Thought Leaders
Donât Sleep on Your Database Infrastructure When Building Large Language Models or Generative AI
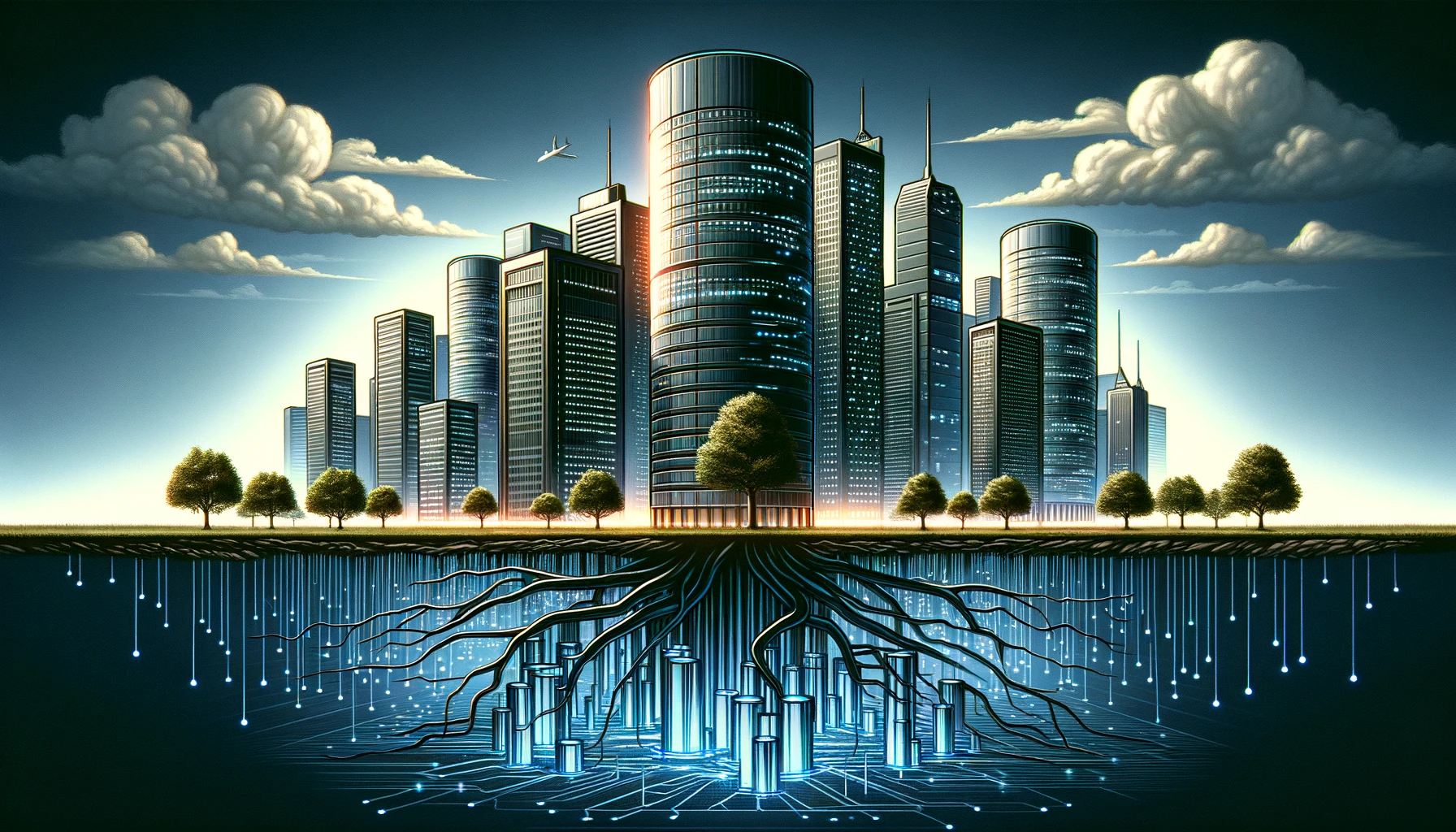
When youâre walking through a city, itâs only natural to look up. The towering skyscrapers seem like impossible feats of engineering. Rising dozens or even hundreds of stories above the ground, they weather lightning strikes, superstorms, and the ravages of time. Skyscrapers are a testament to what can be achieved through strategic design and innovative engineering. However, itâs the unseen, underground foundation that makes these gravity-defying structures possible.
Think of artificial intelligence (AI) systems like those skyscrapers. Just as a building relies on a robust foundation to remain upright in the city skyline, AI systems depend on a solid database infrastructure for reliability, efficiency, and intelligence. This isnât just about having a place to store data; itâs about creating an organized, efficient system capable of managing and processing vast amounts of information as the projectâs complexity grows.
Neglecting the database infrastructure in AI projects is like building on quicksand in a quake zone: it makes the entire structure vulnerable. Without a strong foundation, AI systems can suffer in performance, struggle with scalability, or even fail at crucial moments. The outcome? Loss of user trust. This is doubly true for complex AI systems, such as large language models, that process extensive datasets for tasks like language processing, image recognition, and predictive analysis.
Before we dream about the view from the top, database pros and IT leaders must prioritize the scalability, data quality, performance, and security of our databases. Only then can we raise the potential of AI and large language model projects to breathtaking new heights.
Scalability: To Reach New Heights
Imagine a skyscraper built not only to stand tall today but also capable of growing with the city skyline in the future. This is how we should approach the storage needs of AI data. Every new floor (or, in AIâs case, every new dataset or feature) must be supported by the infrastructure below. This requires scalable databases that can expand along with an organization, helping ensure that AI systems remain fast, secure, and intelligent no matter how large, interdependent, or complex they become. In addition to storage space, teams must consider computing and input/output operations to prevent downtime as the database handles the increasing demands of advanced AI applications.
Architects use modern techniques such as steel frames and modular construction to add more floors to a skyscraper. Similarly, AI relies on cloud-based solutions and strategic methods like data indexing, sharding, and partitioning to distribute workloads evenly across the system. This ensures the infrastructure can handle increased data needs smoothly, keeping the AI system robust and responsive. Moreover, it helps organizations avoid bottlenecks and growing pains as they scale up.
In cloud computing, there are two main strategies for increasing system capacity: scaling up and scaling out. Scaling up means boosting the capacity of existing infrastructure, while scaling out is like adding more buildings to a complex. This means increasing resources like servers or nodes to enhance the capacity. Both methods are crucial for developing robust AI systems that can handle growing demands and complexities.
Data Quality: For Unshakeable Walls
Data is the backbone of every modern enterprise, and its quality and integrity are as essential as the steel frameworks that help skyscrapers withstand any weight or weather. An AI's performance directly depends on the quality of the data it is trained on. Therefore, companies must continuously commit to updating and maintaining their databases to ensure they're accurate, consistent, and up to date.
Similar to routine inspections that verify a skyscraper is stable enough to stay standing, the databases underpinning AI need consistent attention. Teams should be continually updating their databases to reflect the most current information. This entails validating them to ensure data correctness and cleansing them to remove inaccuracies. By doing so, enterprises can ensure that their systems remain unshakable in the face of challenges and continue to deliver accurate and dependable results.
Performance Optimization: To Keep The Lights On
Consider what would happen if a skyscraperâs essential systemsâlike electricity, water, or elevatorsâsuddenly failed. (Spoiler alert: it would very quickly become uninhabitable.) Suppose you donât get excited about the prospect of getting onto an elevator that hasnât been inspected in years or working on the 99th floor of a building with shoddy electricity. In that case, you probably shouldnât leave your critical databases to their own devices, either. Evaluating and enhancing databases to ensure they remain relevant and efficient is necessary to keep AI from becoming outdated, much like a building can deteriorate without proper upkeep.
In the enterprise world, database deterioration can result in decreased accuracy, slower response times, and an inability to handle emerging threats. Just as architects choose specific designs and materials to reduce wind impact and boost a building's energy efficiency, AI architects use query optimization and catching to ensure systems perform as needed. The systems must process and analyze data effectively, regardless of outside conditions. Similarly to how engineers monitor a skyscraper's structural integrity and environmental systems, database monitoring can help proactively detect and address slow queries, resource bottlenecks, and unexpected database behaviors that could hinder AI projects.
Security Measures: The Foundation of Trust
Cybersecurity protocols are essential for protecting an organization's sensitive data. Security personnel, surveillance cameras, and access controls in a building help ensure the safety of its residents; cybersecurity protocols, such as Secure by Design principles and multi-factor authentication, play a crucial role in safeguarding an organization's data integrity.
In a world where data is as valuable as gold, it is crucial to ensure its confidentiality. Security is not just a technical requirement for AI systems; it lays the groundwork upon which trust is built, ethical standards are maintained, and innovation is spurred. In a way, these security measures are fundamental to the rest of the foundation. They not only help AI systems perform tasks but also protect the interests and privacy of the human teams they serve.
Database teams can help keep their AI systems secure by conducting regular security audits to identify and fix potential vulnerabilities. By prioritizing security at every layer of their infrastructureâfrom monitoring to maintenance and everything in betweenâ organizations can ensure that their AI systems are trusted sanctuaries for valuable data.
When developers and users feel confident in the security of AI systems, they are more likely to experiment and push the boundaries of what these technologies can achieve. We must continue to build and manage these critical foundations with diligence and foresight. That way, we can ensure our AI systems remain reliable, effective, and capable of reaching their full potential.