Artificial Intelligence
Machine Learning vs Data Science: Key Differences
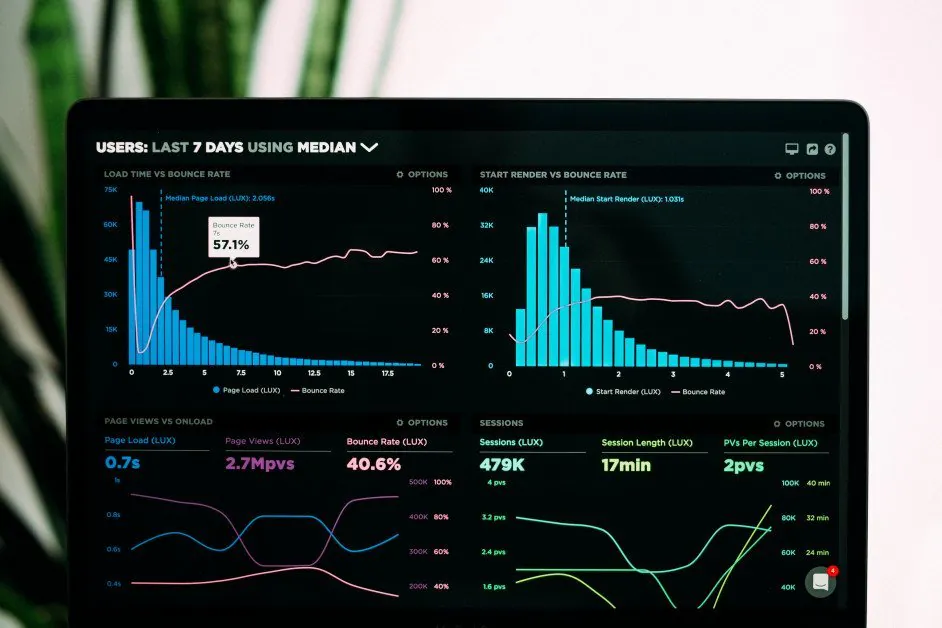
Machine learning (ML) and data science are two separate concepts that are related to the field of artificial intelligence (AI). Both concepts rely on data to improve products, services, systems, decision-making processes, and much more. Both machine learning and data science are also highly sought after career paths in our current data-driven world.
Both ML and data science are used by data scientists in their field of work, and they are being adopted in almost every industry. For anyone looking to get involved in these fields, or any business leader looking to adopt an AI-driven approach into their organization, understanding these two concepts is crucial.
What is Machine Learning?
Machine learning is often used interchangeably with artificial intelligence, but that is incorrect. It is a separate technique and branch of AI that relies on algorithms to extract data and predict future trends. Software programmed with models helps engineers conduct techniques like statistical analysis to help better understand patterns within data sets.
Machine learning is what gives machines the ability to learn without being explicitly programmed, which is why major companies and social media platforms, such as Facebook, Twitter, Instagram, and YouTube use it to predict interests and recommend services, products, and more.
As a set of tools and concepts, machine learning is a part of data science. With that said, its reach goes far beyond the field. Data scientists usually rely on machine learning to gather information quickly and improve trend analysis.
When it comes to machine learning engineers, these professionals require a wide-range of skills, such as:
-
Deep understanding of statistics and probability
-
Expertise in computer science
-
Software engineering and systems design
-
Programming knowledge
-
Data modeling and analysis
What is Data Science?
Data science is the study of data and how to extract meaning from it by using a series of methods, algorithms, tools, and systems. All of these enable experts to extract insights from structured and unstructured data. Data scientists are usually responsible for studying large amounts of data within an organizationβs repository, and the studies often involve content matters and how the data can be leveraged by the company.
By studying structured or unstructured data, data scientists can extract valuable insights about business or marketing patterns, enabling the business to perform better over competitors.
Data scientists apply their knowledge to business, government, and various other bodies to increase profits, innovate products, and build better infrastructure and public systems.
The field of data science has been greatly advanced thanks to the proliferation of smartphones and the digitization of many parts of daily life, which has led to an incredible amount of data available to us. Data science has also been impacted by Mooreβs Law, which refers to the idea that computing dramatically increases in power while decreasing in relative cost over time, leading to the wide-scale availability of cheap computing power. Data science links these two innovations together, and by combining the components, data scientists can extract more insight than ever before from data.
Professionals in the field of data science also require a lot of programming and data analytics skills, such as:
-
Deep understanding of programming languages like Python
-
Ability to work with large amounts of structured and unstructured data
-
Math, statistics, probability
-
Data visualization
-
Data analysis and processing for business
-
Machine learning algorithms and models
-
Communication and team collaboration
Differences Between Machine Learning and Data Science
After defining what each concept is, itβs important to note the major differences between machine learning and data science. Concepts like these, along with others like artificial intelligence and deep learning, can sometimes get confusing and easy to mix up.
Data science is focused on the study of data and how to extract meaning from it, while machine learning involves understanding and constructing methods that use data to improve performance and predictions.
Another way of putting it is that the field of data science determines the processes, systems, and tools that are needed to transform data into insights, which can then be applied throughout different industries. Machine learning is a field of artificial intelligence that enables machines to achieve the human-like capability of learning and adapting through statistical models and algorithms.
Even though these are two separate concepts, there is some overlap. Machine learning is actually part of data science, and the algorithms train on data delivered by data science. They both include some of the same skills like math, statistics, probability, and programming.
Challenges of Data Science and ML
Both data science and machine learning present their own set of challenges, which also helps separate the two concepts.
The primary challenges of machine learning include a lack of data or diversity in the dataset, which makes it difficult to extract valuable insights. A machine cannot learn if there is no available data, while a lacking dataset makes it more difficult to understand patterns. Another challenge of machine learning is that itβs unlikely that an algorithm can extract information when there are no or few variations.
When it comes to data science, its main challenges include the need for a wide variety of information and data for accurate analysis. Another one is that data science results are sometimes not effectively used by the decision makers in a business, and the concept can be hard to explain to teams. It also presents various privacy and ethical issues.
Applications of Each Concept
While data science and machine learning have some overlap when it comes to applications, we can break down each one.
Here are some examples of data science applications:
- Internet Search: Google search relies on data science to search specific results in a fraction of a second.
- Recommendation Systems: Data science is key to the creation of recommendation systems.
- Image/Speech Recognition: Speech recognition systems like Siri and Alexa rely on data science, as do image recognition systems.
- Gaming: The world of gaming uses data science technology to enhance the gaming experience.
Here are some example applications of machine learning:
- Finance: Machine learning is used widely throughout the finance industry, with banks relying on it to identify patterns inside data and to prevent fraud.
- Automation: Machine learning helps automate tasks within various industries, such as robots in manufacturing plants.
- Government: Machine learning is not just used in the private sector. Government organizations use it to manage public safety and utilities.
- Healthcare: Machine learning is disrupting the healthcare industry in many ways. It was one of the first industries to adopt machine learning with image detection.
If you are looking to gain some of the skills within these fields, make sure to check out our lists of best certifications for data science and machine learning.