Artificial Intelligence
Researchers Look To Animals To Give Reinforcement Learning Systems Common Sense
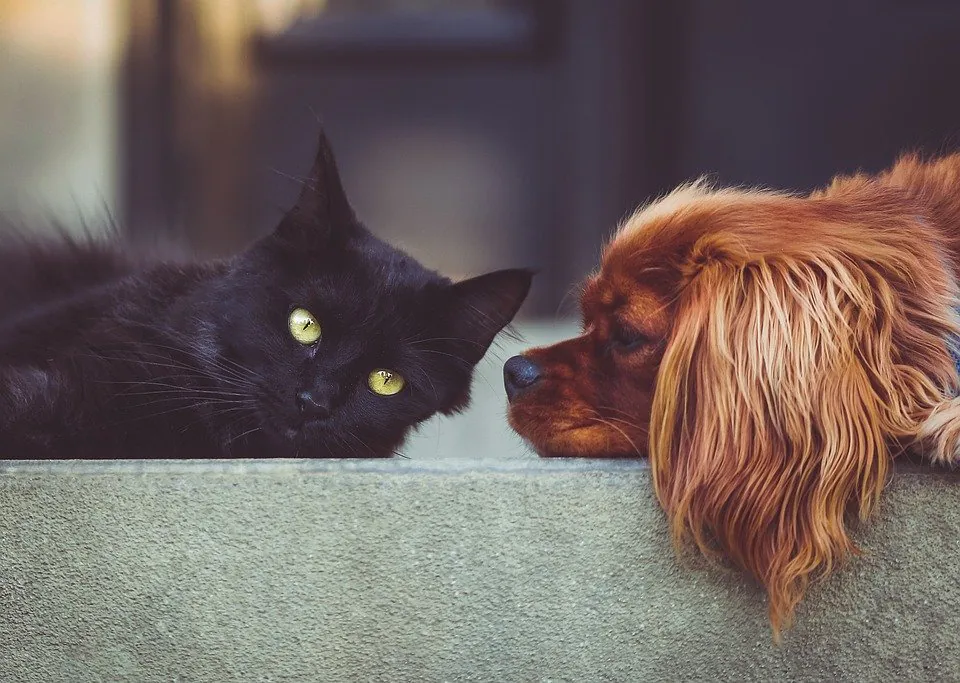
AI researchers from institutes like Imperial College London, University of Cambridge, and Google DeepMind are looking to animals for inspiration on how to improve the performance of reinforcement learning systems. In a joint paper published in CellPress Reviews, entitled āArtificial Intelligence and the Common Sense of Animalsā, the researchers argue that animal cognition provides useful benchmarks and methods of evaluation for reinforcement learning agents and it can also inform the engineering of tasks and environments.
AI researchers and engineers have long looked to biological neural networks for inspiration when designing algorithms, using principals from behavioral science and neuroscience to inform the structure of algorithms. Yet most of the cues AI researchers take from the neuroscience/behavior science fields are based on humans, with the cognition of young children and infants serving as the focal point. AI researchers have yet to take much inspiration from animal models, but animal cognition is an untapped resource that has the potential to lead to important breakthroughs in the reinforcement learning space.
Deep reinforcement learning systems are trained through a process of trial and error, reinforced with rewards whenever a reinforcement learning agent gets closer to completing a desired objective. This is very similar to teaching an animal to carry out a desired task by using food as a reward. Biologists and animal cognition specialists have carried out many experiments assessing the cognitive abilities of a variety of different animals, including dogs, bears, squirrels, pigs, crows, dolphins, cats, mice, elephants, and octopuses. Many animals exhibit impressive displays of intelligence, and some animals like elephants and dolphins may even have a theory of mind.
Looking at the body of research done regarding animal cognition might inspire AI researchers to consider problems from different angles. As deep reinforcement learning has become more powerful and sophisticated, AI researchers specializing in the field are seeking out new ways of testing the cognitive capabilities of reinforcement learning agents. In the research paper, the research team makes reference to the types of experiments carried out with primates and birds, mentioning that they aim to design systems capable of accomplishing similar types of tasks, giving an AI a type of ācommon senseā. According to the authors of the paper, they āadvocate an approach wherein RL agents, perhaps with as-yet-undeveloped architectures, acquire what is needed through extended interaction with rich virtual environments.ā
As reported by VentureBeat, the AI researchers argue that common sense isnāt a trait unique to humans and that it is dependent upon an understanding of basic properties of the physical world, such as how an object occupies a point and space, what constraints there are on that objectās movements, and an appreciation for cause and effect. Animals display these traits in laboratory studies. For instance, crows understand that objects are permanent things, as they are able to retrieve seeds even when the seed is hidden from them, covered up by another object.
In order to endow a reinforcement learning system with these properties, the researchers argue that they will need to create tasks that, when paired with the right architecture, will create agents capable of transferring learned principles to other tasks. The researchers argue that training for such a model should involve techniques that require an agent to gain understanding of a concept after being exposed to only a few examples, called few-shot training. This is in contrast to the traditional hundreds or thousands of trials that typically goes into the trial and error training of an RL agent.
The research team goes on to explain that while some modern RL agents can learn to solve multiple tasks, some of which require the basic transfer of learned principles, it isnāt clear that RL agents could learn a concept as abstract at ācommon senseā. If there was an agent potentially capable of learning such a concept, they would need tests capable of ascertaining if an RL agent understood the concept of a container.
DeepMind in particular is excited to engage with new and different ways of developing and testing reinforcement learning agents. Recently, at the Stanford HAI conference that took place earlier in October, DeepMindās head of neuroscience research, Matthew Botvinick, urged machine learning researchers and engineers to collaborate more in other fields of science. Botvinick highlighted the importance of interdisciplinary work with psychologists and neuroscience for the AI field in a talk called āTriangulating Intelligence: Melding Neuroscience, Psychology, and AIā.