Artificial Intelligence
Researchers Use Memristors To Create More Energy Efficient Neural Networks
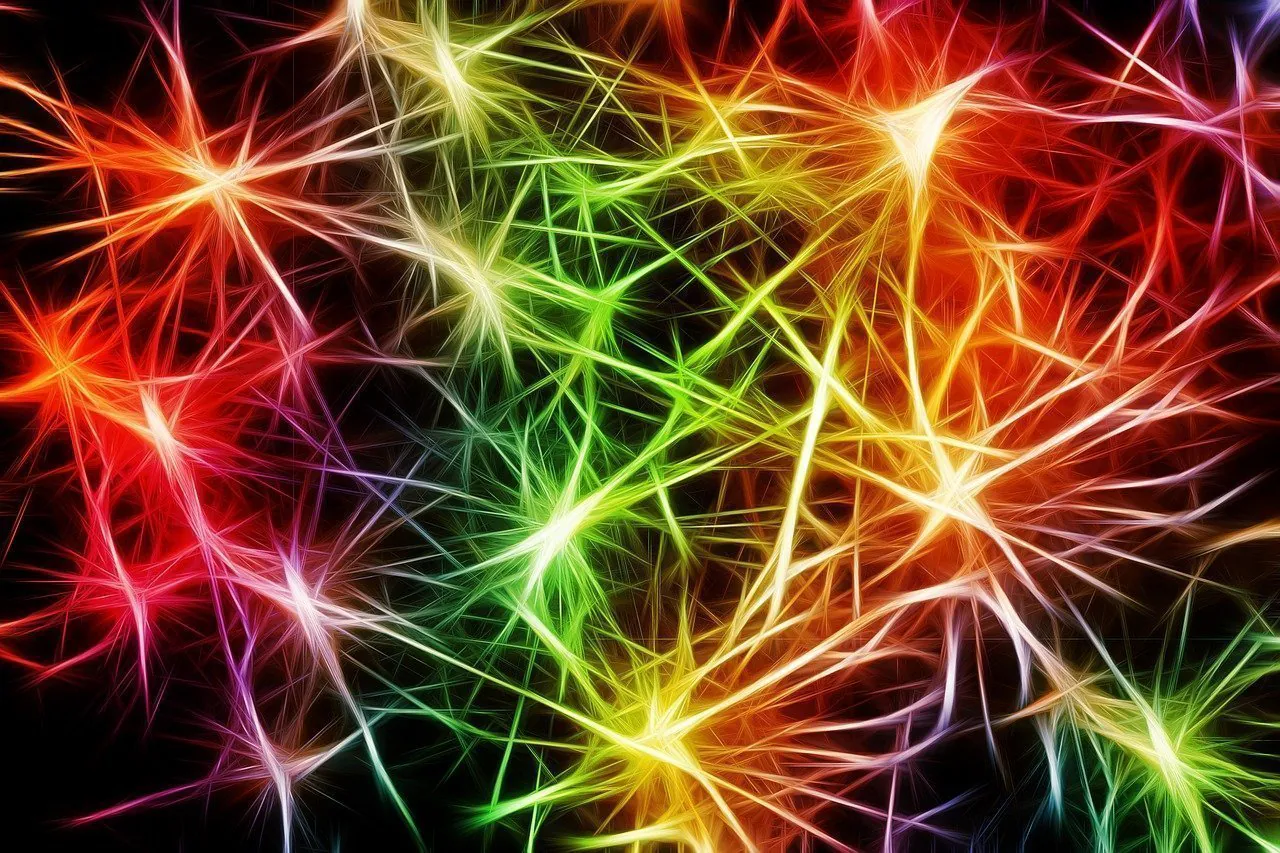
One of the less glamorous aspects of artificial intelligence is that it often requires a large amount of processing power and therefore it often has a large energy footprint. Recent work done by researchers at UCL has determined a method of improving an AIās energy efficiency.
Neural networks and machine learning are powerful tools, but the most impressive feats of artificial intelligence usually have a large energy cost associated with them. For example, when OpenAI taught a robotic hand to manipulate a Rubikās cube, it was estimated that the feat required around 2.8 gigawatt-hours of electricity.
According to TechExplore, Researchers at UCL have designed a new method of generating artificial neural networks. The new method utilizes memristors to generate the network, which are around 1000 times more energy-efficient than networks created with traditional approaches. Memristors are devices that can recall the amount of electrical charge that last flowed through them, preserving that memory state after they have been shut off. This means that they can remember their state even if a device should lose power. Although memristors were first theorized about around 50 years ago, it wasnāt until 2008 that a real memristor was created.
Memristors are occasionally referred to as āneuromorphicā computing devices or ābrain-inspiredā devices. Memristors are similar to the building blocks the brain uses to process information and create memories. They are highly efficient compared to most modern computer systems. These memristor devices possess aspects of capacitors and resistors, and over the past decade or so they have been manufactured and used in a variety of memory devices. The UCL research teams hope that their research will help these devices be used to create AI systems within a few years.
Despite their increased energy efficiency, memristors are traditionally much less efficient than regular neural networks, but the UCL researchers found a way to increase the accuracy of memristors. The researchers found that when using many memristors, they could be split up into multiple sub-groups and then their calculations averaged together. The averaging of the calculations help flaws in the subgroups cancel each other out and the more relevant patterns found.
Dr. Adnan Mehonic and Ph.D. student Dovydas Joksas (both UCL Electronic and Electrical Engineering) and their co-authors tested this averaging approach across various memristor types and found that the technique seemed to improve accuracy in all of the different memristors tested, not just one or two of them. The accuracy improvements applied to all the groups that were tested, no matter the type of material the memristor was made out of.
According to Dr. Mehonic, as quoted by TechExplore:
“We hoped that there might be more generic approaches that improve not the device-level, but the system-level behavior, and we believe we found one. Our approach shows that, when it comes to memristors, several heads are better than one. Arranging the neural network into several smaller networks rather than one big network led to greater accuracy overall.”
The research team was excited to have taken a computer science technique and applied to memristors, also using a common error avoidance technique (averaging calculations) to increase the accuracy of memristive neural networks. Study co-author Professor Tony Kenyon of UCL Electronic & Electrical Engineering believes that memristors could “take a leading role” in creating more energy-sustainable edge computing devices and IoT devices.
Memristors are not only more energy-efficient than traditional neural network models, they can be easily included in a hand-held, mobile device. This is predicted to be of increasing importance in the near future as more data is created and transmitted all the time even though it is difficult to increase transmission capacity beyond a certain point. Memristors could help enable the transfer of large volumes of data at a fraction of the energy cost.