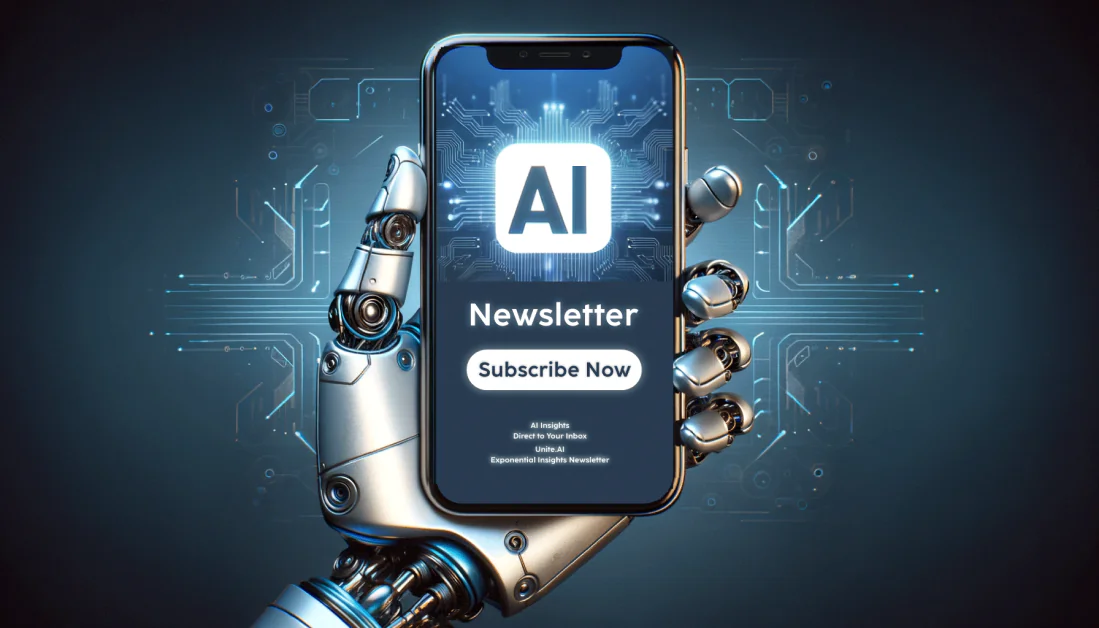