Artificial Intelligence
AI Developed to Translate Brain Activity into Words
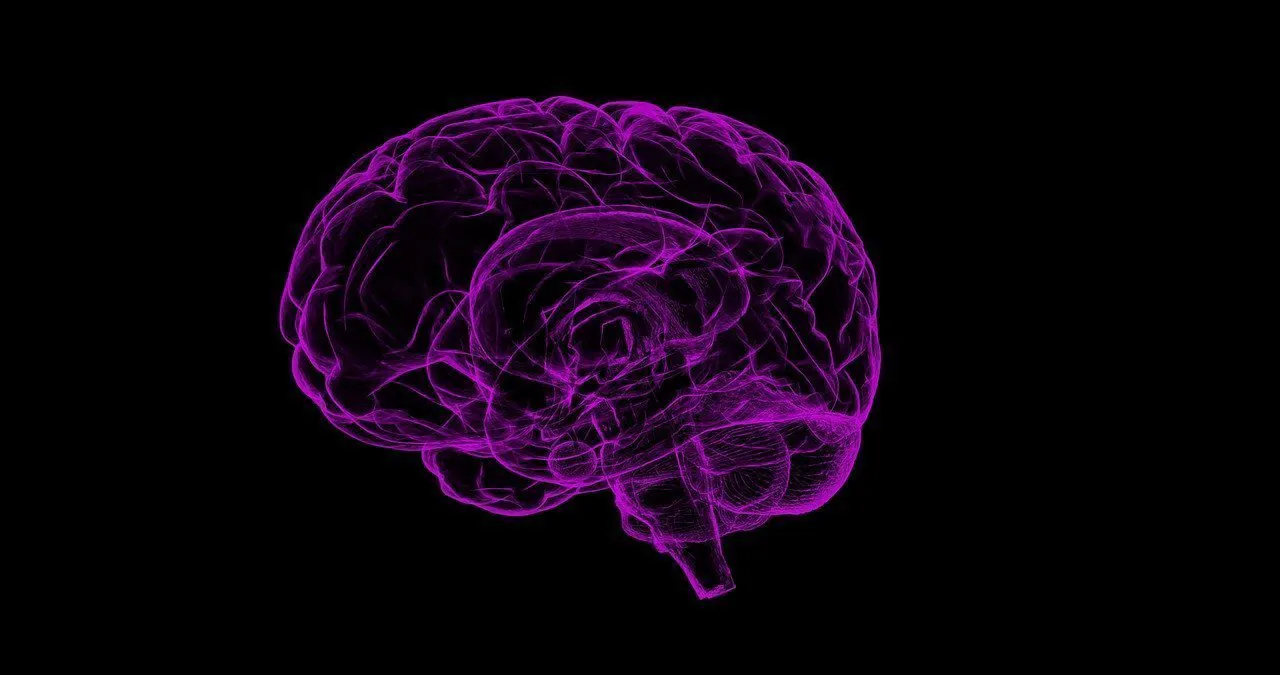
Researchers at the University of California, San Francisco have developed artificial intelligence (AI) that can translate brain activity into text. The system works on neural patterns that are detected when someone is speaking, but experts hope that it can eventually be used on individuals who are unable to speak, like people suffering from locked in syndrome.
Dr. Joseph Makin was co-author of the research.
“We are not there yet but we think this could be the basis of a speech prosthesis,” said Makin.
The research was published in the journal Nature Neuroscience.
Testing the System
Joseph Makin and his team relied on deep learning algorithms to study the brain signals of four women as they spoke. All of the women have epilepsy, and electrodes were attached to their brains to monitor seizures.
After the electrodes were attached, each woman then read aloud a set of sentences while her brain activity was measured. The largest amount of unique words used was 250. They could choose from a set of 50 different sentences, including “Tina Turner is a pop singer,” and “Those thieves stole 30 jewels.”
The brain activity data was then fed to a neural network algorithm, and it was trained to identify regularly occurring patterns. These patterns could then be linked to repeated aspects of speech like vowels or consonants. They were then fed to a second neural network that attempted to convert them into words to form a sentence.
Each woman was asked to repeat the sentences at least twice, with the final repetition not making it into the training data. This allowed the researchers to test the system.
“Memorising the brain activity of these sentences wouldn’t help, so the network instead has to learn what’s similar about them so that it can generalise to this final example,” says Makin.
Results
The first results from the system did not make sentences that made sense, but it improved as the system compared each sequence of words with the sentences that were read aloud.
The team then tested the system by generating written text only from the brain activity during speech.
There were a lot of mistakes in the translation, but the accuracy rate was still very impressive and much better than previous approaches. Accuracy varied from person to person, but for one individual only 3% of each sentence on average needed corrections.
The team also learned that the training algorithm on one individual’s data allowed the final user to provide much less.
According to Dr. Christian Herff, who is from Maastricht University but not involved in the study, it is impressive that the system required less than 40 minutes of training data for each participant and a limited collection of sentences, compared to the millions of hours normally required.
“By doing so they achieve levels of accuracy that haven’t been achieved so far,” he said.
“Of course this is fantastic research but those people could just use ‘OK Google’ as well,” he said. “This is not translation of thought [but of brain activity involved in speech].”
Another challenge could be that people with speech disabilities might have different brain activity.
“We want to deploy this in a patient with an actual speech disability,” Makin says, “although it is possible their brain activity may be different from that of the women in this study, making this more difficult.”
There is still a long way to go to translate brain signal data comprehensively. Humans use a massive amount of words, and the study only used a very restricted set of speech.
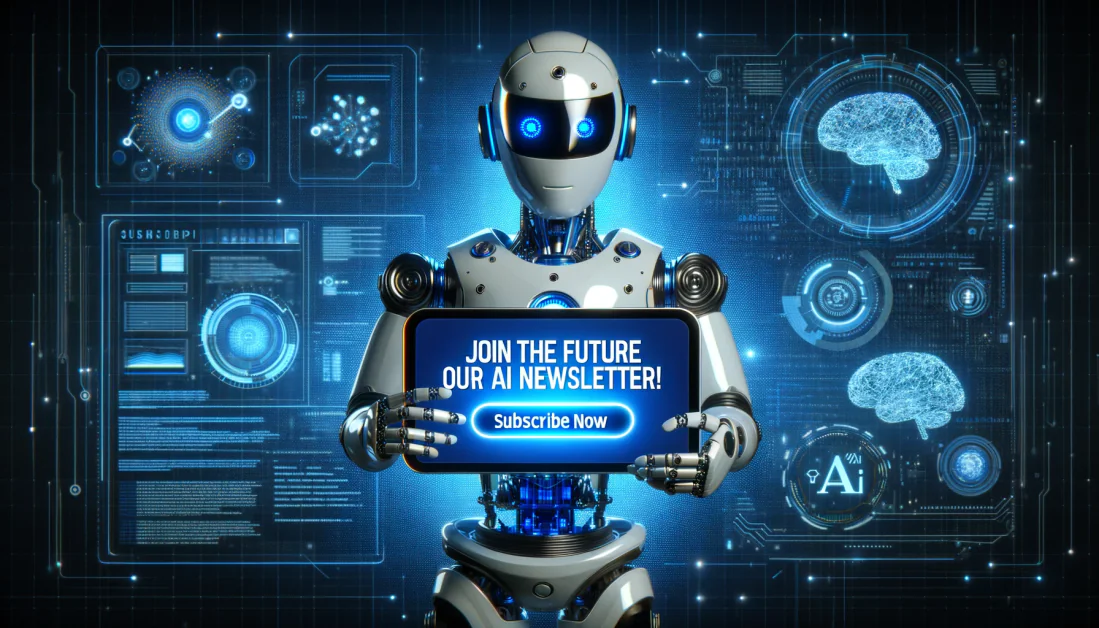
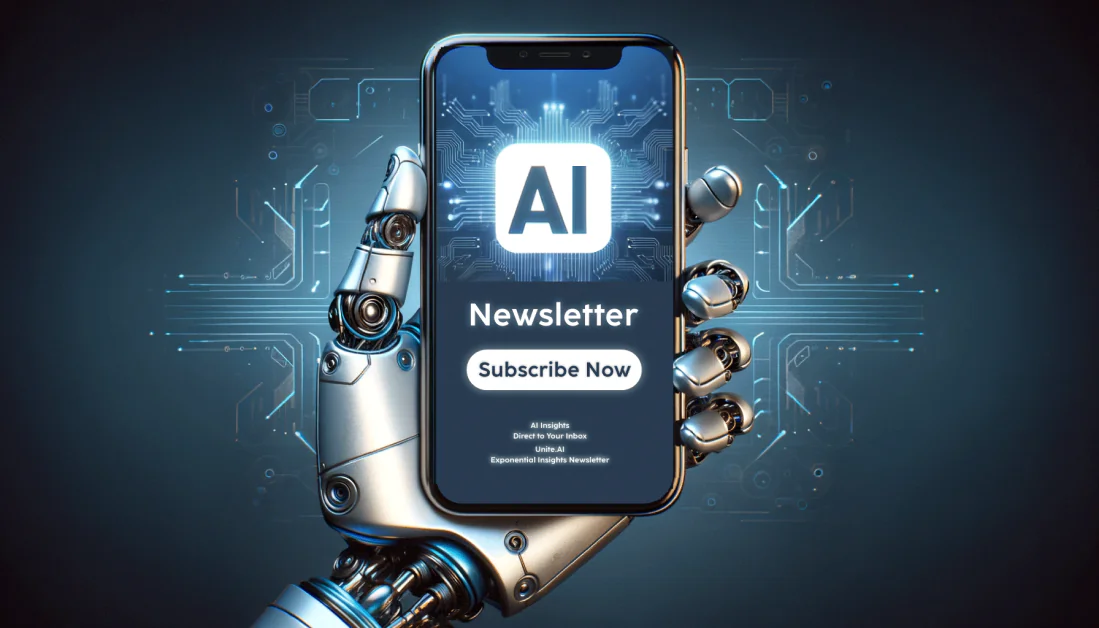