Thought Leaders
A Practical Guide to Making the Most of Your Investment in AI
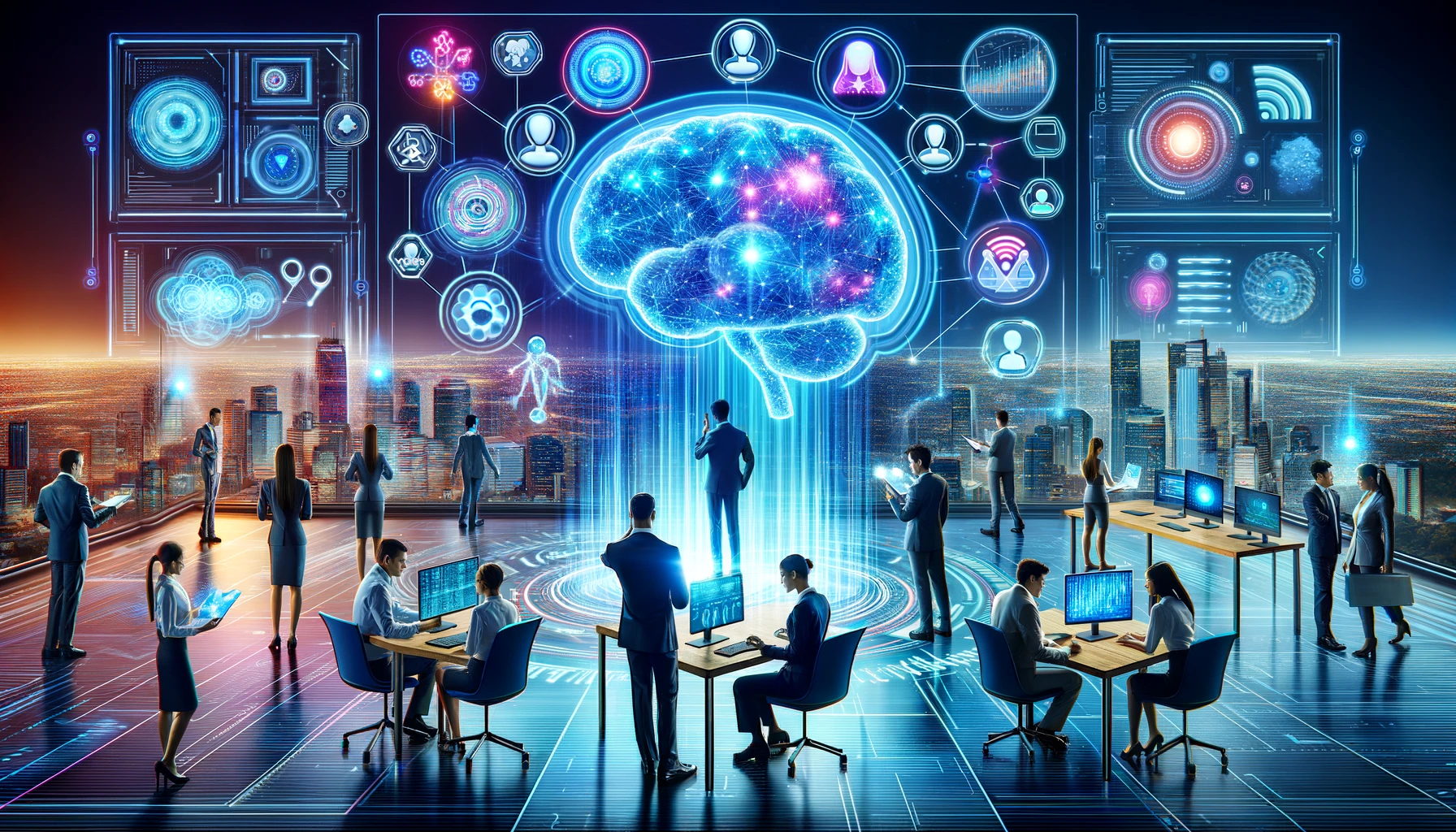
POV: You’ve heard a lot of noise about AI, and so you decide to do your own research. No matter where you turn, an expert expounds on AI's benefits and business-unlocking potential, and so you conclude that, yes, there is a business case to be made for an AI solution within your business.
Now what?
The pitch for AI solutions to be utilized in a myriad of different ways, from machine learning tools that bolster customer service to better personalization and product recommendation engines for customers to logistics and supply chain optimization tools, is a strong one. When integrated successfully, AI technology can have massive ROI, leading to better sales, more satisfied customers, and streamlined operations that save thousands of dollars each year. With all of this in mind, it’s no surprise that investment in AI is projected to top $200 billion by 2025.
In many cases, however, companies are investing in AI without having a clear roadmap to rolling it out. Investing in an AI solution without an outlined path for integration or implementation is a bit like buying a high-performance sports car without even knowing how to drive a stick shift.
Let’s take a look at a few of the steps businesses should take after investing in AI to ensure a successful implementation, including data considerations, training, best practices and how a successful rollout can improve customer experience overall.
The Essential Role of Data in AI Implementation
Looking at the applications for the current generation of AI and machine learning, they appear to have solved a very specific problem: companies are overwhelmed by data inputs that they can’t turn into actionable insights manually.
But the catch is that the efficacy of an AI engine is determined by the strength and utility of the data it has to build from. To maximize any AI investment, organizations need to optimize their data for quality, quantity and relevance.
A solid data foundation can be achieved in three phases.
The first phase is all about developing a data strategy based on the specific application for the AI system. During this phase, a brand will define which data will be collected, how it will be stored, and how it will be utilized to support AI initiatives.
Identifying key data sources means understanding the role a company expects its AI investment to play. For example, leveraging AI to create a more robust and effective product recommendation and personalization engine requires connecting user data from a CRM and sourcing product data from a Product Information Management (PIM) system. Taking stock of which data the company has available and identifying any blind spots can help build out data-gathering initiatives.
From there, a brand will need to set data governance rules and implement frameworks for data quality assurance, privacy compliance, and security. The brand will also want to assess data storage infrastructure and potentially invest in a scalable solution — implementing an AI engine can require large volumes of data.
With a solid data strategy in place, the next phase is data onboarding and initialization. Onboarding data into AI systems is a crucial step that requires careful planning and execution. The goal is to streamline data integration processes to enable AI models to learn effectively from the data.
But before data can be onboarded, it needs to be preprocessed to remove inconsistencies or conflicting and irrelevant information and formatted to ensure compatibility with AI algorithms. This process can be arduous, but with proper planning and a firm understanding of which relevant data will be imported, it should be manageable for even the smaller-sized teams.
What’s more, this initialization process only needs to be done once. With data preprocessed, the next step is automating data pipelines to supply the AI system with properly formatted, relevant data in a way that minimizes manual intervention. From there, the system simply needs to be monitored for quality and fitted with protocols to track data versions over time.
Lastly, investment in AI requires consistent upkeep and optimization on the data side. Through constant monitoring of the AI’s performance and eliciting customer feedback on their interactions with AI, companies should always be on the hunt for improvements within their AI implementation process and ongoing integration. When AI systems represent such a major investment — with major benefits to match — it’s only prudent to give it the best chance of success through best data practices.
AI Literacy is Necessary for Long-Term Project Success
If you’re in a managerial position, it can be easy to see AI through rose-colored glasses. Seeing the business potential can obscure the fact that there may be resistance among team members to accept new systems and new technologies, particularly ones that some workers see as a threat to their jobs. In fact, one Pew Research study showed that more than 80 percent of Americans feel mixed or negative emotions about the rise of AI.
Once a business has decided to invest in an AI solution, the first step is clearly defining the role AI will play and transparently communicating that role to employees. When employees understand AI’s potential and utility, it will remove friction points in training them to make the most of the technology.
Effective AI adoption also requires collaboration between disparate teams and disciplines. One way to encourage this collaboration is to form teams with diverse skill sets to tackle AI projects from multiple perspectives. Creating forums and leveraging existing communication channels to share AI insights, best practices, and success stories can build additional excitement around the initiative.
At the end of the day, however, making the most of your AI investment needs to be an organizational decision championed from the top down. Executive leadership needs to be on board with the project and communicate that enthusiasm throughout the team.
Making the Most of Your AI Investment
Despite what many sales pitches say, implementing an AI solution is not easy. It takes planning, organizational buy-in, and training. When executed successfully, however, it can have a transformative impact on user experience, organizational functionality, and much more.
On a practical level, making the most of your AI investment comes down to three key things. First and foremost, defining clear objectives will help the organization plan for implementation and understand what success looks like with AI. Next, remember that everything doesn’t have to be completed on day one. Taking an iterative approach to implementation can slow the process down and ensure that your team and the technology are working in lockstep.
Lastly, AI is not a cure-all, especially right out of the gate. True success with AI requires monitoring and evaluation, taking what works, replicating those successes, and optimizing them. AI is a long-term strategy whose value can be game-changing for a business. Approaching it in a smart and measured way can help truly unlock that major investment.
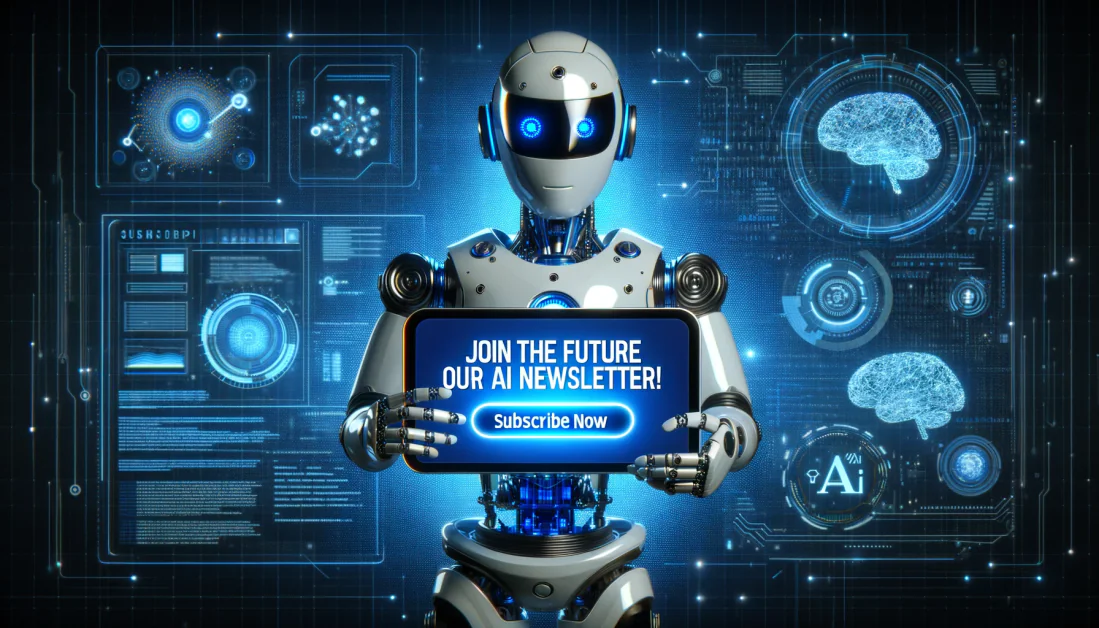
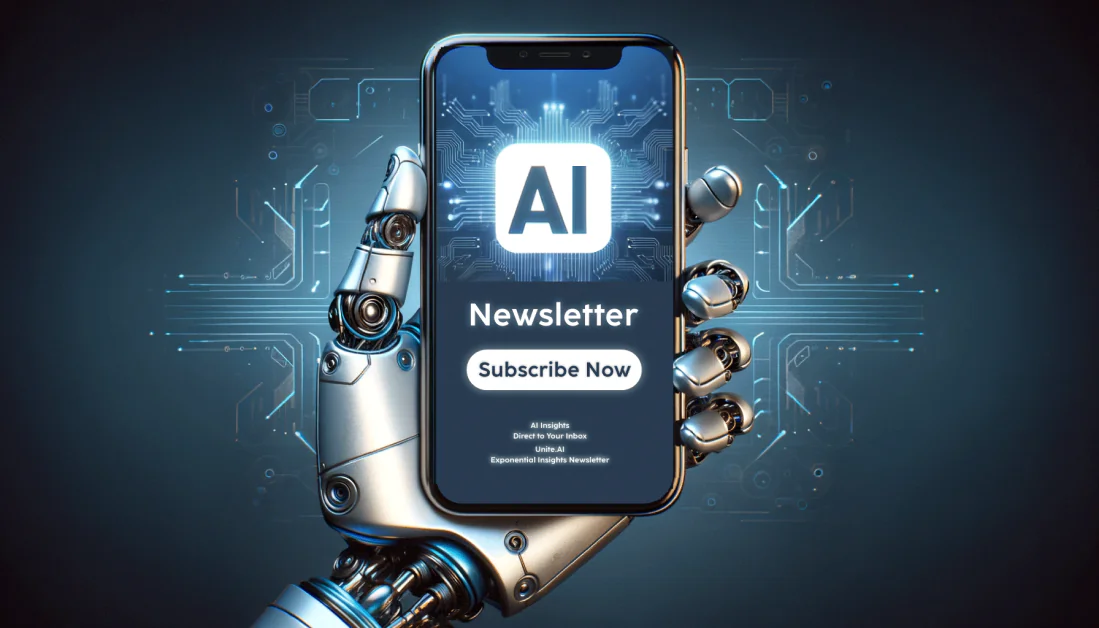