Artificial Intelligence
A New AI System Could Create More Hope For People With Epilepsy
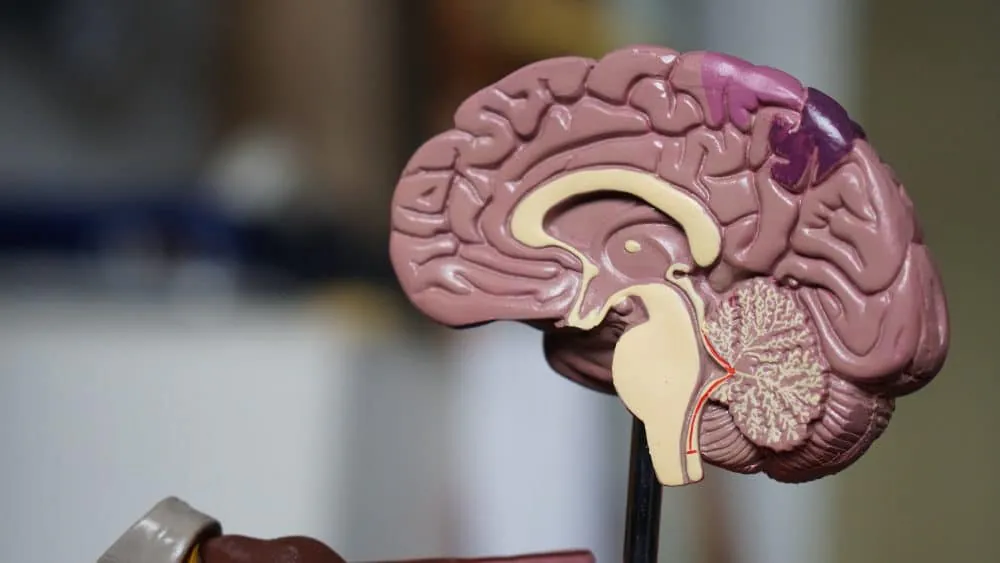
As Endgadget reports, two AI researchers may have created a system that creates new hope for people suffering from epilepsy – a system “that can predict epileptic seizures with 99.6-percent accuracy,” and do it up to an hour before seizures occur.
This would not be the first new advancement, since previously researchers at Technical University (TU) in Eindhoven, Netherlands developed a smart arm bracelet that can predict epileptic seizures during nighttime. But the accuracy and short time-frame the new AI system can work on as IEEE Spectrum notes, gives more hope to around 50 million people around the world who suffer from epilepsy (based on the data from World Health Organization). Out of this number of patients, 70 percent of them can control their seizures with medication if taken on time.
The new AI system was created by Hisham Daoud and Magdy Bayoumi of the University of Louisiana at Lafayette, and is lauded as “a major leap forward from existing prediction methods.” As Hisham Daoud, one of the two researchers that developed the system explains, “Due to unexpected seizure times, epilepsy has a strong psychological and social effect on patients.”
As is explained, “each person exhibits unique brain patterns, which makes it hard to accurately predict seizures.” So far, the previously existing models predicted seizures “ in a two-stage process, where the brain patterns must be extracted manually and then a classification system is applied,” which, as Daoud explains, added to the time needed to make a seizure prediction.
In their approach explained in a study published on 24 July in IEEE Transactions on Biomedical Circuits and Systems, “the features extraction and classification processes are combined into a single automated system, which enables earlier and more accurate seizure prediction.”
To further boost the accuracy of their system Daoud and Bayoumi “incorporated another classification approach whereby a deep learning algorithm extracts and analyzes the spatial-temporal features of the patient’s brain activity from different electrode locations, boosting the accuracy of their model.” Since “EEG readings can involve multiple ‘channels’ of electrical activity,” to speed up the prediction process, even more, the two researchers “applied an additional algorithm to identify the most appropriate predictive channels of electrical activity.”
The complete system was then tested on 22 patients at the Boston Children’s Hospital. While the sample size was small, the system proved to be very accurate (99.6%), and had “a low tendency for false positives, at 0.004 false alarms per hour.”
As Daoud explained the next step would be the development of a customized computer chip to process the algorithms. “We are currently working on the design of efficient hardware [device] that deploys this algorithm, considering many issues like system size, power consumption, and latency to be suitable for practical application in a comfortable way to the patient.”
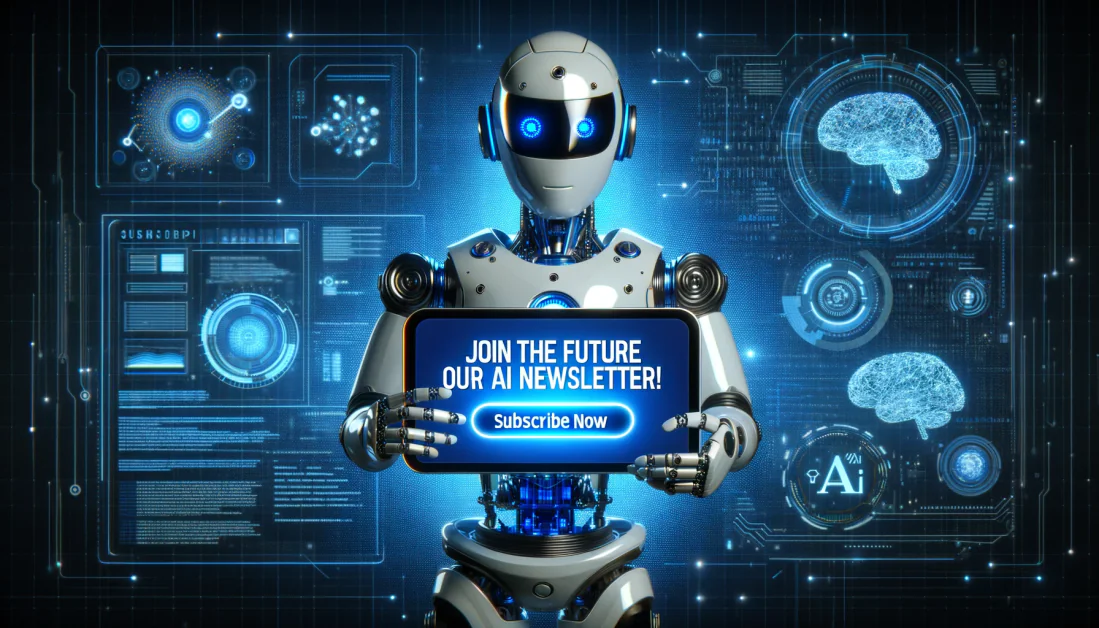
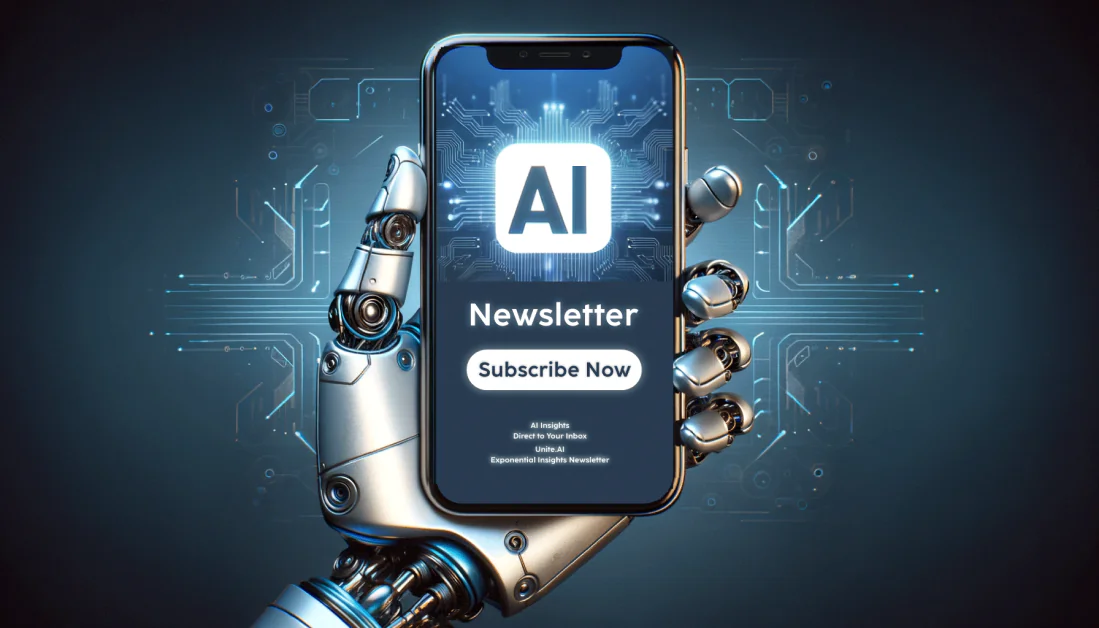