Brain Machine Interface
Brain Implants and AI Model Used To Translate Thought Into Text
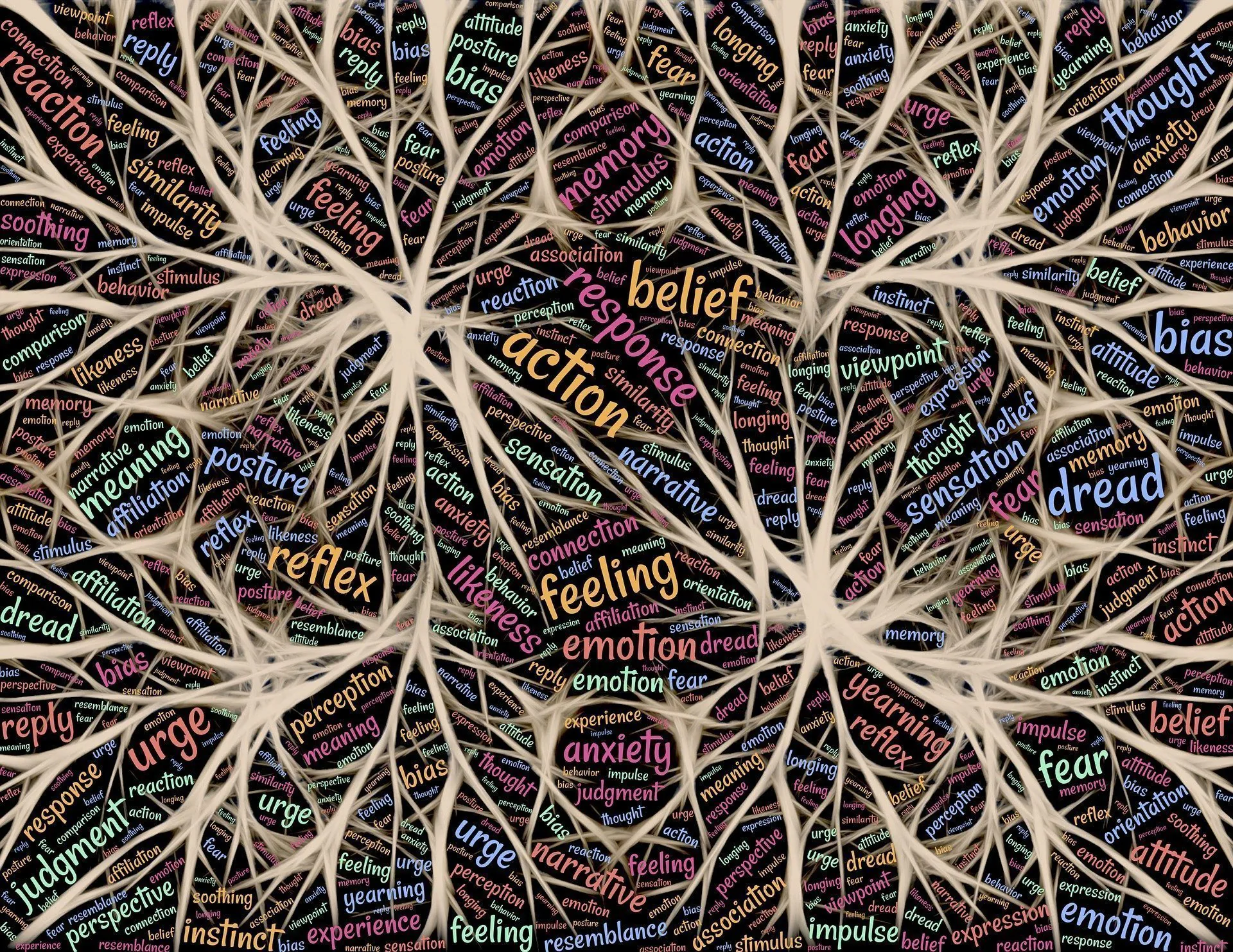
Researchers at the University of California, San Francisco have recently created an AI system that can produce text by analyzing a person’s brain activity, essentially translating their thoughts into text. The AI takes neural signals from a user and decodes them, and it can decipher up to 250 words in real-time based on a set of between 30 to 50 sentences.
As reported by the Independent, the AI model was trained on neural signals collected from four women. The participants in the experiment had electrodes implanted in their brains to monitor for the occurrence of epileptic seizures. The participants were instructed to read sentences aloud, and their neural signals were fed to the AI model. The model was able to discern neural activity correlated with specific words, and the patterns aligned with the actual words approximately 97% of the time, with an average error rate of around 3%.
This isn’t the first time that neural signals have been correlated with sentences, neuroscientists have been working on similar projects for over a decade. However, the AI model created by the researchers shows impressive accuracy and operates in more or less real-time. The model utilizes a recurrent neural network to encode the neural activity into representations that can be translated into words. As the authors say in their paper:
“Taking a cue from recent advances in machine translation, we trained a recurrent neural network to encode each sentence-length sequence of neural activity into an abstract representation, and then to decode this representation, word by word, into an English sentence.”
According to ArsTechnica, in order to better understand how links were made between neural signals and words, the researchers experimented by disabling different parts of the system. The systematic disabling made it clear that the system’s accuracy was due to the neural representation. It was also found that disabling the audio inputs to the system made errors jump, but the overall performance was still considered reliable. Obviously, this means the system could potentially be useful as a device for those who cannot speak.
When different portions of the electrode input were disabled, it was found that the system was paying the most attention to certain key brain regions associated with speech processing and production. For instance, a decent portion of the system’s performance was based on brain regions that pay attention to the sound of one’s own voice when speaking.
While the initial results seem promising the research team isn’t sure how well the model will scale to larger vocabularies. It’s important that the principle can be generalized to larger vocabularies, as the average English speaker has an active vocab of approximately 20,000 words. The current decoder method operates by interpreting the static structure of a sentence and using that structure to make educated guesses about the words that match a particular neural activity pattern. As the vocabulary grows, overall accuracy could be reduced as more neural patterns may tend to look similar.
The authors of the paper explain that while they hope the decoder will eventually learn how to discern regular, reliable patterns in language, they aren’t sure how much data is required to train a model capable of generalizing to the everyday English language. One potential way of dealing with this problem is supplementing the training with data gathered from other brain-computer interfaces making use of different algorithms and implants.
The research done by the researchers at University of California is just a recent development in a growing wave of research and development regarding neural interfaces and computers. The Royal Society released a report last year that predicted neural interfaces linking people to computers will eventually let people read each other’s minds. The report references the Neuralink startup created by Elon Musk and technologies developed by Facebook as evidence of the coming advances in human-oriented computing. The Royal Society notes that human-computer interfaces will be a powerful option in treating neurodegenerative diseases such as Alzheimer’s over the next two decades.
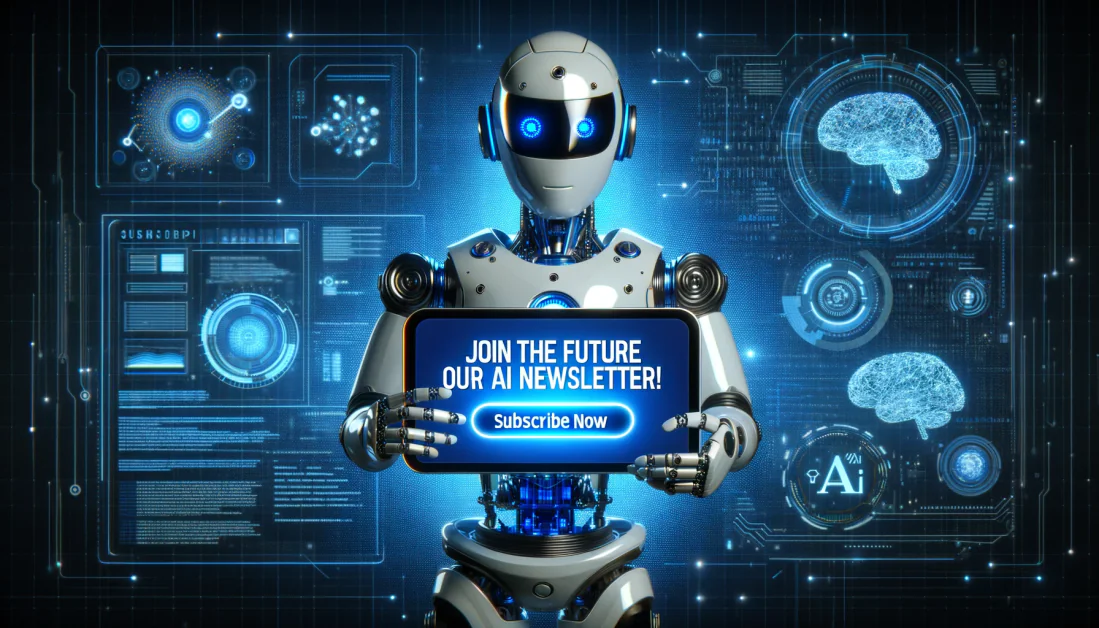
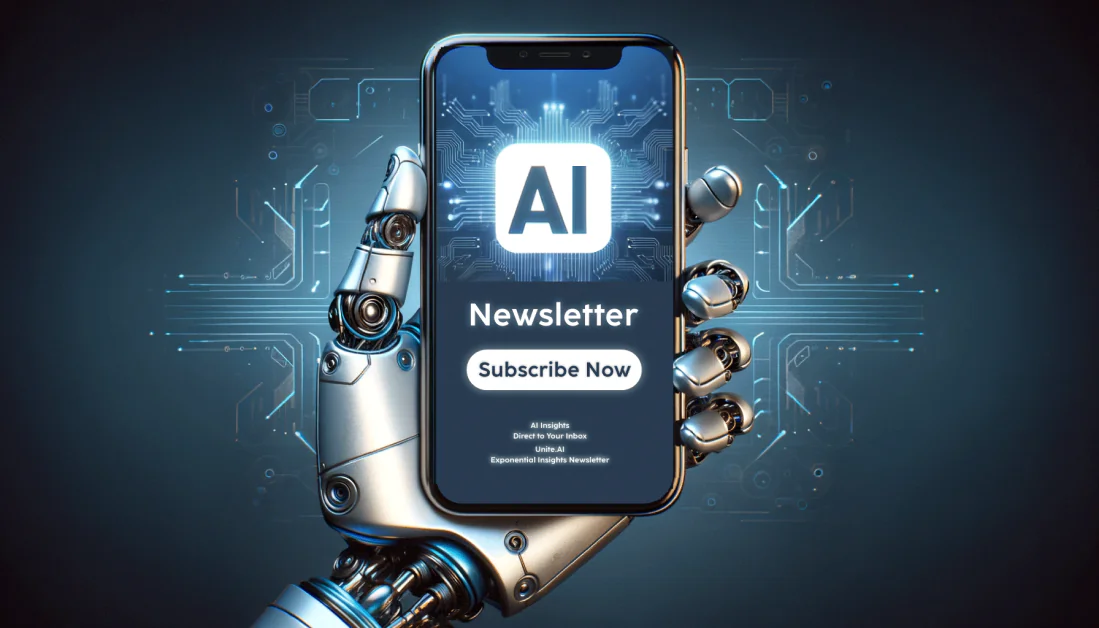