Healthcare
Recognizing Employee Stress Through Facial Analysis at Work
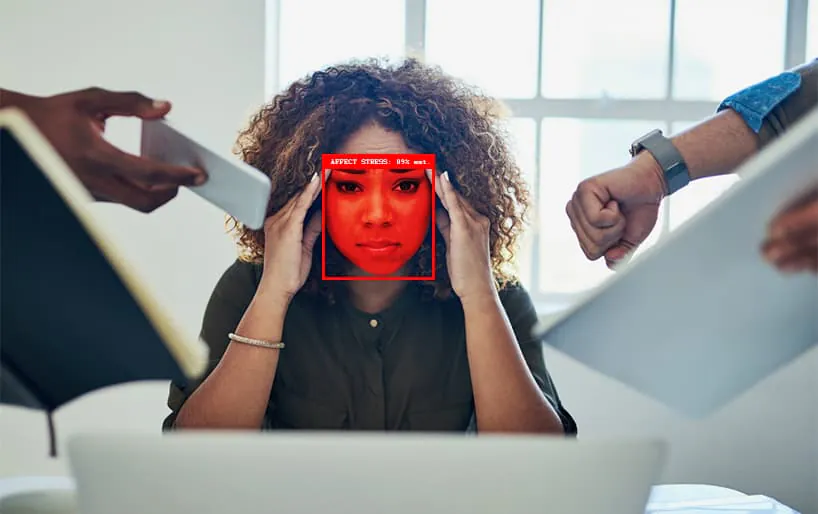
In the context of the changing culture around Zoom-meeting etiquette, and the emergence of Zoom fatigue, researchers from Cambridge have released a study that uses machine learning to determine our stress levels via AI-enabled webcam coverage of our facial expressions at work.
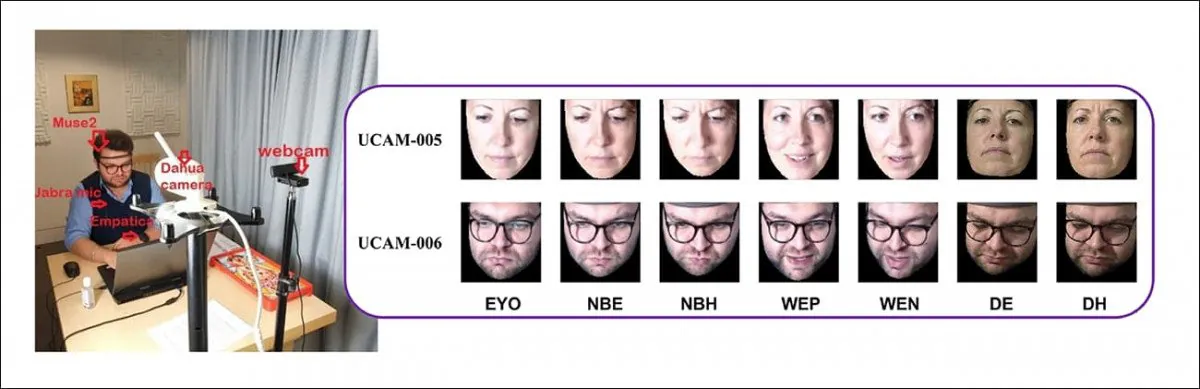
On the left, the data-gathering environment, with multiple monitoring equipment either trained on or attached to a volunteer; on the right, example facial expressions generated by test subjects at varying levels of task difficulty. Source: https://arxiv.org/pdf/2111.11862.pdf
The research is intended for affect analysis (i.e., emotion recognition) in ‘Ambient Assistive Living' systems, and presumably is designed to enable video-based AI facial expression monitoring frameworks in such systems; though the paper does not expand on this aspect, the research effort makes no sense in any other context.
The specific ambit of the project is to learn facial expression patterns in working environments – including remote working arrangements – rather than ‘leisure' or ‘passive' situations, such as traveling.
Face-Based Emotion Recognition in the Workplace
While ‘Ambient Assistive Living' may sound like a scheme for elder care, that's far from the case. Speaking of the intended ‘end users', the authors state*:
‘Systems created for ambient assistive living environments [†] aim to be able to perform both automatic affect analysis and responding. Ambient assistive living relies on the usage of information and communication technology (ICT) to aid in person’s every day living and working environment to keep them healthier and active longer, and enable them to live independently as they age. Thus, ambient assistive living aims to facilitate health workers, nurses, doctors, factory workers, drivers, pilots, teachers as well as various industries via sensing, assessment and intervention.
‘The system is intended to determine the physical, emotional and mental strain and respond and adapt as and when needed, for instance, a car equipped with a drowsiness detection system can inform the driver to be attentive and can suggest them to take a little break to avoid accidents [††].'
The paper is titled Inferring User Facial Affect in Work-like Settings, and comes from three researchers at the Affective Intelligence & Robotics Lab at Cambridge.
Test Conditions
Since prior work in this field has depended largely on ad hoc collections of images scraped from the internet, the Cambridge researchers conducted local data-gathering experiments with 12 campus volunteers, 5 male and 7 female. The volunteers came from nine countries, and were aged 22-41.
The project aimed to recreate three potentially stressful working environments: an office; a factory production line; and a teleconference call – such as the kind of Zoom group chat that has become a frequent feature of homeworking since the advent of the pandemic.
Subjects were monitored by various means, including three cameras, a Jabra neck-worn microphone, an Empatica wristband (a wireless multi-sensor wearable offering real-time biofeedback), and a Muse 2 headband sensor (which also offers biofeedback). Additionally, the volunteers were asked to complete surveys and self-evaluate their mood periodically.
However, this does not mean that future Ambient Assistive Living rigs are going to ‘plug you in' to that extent (if only for cost reasons); all of the non-camera monitoring equipment and methods used in the data-gathering, including the written self-assessments, are intended to verify the face-based affect recognition systems that are enabled by camera footage.
Ramping up the Pressure: The Office Scenario
In the first two of the three scenarios (‘Office' and ‘Factory'), the volunteers were started off at an easy pace, with the pressure gradually increasing over four phases, with different types of task for each.
At the highest level of induced stress, the volunteers also had to endure the ‘white coat effect' of someone looking over their shoulder, plus 85db of additional noise, which is just five decibels below the legal limit for an office environment in the US, and the exact maximum limit specified by the National Institute for Occupational Safety and Health (NIOSH).
In the office-like data-gathering phase, the subjects were tasked with remembering previous letters that had flashed across their screen, with increasing levels of difficulty (such as having to remember two-letter sequences that occurred two screens ago).
The Factory Scenario
To simulate a manual labor environment, the subjects were asked to play the game Operation, which challenges user dexterity by requiring the player to extract small objects from a board through narrow, metal-rimmed apertures without touching the sides, which event triggers a ‘failure' buzzer.
By the time the toughest phase came round, the volunteer was challenged to extract all 12 items without error inside one minute. For context, the world record for this task, set in the UK in 2019, stands at 12.68 seconds.
The Teleconferencing Scenario
Finally, in the homeworking/teleconference test, the volunteers were asked by an experimenter over an MS Teams call to recall their own positive and negative memories. For the most stressful phase of this scenario, the volunteer was required to recall a very negative or sad memory from their recent past.
The various tasks and scenarios were executed in random order, and compiled into a custom dataset titled Working-Environment-Context-Aware Dataset (WECARE-DB).
Method and Training
The results of the users' self-assessments of their mood were used as ground truth, and mapped to valence and arousal dimensions. The captured video of the experiments were run through a facial landmark detection network, and the aligned images fed to a ResNet-18 network trained on the AffectNet dataset.
450,000 images from AffectNet, all drawn/labeled from the internet using emotion-related queries, were manually annotated, the paper says, with valence and arousal dimensions.
Next, the researchers refined the network based solely on their own WECARE dataset, while spectral representation encoding was used to summarize frame-based predictions.
Results
The model's performance was evaluated on three metrics commonly associated with automated affect prediction: Concordance Coefficient Correlation; Pearson Coefficient Correlation; and Root Mean Square Error (RMSE).
The authors note that the model fine-tuned on their own WECARE dataset outperformed ResNet-18, and deduce from this that the way we govern our facial expressions is very different in a work environment than in the more abstract contexts from which prior studies have derived source material from the internet.
They state:
‘Looking at the table we observe that the model fine-tuned on WECARE-DB outperformed the ResNet-18 model pre-trained on [AffectNet], indicating that the facial behaviours displayed in work-like environments are different compared to the in-the-wild Internet settings utilised in the AffectNet DB. Thus, it is necessary to acquire datasets and train models for recognising facial affect in work-like settings.'
As regards the future of in-work affect recognition, enabled by networks of cameras trained at employees, and constantly making predictions of their emotional states, the authors conclude*:
‘The ultimate goal is to implement and use the trained models in real time and in real work settings to provide input to decision support systems to promote health and well-being of people during their working age in the context of the EU Working Age Project.'
* My emphasis.
† Here the authors make three citations:
Automatic, dimensional and Continuous Emotion recognition – https://ibug.doc.ic.ac.uk/media/uploads/documents/GunesPantic_IJSE_2010_camera.pdf
Exploring the ambient assisted living domain: a systematic review – https://link.springer.com/article/10.1007/s12652-016-0374-3
A Review of Internet of Things Technologies for Ambient Assisted Living Environments – https://mdpi-res.com/d_attachment/futureinternet/futureinternet-11-00259/article_deploy/futureinternet-11-00259-v2.pdf
†† Here the authors make two citations:
Real-time Driver Drowsiness Detection for Embedded System Using Model Compression of Deep Neural Networks – https://openaccess.thecvf.com/content_cvpr_2017_workshops/w4/papers/Reddy_Real-Time_Driver_Drowsiness_CVPR_2017_paper.pdf
Real-Time Driver-Drowsiness Detection System Using Facial Features – https://www.semanticscholar.org/paper/Real-Time-Driver-Drowsiness-Detection-System-Using-Deng-Wu/1f4b0094c9e70bf7aa287234e0fdb4c764a5c532
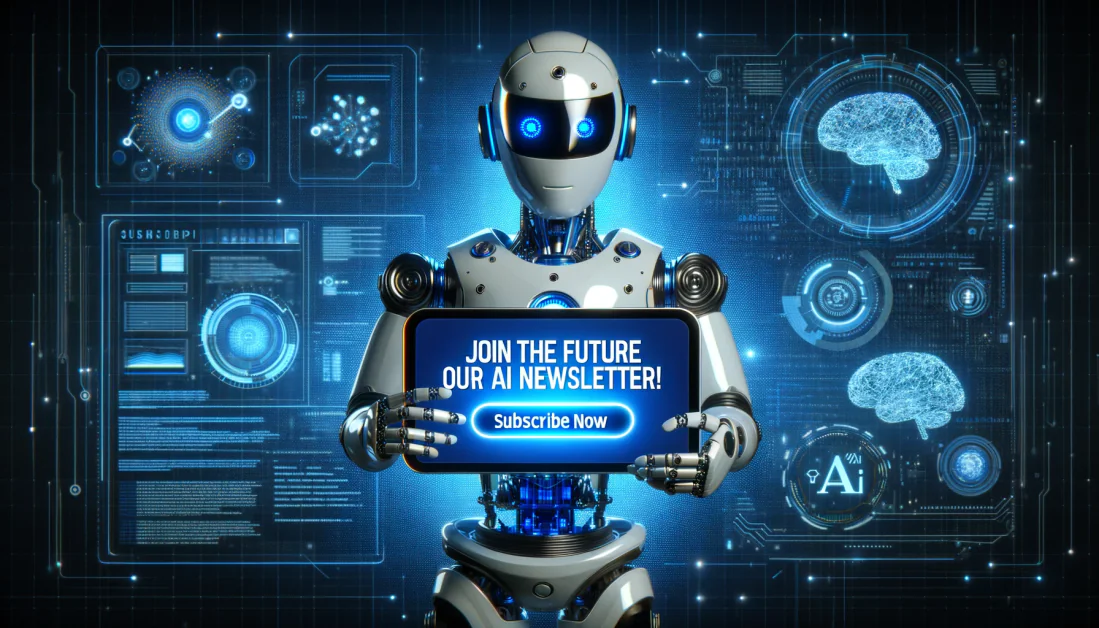
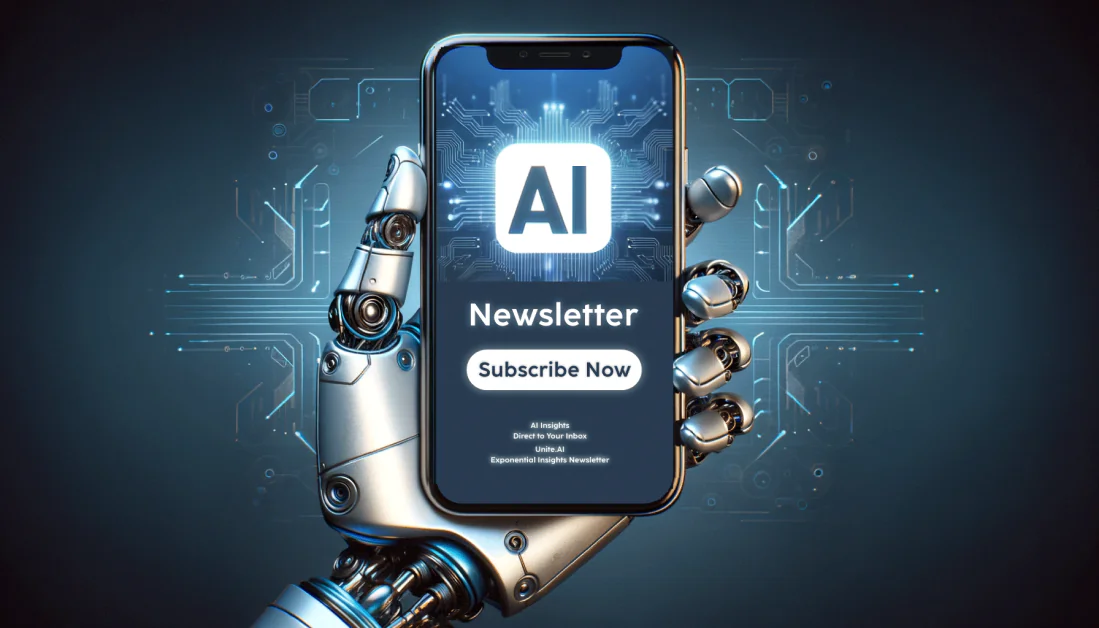