Artificial Intelligence
AI Model Can Predict How Much Students Are Learning
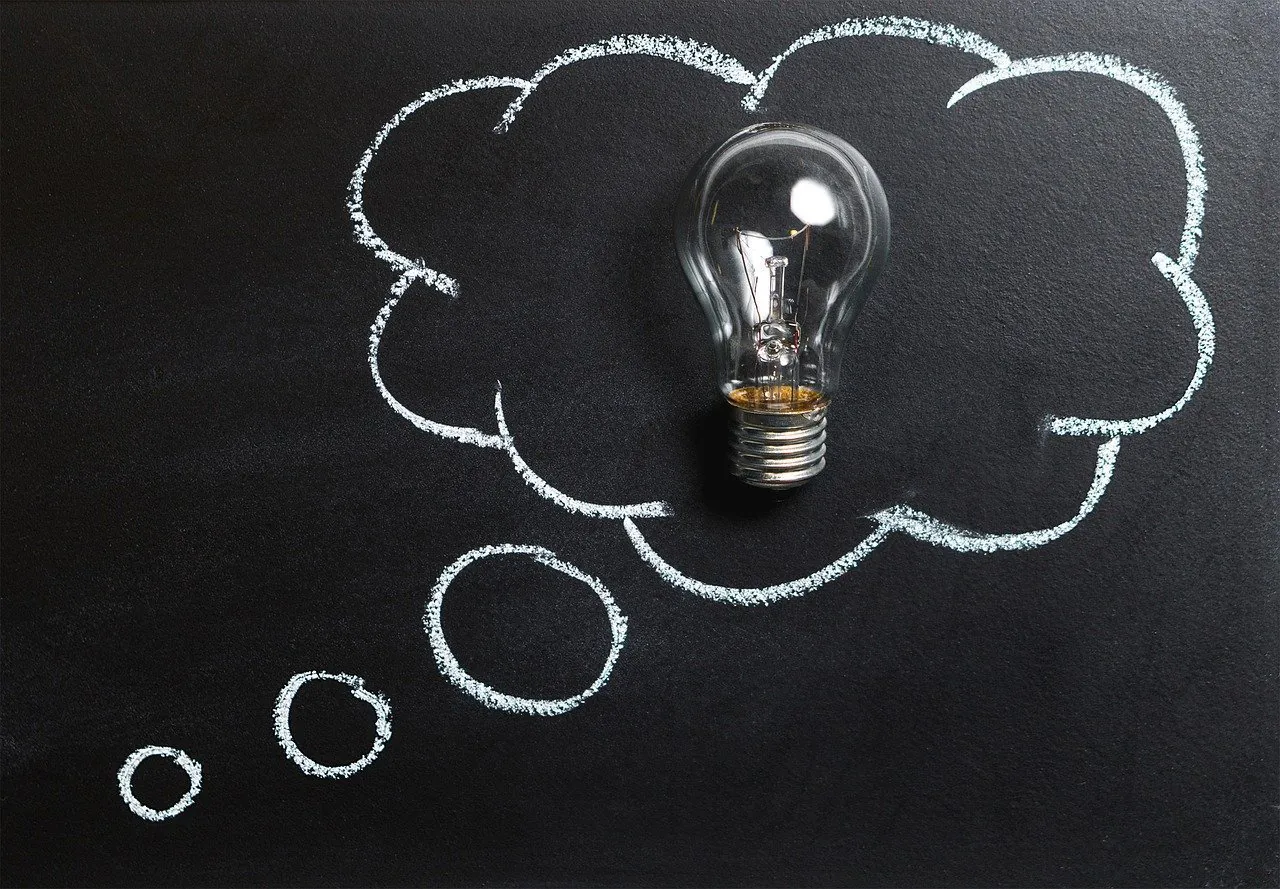
Researchers from North Carolina State University have developed an artificial intelligence (AI) model that is capable of predicting the amount students are learning in educational games. The model relies on multi-task learning, an AI training concept where one model performs multiple tasks. The system can help improve instruction and learning outcomes.
Jonathan Rowe is the co-author of the paper detailing the work and a research scientist in North Carolina State University's Center for Educational Informatics (CEI).
“In our case, we wanted the model to be able to predict whether a student would answer each question on a test correctly, based on the student's behavior while playing an educational game called Crystal Island,” says Rowe.
“The standard approach for solving this problem looks only at overall test score, viewing the test as one task,” he continues. “In the context of our multi-task learning framework, the model has 17 tasks — because the test has 17 questions.”
The researchers used gameplay and testing data from 181 students. The AI analyzed the gameplay of each student and how they answered Question 1 on the test. The AI learned the common behaviors of the students who answered Question 1 correctly, and it then learned the behaviors of those who answered it incorrectly. With this data, the AI was able to determine how a new student would answer Question 1.
The function is performed at the same time for every question. While the gameplay that is reviewed for a student is the same, the AI studies the behavior in the context of Question 2, Question 3, etc.
The multi-task approach was successful and made a difference. The multi-task model was around 10 percent more accurate than the other models that used conventional AI training methods.
Michael Geden is the first author of the paper and a post-doctoral researcher at NC State.
“We envision this type of model being used in a couple of ways that can benefit students,” he says. “It could be used to notify teachers when a student's gameplay suggests the student may need additional instruction. It could also be used to facilitate adaptive gameplay features in the game itself. For example, altering a storyline in order to revisit the concepts that a student is struggling with.
“Psychology has long recognized that different questions have different values,” Geden continues. “Our work here takes an interdisciplinary approach that marries this aspect of psychology with deep learning and machine learning approaches to AI.”
Andrew Emerson is the co-author of the paper and a Ph.D. student at NC State.
“This also opens the door to incorporating more complex modeling techniques into educational software — particularly educational software that adapts to the needs of the student,” Emerson says.
The paper is titled “Predictive Student Modeling in Educational Games with Multi-Task Learning,” and it will be presented at the 34th AAAI Conference on Artificial Intelligence that is set to take place between Feb. 7-12 in New York, N.Y. The co-authors of the paper were James Lester, Distinguished University Professor of Computer Science and director of CEI at NC State, as well as Roger Azevedo of the University of Central Florida.
The work was supported by the National Science Foundation and the Social Sciences and Humanities Research Council of Canada.
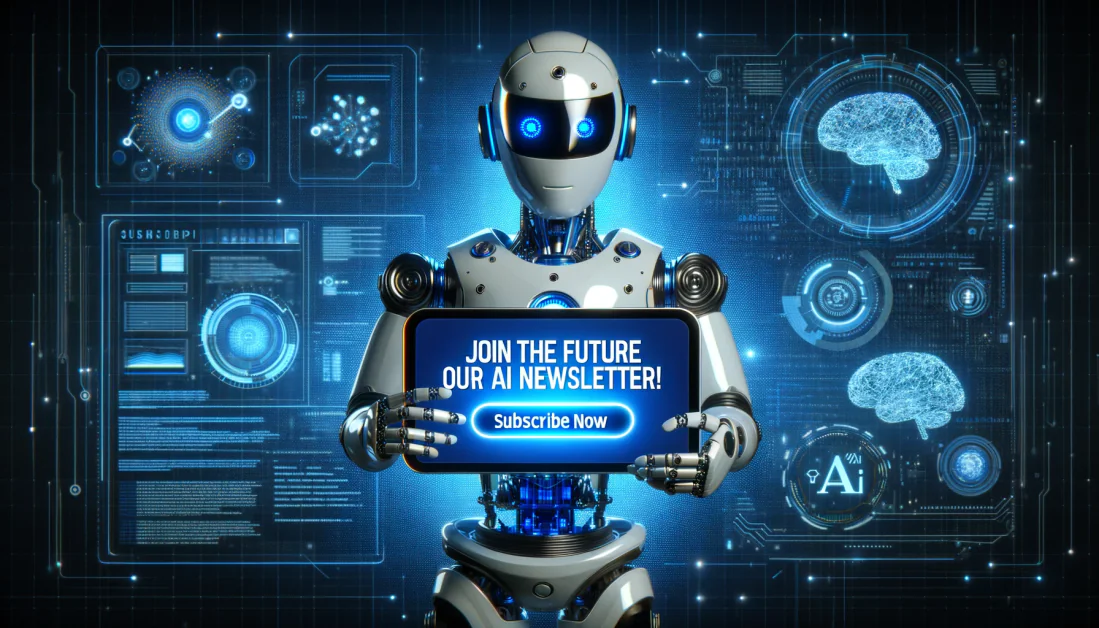
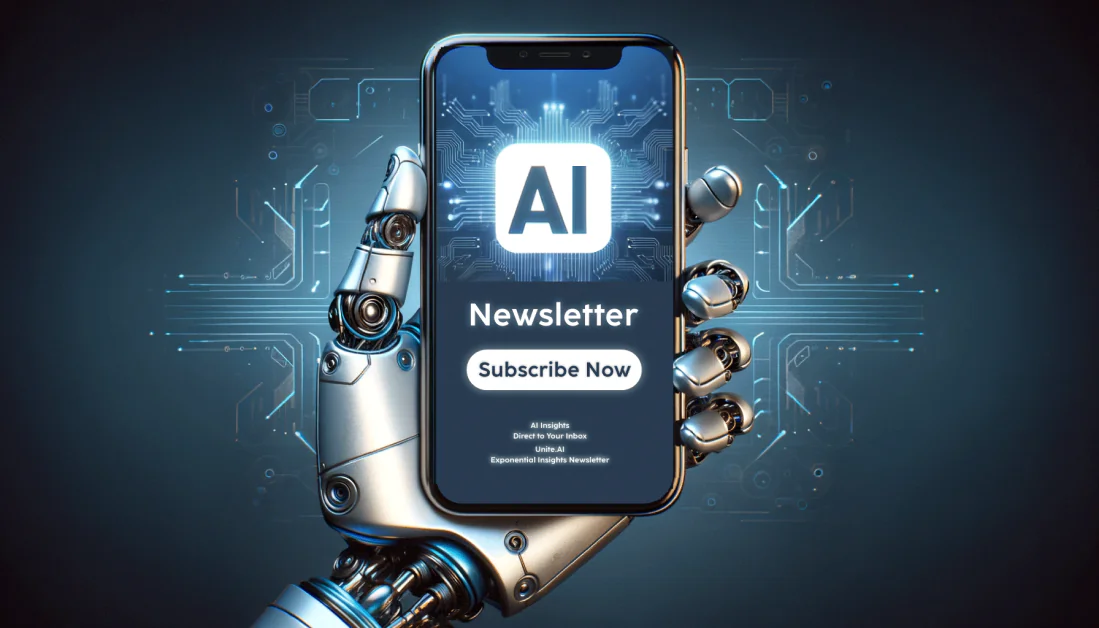