Ethics
The AI Incident Database Aims to Make AI Algorithms Safer
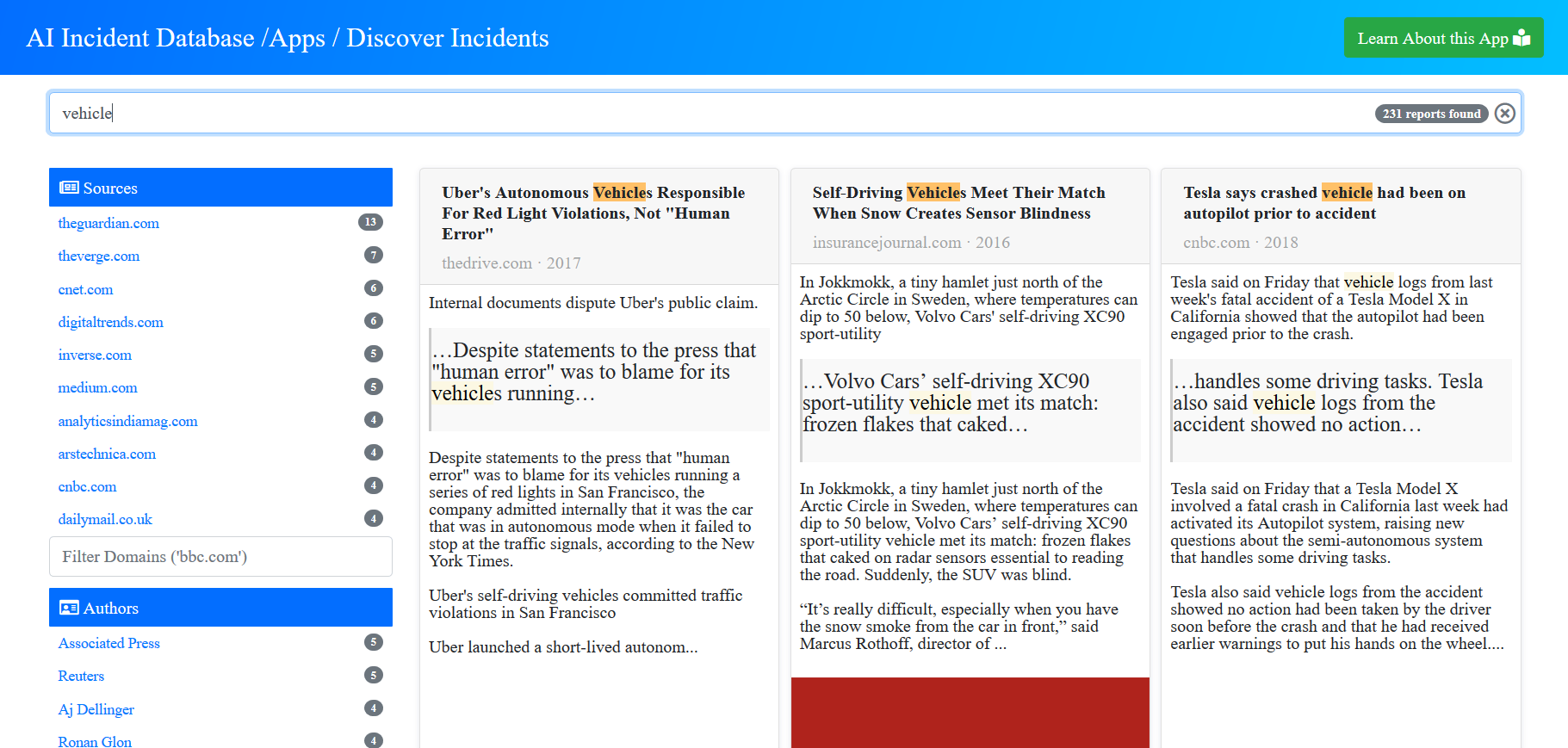
Any sufficiently large system will have errors, and part of correcting for these errors is having a database of them that can be analyzed for impacts and potential causes. Much like the FDA maintains a database for adverse medication reactions, or the National Transportation Safety Board maintains a database for aviation accidents, the AI Incident Database is a database intended to catalog failures of AI systems and to help AI researchers engineer new methods of avoiding these failures. The creators of the AI Incident Database (AIID) hope that it will assist AI companies in developing safer, more ethical AI.
What is the AIID?
The AIID is the product of the Partnership on AI (PAI) organization. PAI was initially founded in 2016 by members of AI research teams at large tech companies like Facebook, Apple, Amazon, Google, IBM, and Microsoft. Since then, the organization has recruited members from many more organizations, including various nonprofits. In 2018, PAI set out to create a consistent classification standard for AI failures. However, there was no collection of AI incidents on which to base this classification. For this reason, PAI created the AIID.
According to TechTalks, the format of AIID was informed by the structure of the aviation accidents database maintained by the National Transportation Safety Board. Since reports started being collected in 1996, the commercial air travel system has managed to increase the safety of the aviation industry by archiving and analyzing incidents. The hope is that a similar repository of AI incidents can make AI systems safer, more ethical, and more reliable. AIID also took inspiration from the Common Vulnerabilities and Exposures database, which is a repository of notable software failures that span a variety of different industries and disciplines.
Sean McGregor is the lead technical consultant at IBM for the Watson AI XPRIZE. McGregor is also responsible for overseeing the development of AIID’s actual database. McGregor explained that the goal of AIID is ultimately to prevent AI systems from causing har, or at least decrease the severity of adverse incidents. As McGregor noted, machine learning systems are substantially more complex and unpredictable than traditional software systems, and as a result, they can’t be tested in the same way that other software can. Machine learning systems can change their behavior in unexpected ways. McGregor notes that the capacity of deep learning systems to learn can mean “malfunctions are more likely, more complicated, and more dangerous” when they enter into an unstructured world.
More than 1,000 AI-related Incidents Logged
Since AIID was initially created, there have been more than 1,000 AI-related incidents logged into the database. Out of all the incidents in the database, issues involving AI fairness were the most common type of adverse incident. Many of these fairness incidents involve the use of facial recognition algorithms by government agencies. McGregor also notes that there is an increasing number of incidents involving robotics being added to the database.
Visitors to the database can execute queries, searching the database for incidents based on criteria like keywords, incident ID, source, or author. For example, running a search for “Deepfake” returns 7 reports, while searching for “robots” returns 158 reports.
A centralized database for incidents of AI’s malfunctioning and operating in unexpected ways can help researchers, engineers, and ethicists oversee the development and deployment of AI systems. Product managers at tech companies could use the AIID to see if there are any potential issues before employing AI recommendation systems, or an AI engineer could get an idea of potential biases that need to be corrected when creating the AI application. Likewise, risk officers could use the database to determine potential negative side-effects associated with an AI model, enabling them to plan ahead and develop measures to mitigate potential harms.
The architecture underlying AIID is meant to be flexible, as this enables the creation of new tools to query the database and extract meaningful insights. The Partnership on AI and McGregor will be collaborating to engineer a flexible taxonomy that can be used to classify all different forms of AI incidents. The team hopes that the once the flexible taxonomy has been created, it can be combined with an automated system that will automatically log AI incidents.
“The AI community has begun sharing incident records with each other to motivate changes to their products, control procedures, and research programs,” McGregor exlpained via TechTalks. “The site was publicly released in November, so we are just starting to realize the benefits of the system.”
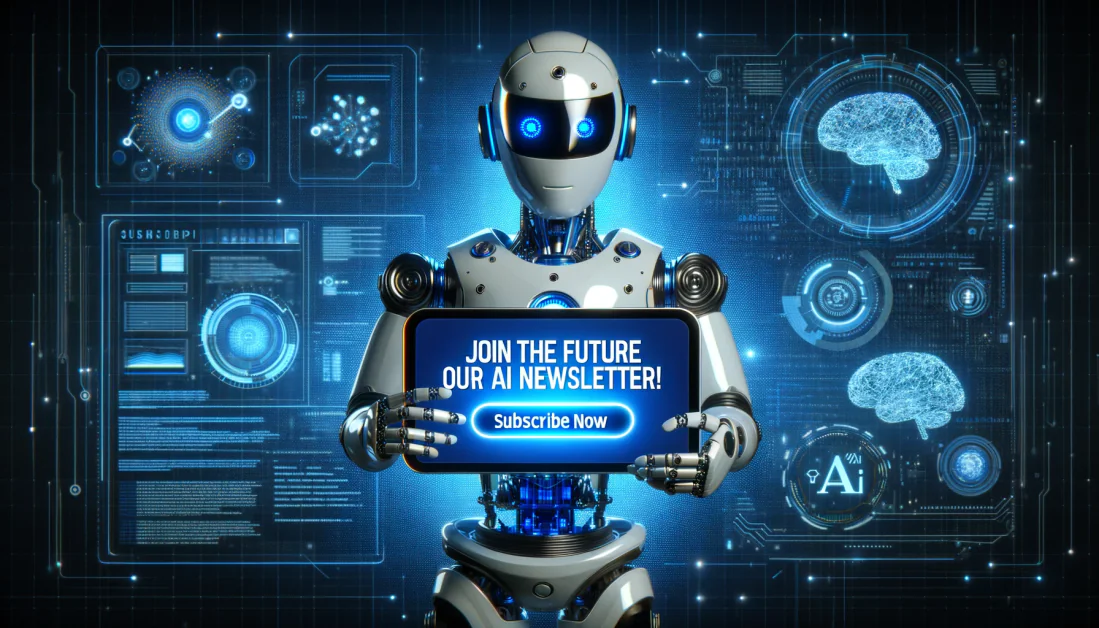
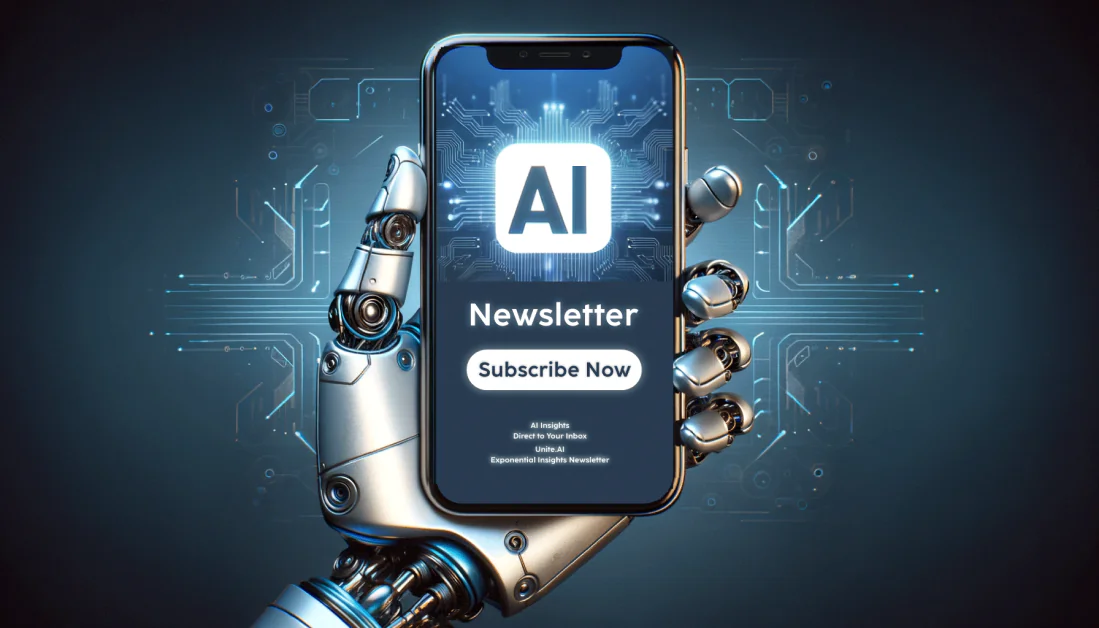