Thought Leaders
AI: By the People, for the People and of the People
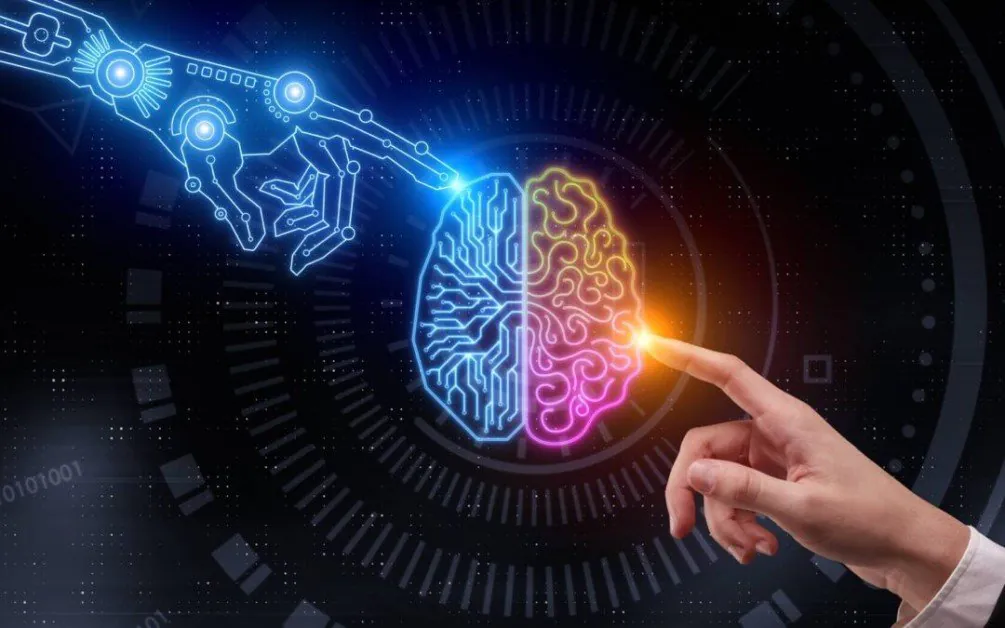
By: Balakrishna (Bali) D R, Senior Vice President, Service Offering Head – ECS, AI and Automation at Infosys.
We are fortunate to live in the age of technology where Artificial Intelligence works so hard to make our lives easier – our phones now recognize us and will unlock as they ‘see’ us, we have talking maps that help us find the shortest, least crowded paths to get us where we want to go, smart devices that warm and cool our homes even before we say the word, intelligent apps that predict and prevent fraud, and so much more.
However, we also have had instances where these smart AI-powered systems have failed us from a fairness and ethics perspective. For example, a reputed bank that works with us suspected that the AI models they use to evaluate credit worthiness before issuing loans might be biased, and they called on us to help them. On another occasion, a machinery manufacturer worked with us to analyze warranty claims patterns to eliminate bias, from the data set and process, before reengineering and automating their claims approval process. Recruitment related AI models, we often find, are corrupted by biases of age, gender, race, even zip codes sometimes, in their data sets – delivering unfair outcomes if left unchecked.
Due to the tremendous impact AI bias can have throughout the enterprise, ethical questions have taken center stage in how AI systems are developed, implemented, and used. AI systems are the amalgamation of many human decisions, which inherently are also based on human biases. The protection of employee autonomy and privacy, risks of biases impacting career growth and opportunities, discrimination based on skin color, race or gender, the lack of explainability of choices made by AI solution, and thereby, accountability of AI decision making, are being hotly debated when it comes to discussing AI and its merits.
Creating Responsible AI
AI bias can have a ripple effect throughout the entire organization. it is pertinent that IT leaders ensure they are deploying AI in an ethical manner that works with, and not against, employees. To do so, organizations should look to include the following in their AI deployments.
Data Governance: Ethical use of AI is also predicated on well-governed use of data, starting from sourcing data ethically and transparently. To do this, IT leaders should establish a well-defined governance framework that will ensure data security, integrity, and privacy as well as prevent data corruption and loss – are all necessary.
Accountability: Machine Learning models need to be fair, unbiased, treat people equally and also share benefits equitably (similar acceptance and rejection rate) across all attributes such as race, religion, gender etc. They need to achieve acceptable accuracy not just on the overall figure but also on the minority classes. These models should be explainable when it comes to outlining how the outcome is arrived at. For example, being able to explain why the model rejected a loan application from applicant A but approved the same application from applicant B. IT leaders developing AI solutions need to make the underlying logic that drives these decisions clear to business stakeholders so there is overall greater transparency within the enterprise.
Adversarial Robustness: The entire AI ecosystem needs to agree on the need for AI models to be tested and hacked through simulations to study potential adverse outcomes. For example, IT leaders can use the following tests to check their AI systems and prepare for potential obstacles.
- Modify data: directly change the dataset used for training by data injection, modification and logic corruption
- Modify models: test for confidence reduction and misclassification
- Auxiliary tools: use tools to influence or corrupt the results
Human in the Loop: AI models need to be de-risked by having humans in the loop for all key decisions points and having an effective backup mechanism or an alternate path if the AI system needs to be pulled back.
Developing social consent for AI
In addition to these guidelines, we need to promote inclusive discussions and deliberations between all leaders across the enterprise, about benefits, interests, costs and consequences of any large AI deployment. Only such inclusive processes involving all stakeholders, to discuss and decide acceptable outcomes, and weigh out the risks and benefits, is likely to win AI technologies the license to operate throughout the enterprise.
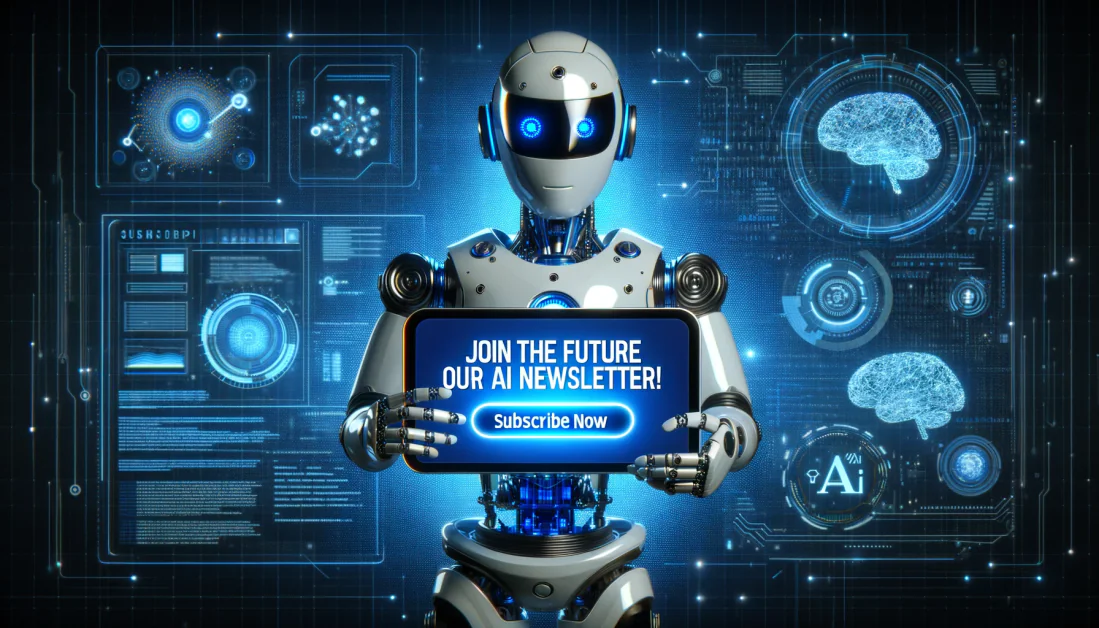
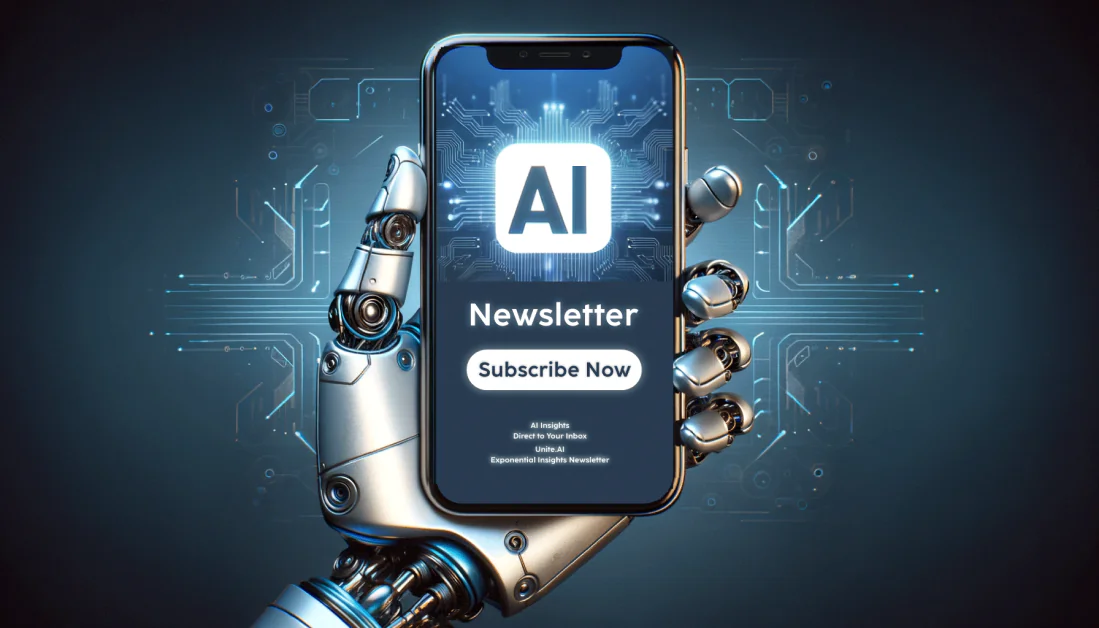