Artificial Intelligence
The Shortcomings of Amazon Mechanical Turk May Threaten Natural Language Generation Systems
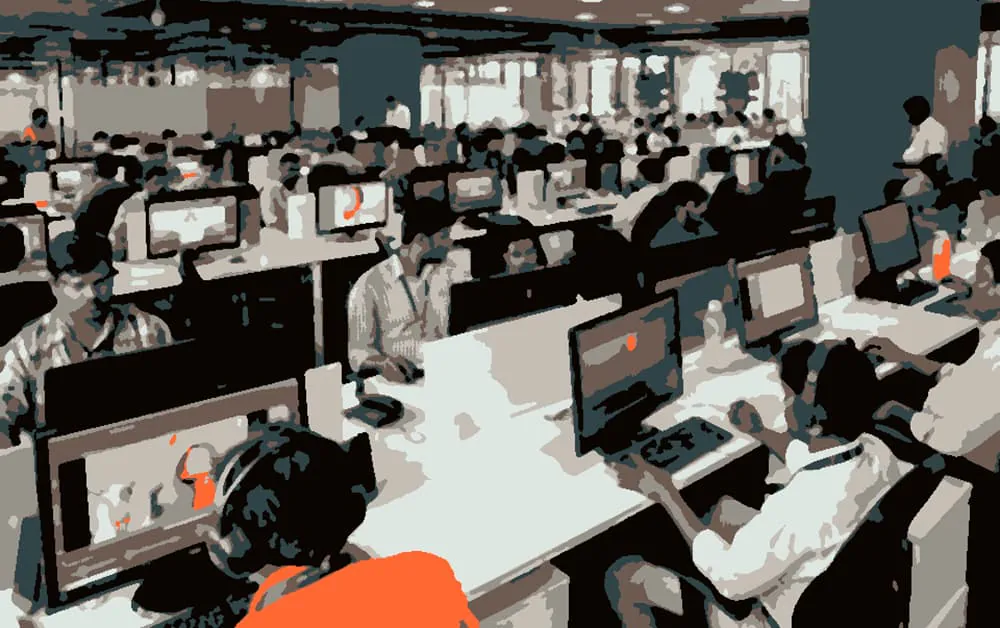
A new study from the University of Massachusetts Amherst has pitted English teachers against crowdsourced workers at Amazon Mechanical Turk in assessing the output of Natural Language Generation (NLG) systems, concluding that lax standards and the ‘gaming' of prized tasks among AMT workers could be hindering the development of the sector.
The report comes to a number of damning conclusions regarding the extent to which the ‘industrial-scale' cheap outsourcing of open-ended NLG evaluation tasks could lead to inferior results and algorithms in this sector.
The researchers also compiled a list of 45 papers on open-ended text generation where the research had made use of AMT, and found that ‘the vast majority' failed to report critical details about the use of Amazon's crowd service, making it difficult to reproduce the papers' findings.
Sweat-Shop Labor
The report levels criticism at both the sweat-shop nature of Amazon Mechanical Turk, and the (likely budget-constrained) academic projects that are lending AMT additional credence by using (and citing) it as a valid and consistent research resource. The authors note:
‘While AMT is a convenient and affordable solution, we observe that high variance between workers, poor calibration, and cognitively-demanding tasks can lead researchers to draw misleading scientific conclusions (e.g., that human-written text is “worse” than GPT-2’s).'
The report blames the game rather than the players, with the researchers observing:
‘[Crowd] workers are frequently underpaid for their labor, which harms both the quality of the research, and more importantly, the ability of these crowd workers to earn an adequate living.'
The paper, titled The Perils of Using Mechanical Turk to Evaluate Open-Ended Text Generation, further concludes that ‘expert raters' such as language teachers and linguists should be used to evaluate open-ended artificial NLG content, even if AMT is cheaper.
Test Tasks
In comparing AMT's performance against less time-constrained, expert readers, the researchers spent $144 on the AMT services actually used in the comparison tests (though much more was spent on ‘non-usable' results – see below), requiring random ‘Turks' to evaluate one of 200 texts, split between human-created text content and artificially generated text.
Tasking professional teachers with the same work cost $187.50, and confirming their superior performance (compared to AMT workers) by hiring Upwork freelancers to replicate the tasks cost an additional $262.50.
Each task consisted of four evaluative criteria: grammar (‘How grammatically correct is the text of the story fragment?'); coherence (‘How well do the sentences in the story fragment fit together?'); likability (‘How enjoyable do you find the story fragment?'); and relevance (‘How relevant is the story fragment to the prompt?').
Generating the Texts
To obtain NLG material for the tests, the researchers used Facebook AI Research's 2018 Hierarchical Neural Story Generation dataset, which comprises 303,358 English language stories composed by users at the very popular (15m+ users) r/writingprompts subreddit, where subscribers' stories are ‘seeded' by single-sentence ‘prompts' in a similar way to current practices in text-to-image generation – and, of course, in open-ended Natural Language Generation systems.
200 prompts from the dataset were randomly selected and passed through a medium-sized GPT-2 model using the Hugging-Face Transformers library. Thus two sets of results were obtained from the same prompts: the human-written discursive essays from Reddit users, and GPT-2-generated texts.
In order to prevent the same AMT workers judging the same story multiple times, three AMT worker judgements were solicited per example. Together with experiments regarding the English language capabilities of the workers (see end of article) and discounting results from low-effort workers (see ‘Short Time' below), this increased the total expenditure on AMT to around $1,500 USD.
To create a level playing field, all tests were conducted week days between 11.00am-11:30am PST.
Results and Conclusions
The sprawling study covers a lot of ground, but the key points are as follows:
Short Time
The paper found that an official Amazon-reported average task time of 360 seconds boiled down to a real-world working time of just 22 seconds, and a median working time of only 13 seconds – a quarter of the time taken by the fastest English teacher replicating the task.
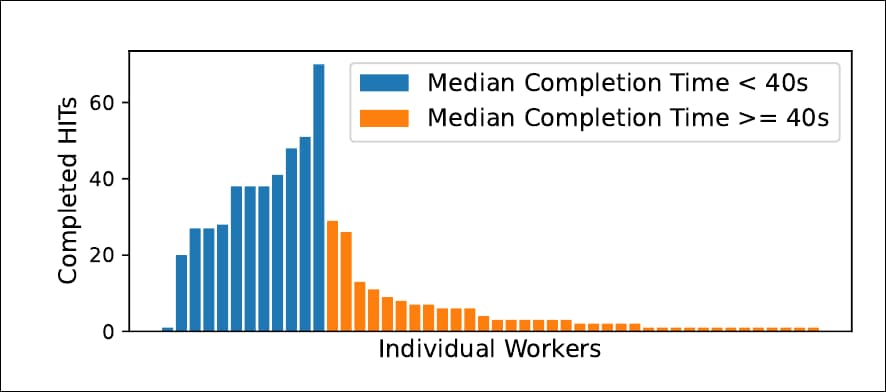
From Day 2 of the study: the individual workers (in orange) spent notably less time evaluating each task than the better-paid teachers, and (later) the even better-paid Upwork contractors. Source: https://arxiv.org/pdf/2109.06835.pdf
Since AMT imposes no limit on the Human Intelligence Tasks (HITs) that an individual worker can take on, AMT ‘big hitters' have emerged, with (profitable) reputations for completing high numbers of tasks per experiment. In order to compensate for accepted hits by the same worker, the researchers measured the time between consecutively submitted HITs, comparing the start and end time of each HIT. In this way, the shortfall between AMT's reported WorkTimeInSeconds and the actual time spent on the task came into focus.
Since such work cannot be accomplished in these reduced time-frames, the researchers had to compensate for this:
‘As it is impossible to carefully read a paragraph-length story and assess all four properties in as little as 13 seconds, we measure the impact on average ratings when filtering out workers who spend too little time per HIT…Specifically, we remove judgments from workers whose median time is below 40s (which is a low bar), and find that on average about 42% of our ratings are filtered out (ranging from 20%-72% across all experiments).'
The paper contends that misreported actual work time in AMT is ‘a major issue' typically overlooked by researchers using the services.
Hand-Holding Necessary
The findings further suggest that AMT workers can't reliably distinguish between text written by a human and text written by a machine, unless they see both texts side by side, which would effectively compromise a typical evaluation scenario (where the reader should be able to make a judgement based on a single sample of text, ‘real' or artificially generated).
Casual Acceptance of Low-Quality Artificial Text
AMT workers consistently rated low-quality GPT-based artificial text on a par with higher quality, coherent text written by humans, in contrast to the English teachers, who were easily able to distinguish the difference in quality.
No Prep Time, Zero Context
Entering the correct mind-set for such an abstract task as evaluation of authenticity does not come naturally; English teachers required 20 tasks in order to calibrate their sensibilities to the evaluative environment, while AMT workers typically get no ‘orientation time' at all, lowering the quality of their input.
Gaming The System
The report maintains that the total time AMT workers spend on individual tasks are inflated by workers that accept multiple tasks simultaneously, and run through the tasks in different tabs on their browsers, instead of concentrating on one task for the recorded task duration.
Country of Origin is Important
The default settings of AMT does not filter workers by country of origin, and the report notes prior work indicating that AMT workers use VPNs to work around geographical restrictions, enabling non-native speakers to present as native English speakers (in a system that, perhaps rather naively, equates a worker's mother tongue with their IP-based geographical location).
Thus the researchers re-ran the evaluation tests on AMT with filters limiting potential takers to non-English speaking countries, finding that ‘workers from non-English speaking countries rated coherence, relevance, and grammar…significantly lower than identically-qualified workers from English-speaking countries'.
The report concludes:
‘[Expert] raters such as linguists or language teachers should be used whenever possible as they have already been trained to evaluate written text, and it is not much more expensive…'.
Published 16th September 2021 – Updated 18th December 2021: Added tags
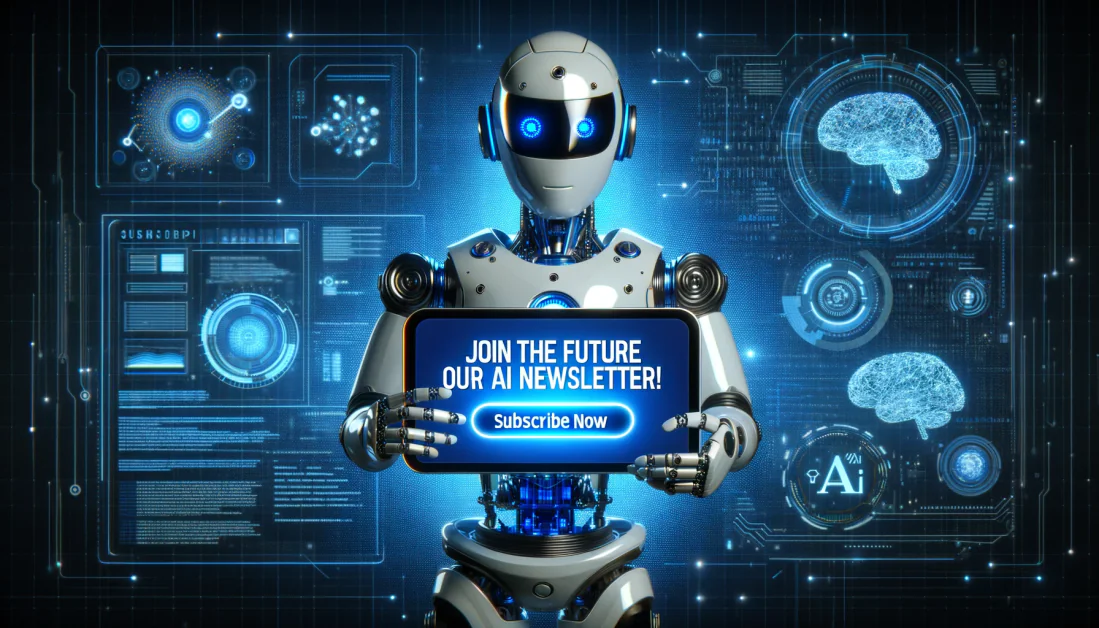
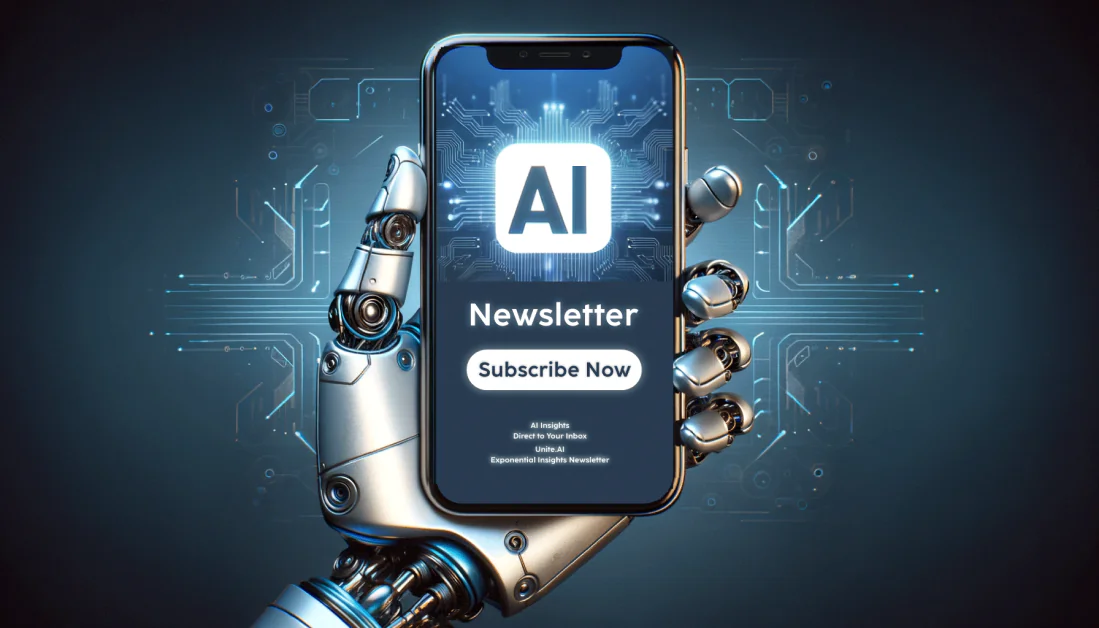