Artificial Intelligence
Google’s LipSync3D Offers Improved ‘Deepfaked’ Mouth Movement Synchronization

A collaboration between Google AI researchers and the Indian Institute of Technology Kharagpur offers a new framework to synthesize talking heads from audio content. The project aims to produce optimized and reasonably-resourced ways to create ‘talking head' video content from audio, for the purposes of synching lip movements to dubbed or machine-translated audio, and for use in avatars, in interactive applications, and in other real-time environments.
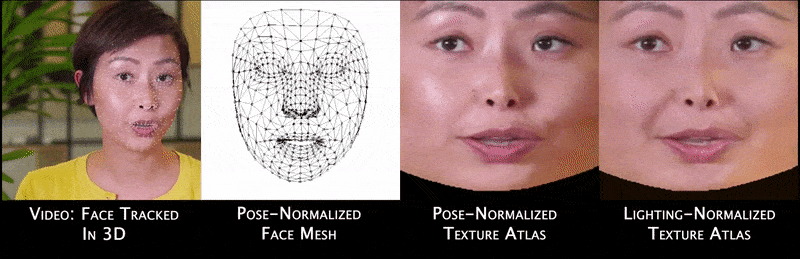
Source: https://www.youtube.com/watch?v=L1StbX9OznY
The machine learning models trained in the process – called LipSync3D – require only a single video of the target face identity as input data. The data preparation pipeline separates extraction of facial geometry from evaluation of lighting and other facets of an input video, allowing more economical and focused training.
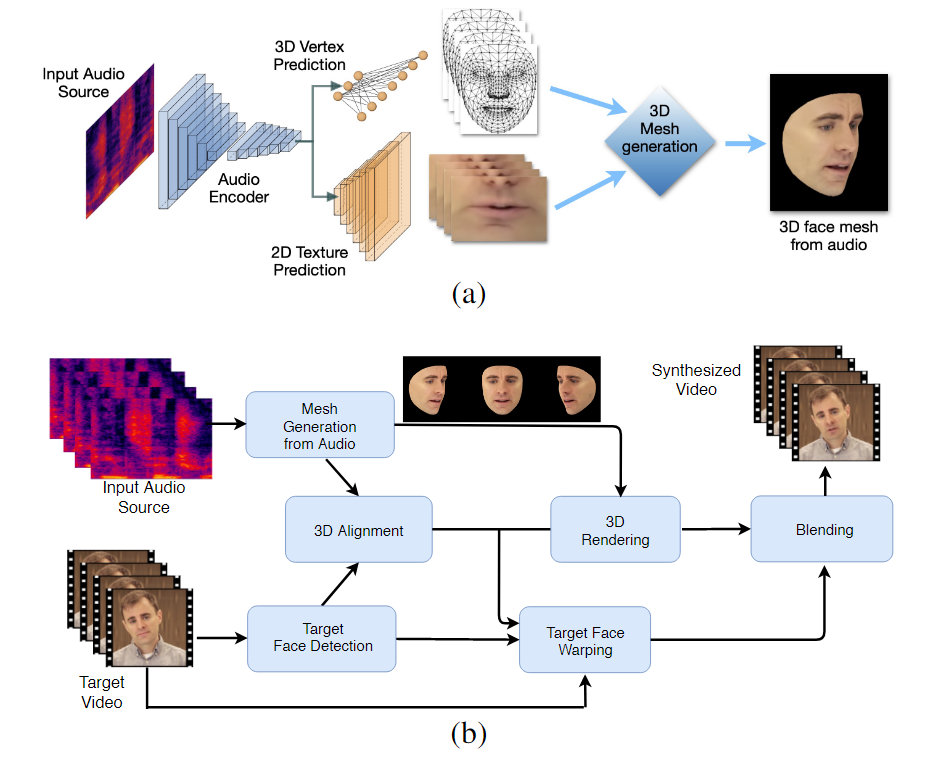
The two-stage work-flow of LipSync3D. Above, the generation of a dynamically textured 3D face from the ‘target' audio; below, the insertion of the generated mesh into a target video.
In fact, LipSync3D's most notable contribution to the body of research effort in this area may be its lighting normalization algorithm, which decouples training and inference illumination.
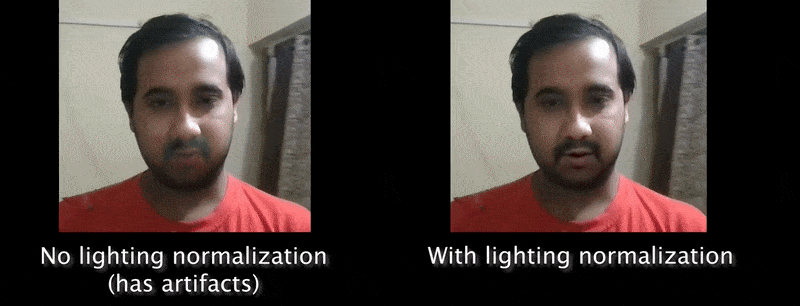
Decoupling illumination data from general geometry helps LipSync3D to produce more realistic lip movement output under challenging conditions. Other approaches of recent years have limited themselves to ‘fixed' lighting conditions that won't reveal their more limited capacity in this respect.
During pre-processing of the input data frames, the system must identify and remove specular points, since these are specific to the lighting conditions under which the video was taken, and will otherwise interfere with the process of relighting.
LipSync3D, as its name suggests, is not performing mere pixel analysis on the faces that it evaluates, but actively using identified facial landmarks to generate motile CGI-style meshes, together with the ‘unfolded' textures that are wrapped around them in a traditional CGI pipeline.
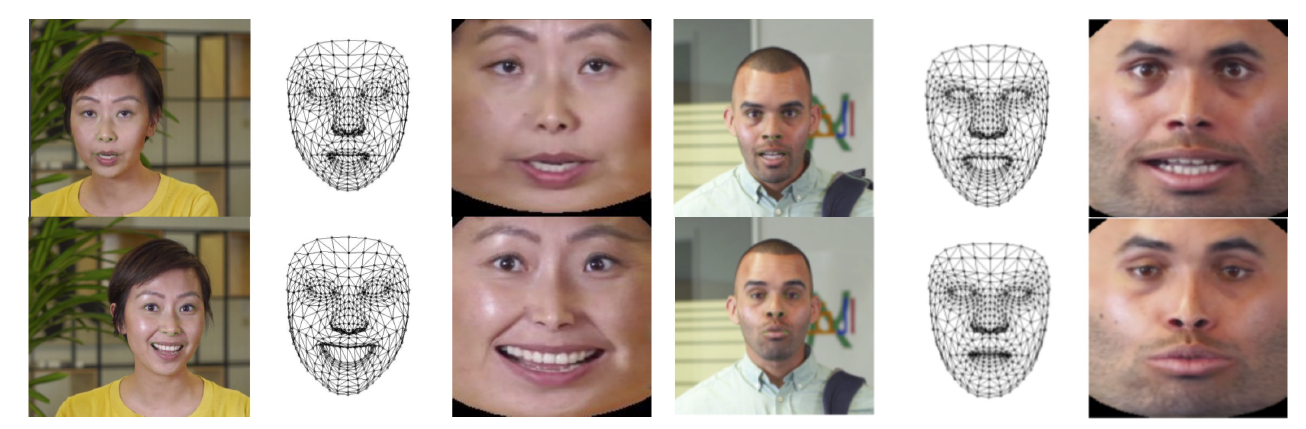
Pose normalization in LipSync3D. On the left are the input frames and detected features; in the middle, the normalized vertices of the generated mesh evaluation; and on the right, the corresponding texture atlas, which provides the ground truth for texture prediction. Source: https://arxiv.org/pdf/2106.04185.pdf
Besides the novel relighting method, the researchers claim that LipSync3D offers three main innovations on previous work: the separation of geometry, lighting, pose and texture into discrete data streams in a normalized space; an easily trainable auto-regressive texture prediction model that produces temporally consistent video synthesis; and increased realism, as evaluated by human ratings and objective metrics.
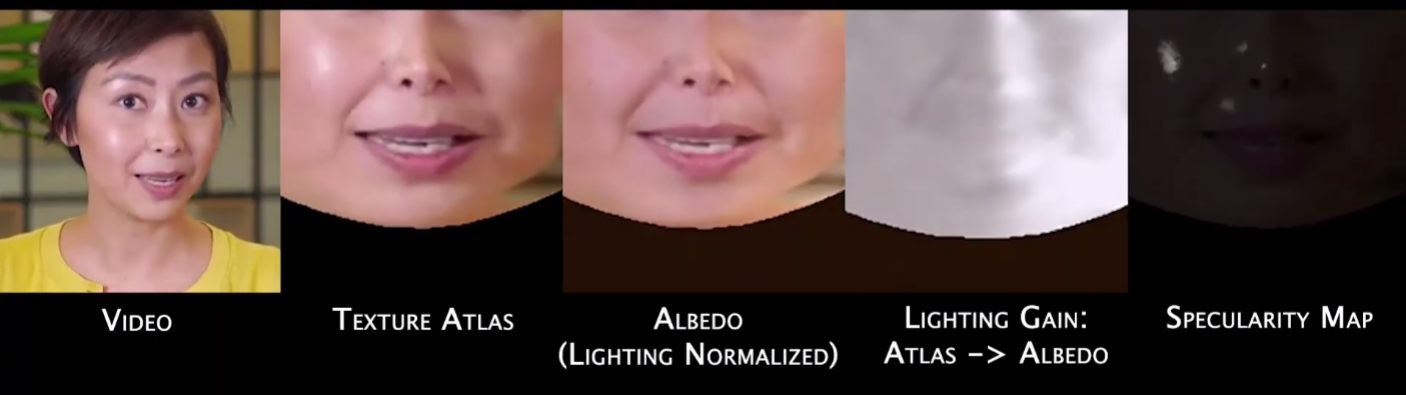
Splitting out the various facets of the video facial imagery allows greater control in video synthesis.
LipSync3D can derive appropriate lip geometry movement directly from audio by analyzing phonemes and other facets of speech, and translating them into known corresponding muscle poses around the mouth area.
This process uses a joint-prediction pipeline, where the inferred geometry and texture have dedicated encoders in an autoencoder set-up, but share an audio encoder with the speech that is intended to be imposed on the model:
LipSync3D's labile movement synthesis is also intended to power stylized CGI avatars, which in effect are only the same kind of mesh and texture information as real-world imagery:
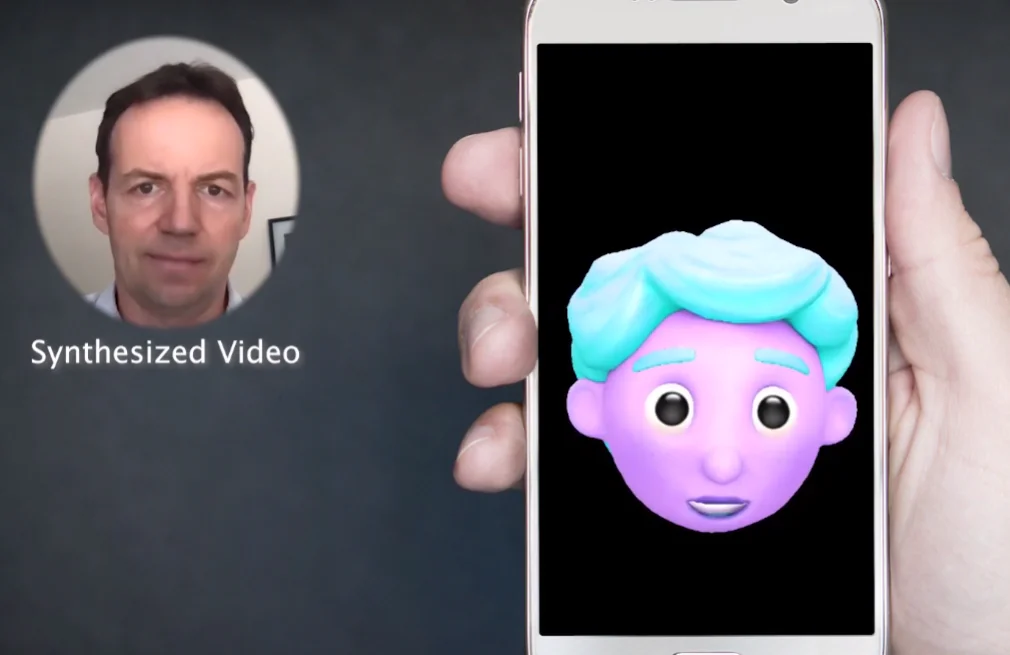
A stylized 3D avatar has its lip movements powered in real time by a source speaker video. In such a scenario, the best results would be obtained by personalized pre-training.
The researchers also anticipate the use of avatars with a slightly more realistic feel:
Sample training times for the videos range from 3-5 hours for a 2-5 minute video, in a pipeline that uses TensorFlow, Python and C++ on a GeForce GTX 1080. The training sessions used a batch size of 128 frames over 500-1000 epochs, with each epoch representing a complete evaluation of the video.
Towards Dynamic Re-Synching Of Lip Movement
The field of re-synching lips to accommodate a novel audio track has received a great deal of attention in computer vision research in the last few years (see below), not least as it's a by-product of controversial deepfake technology.
In 2017 the University of Washington presented research capable of learning lip sync from audio, using it to change the lip movements of then-president Obama. In 2018; the Max Planck Institute for Informatics led another research initiative to enable identity>identity video transfer, with lip synch a by-product of the process; and in May of 2021 AI startup FlawlessAI revealed its proprietary lip-sync technology TrueSync, widely received in the press as an enabler of improved dubbing technologies for major film releases across languages.
And, of course, the ongoing development of deepfake open source repositories provides another branch of active user-contributed research in this sphere of facial image synthesis.
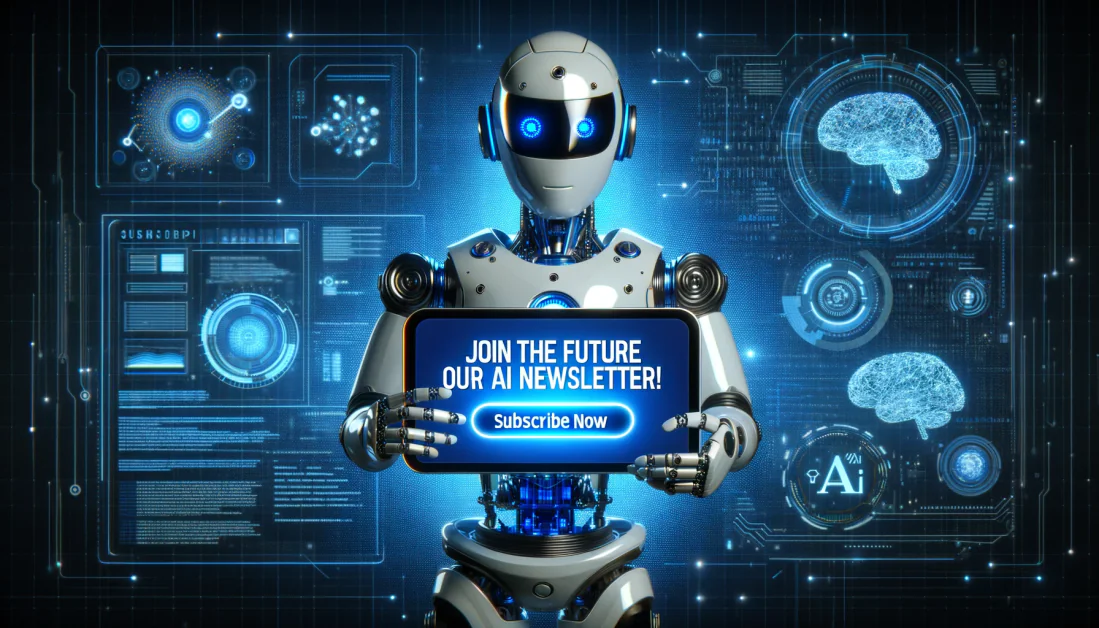
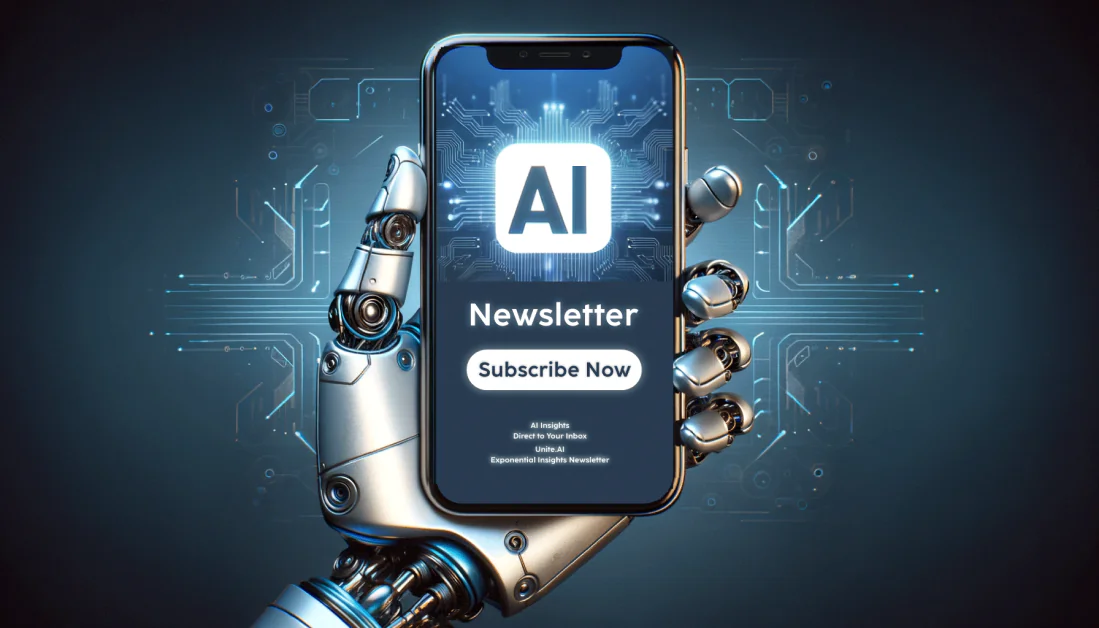