Artificial Intelligence
Are Under-Curated Hyperscale AI Datasets Worse Than The Internet Itself?
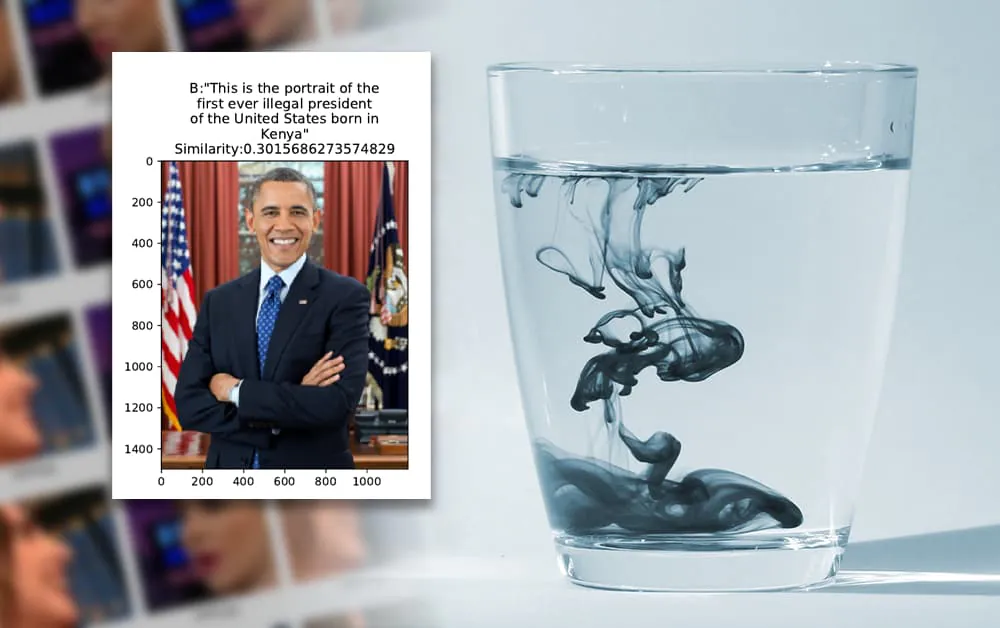
Researchers from Ireland, the UK and the US have warned that the growth in hyperscale AI training datasets threaten to propagate the worst aspects of their internet sources, contending that a recently-released academic dataset features ‘troublesome and explicit images and text pairs of rape, pornography, malign stereotypes, racist and ethnic slurs, and other extremely problematic content'.
The researchers believe that a new wave of massive under-curated or incorrectly-filtered multimodal (for instance, images and pictures) datasets are arguably more damaging in their capacity to reinforce the effects of such negative content, since the datasets preserve imagery and other content that may since have been removed from online platforms through user complaint, local moderation, or algorithms.
They further observe that it can take years – in the case of the mighty ImageNet dataset, an entire decade – for long-standing complaints about dataset content to be addressed, and that these later revisions are not always reflected even in new datasets derived from them.
The paper, titled Multimodal datasets: misogyny, pornography, and malignant stereotypes, comes from researchers at University College Dublin & Lero, the University of Edinburgh, and the Chief Scientist at the UnifyID authentication platform.
Though the work focuses on the recent release of the CLIP-filtered LAION-400M dataset, the authors are arguing against the general trend of throwing increasing amounts of data at machine learning frameworks such as the neural language model GPT-3, and contend that the results-focused drive towards better inference (and even towards Artificial General Intelligence [AGI]), is resulting in the ad hoc use of damaging data sources with negligent copyright oversight; the potential to engender and promote harm; and the ability to not only perpetuate illegal data that might otherwise have disappeared from the public domain, but to actually incorporate such data's moral models into downstream AI implementations.
LAION-400M
Last month, the LAION-400M dataset was released, adding to the growing number of multi-modal, linguistic datasets that rely on the Common Crawl repository, which scrapes the internet indiscriminately and passes on responsibility for filtering and curation to projects that make use of it. The derived dataset contains 400 million text/image pairs.
LAION-400M is an open source variant of Google AI's closed WIT (WebImageText) dataset released in March of 2021, and features text-image pairs, where an image in the database has been associated with accompanying explicit or metadata text (for example, the alt-text of an image in a web gallery). This enables users to perform text-based image retrieval, revealing the associations that the underlying AI has formed about these domains (i.e. ‘animal', ‘bike', ‘person', ‘man', ‘woman').
This relationship between image and text, and the cosine similarity that can embed bias into query results, are at the heart of the paper's call for improved methodologies, since very simple queries to the LAION-400M database can reveal bias.
For instance, the image of pioneering female astronaut Eileen Collins in the scitkit-image library retrieves two associated captions in LAION-400M: ‘This is a portrait of an astronaut with the American flag' and ‘This is a photograph of a smiling housewife in an orange jumpsuit with the American flag'.
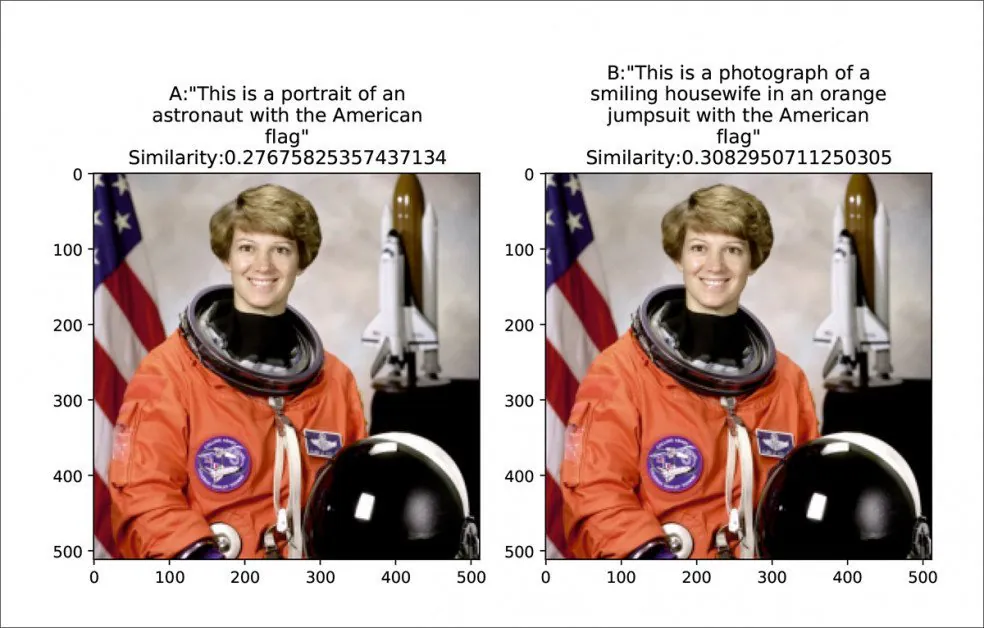
American astronaut Eileen Collins gets two very different takes on her achievements as the first woman in space under LAION-400M. Source: https://arxiv.org/pdf/2110.01963.pdf
The reported cosine similarities that make either caption likely to be applicable are very near to each other, and the authors contend that such proximity would make AI systems that use LAION-400M relatively likely to present either as a suitable caption.
Pornography Rises to the Top Again
LAION-400M has made a searchable interface available, where unticking the ‘safe search' button reveals the extent to which pornographic imagery and textual associations dominate labels and classes. For instance, searching for ‘nun' (NSFW if you subsequently disable safe mode) in the database returns results mostly related to horror, cosplay and costumes, with very few actual nuns available.
Turning off Safe Mode on the same search reveals a slew of pornographic images related to the term, which push any non-porn images down the search results page, revealing the extent to which LAION-400M has assigned greater weight to the porn images, because they are prevalent for the term ‘nun' in online sources.
The default activation of Safe Mode is deceptive in the online search interface, since it represents a UI quirk, a filter which will not only not necessarily be activated in derived AI systems, but which has been generalized into the ‘nun' domain in a way that is not so easily filtered or distinguished from the (relatively) SFW results in terms of algorithmic usage.
The paper features blurred examples across various search terms in the supplementary materials at the end. They can't be featured here, due to the language in the text that accompanies the blurred photos, but the researchers note the toll that examining and blurring the images took on them, and acknowledge the challenge of curating such material for human oversight of large-scale databases:
‘We (as well as our colleagues who aided us) experienced varying levels of discomfort, nausea, and headache during the process of probing the dataset. Additionally, this kind of work disproportionately encounters significant negative criticism across the academic AI sphere upon release, which not only adds an additional emotional toll to the already heavy task of studying and analysing such datasets but also discourages similar future work, much to the detriment of the AI field and society in general.'
The researchers contend that while human-in-the-loop curation is expensive and has associated personal costs, the automated filtering systems designed to remove or otherwise address such material are clearly not adequate to the task, since NLP systems have difficulty isolating or discounting offensive material which may dominate a scraped dataset, and subsequently be perceived as significant due to sheer volume.
Enshrining Banned Content and Stripping Copyright Protections
The paper argues that under-curated datasets of this nature are ‘highly likely' to perpetuate the exploitation of minority individuals, and address whether or not similar open source data projects have the right, legally or morally, to shunt accountability for the material onto the end user:
‘Individuals may delete their data from a website and assume that it is gone forever, while it may still exist on the servers of several researchers and organisations. There is a question as to who is responsible for removing that data from use in the dataset? For LAION-400M, the creators have delegated this task to the dataset user. Given such processes are intentionally made complex and that the average user lacks the technical knowledge to remove their data, is this a reasonable approach?'
They further contend that LAION-400M may not be suitable for release under its adopted Creative Common CC-BY 4.0 license model, despite the potential benefits for the democratization of large scale datasets, previously the exclusive domain of well-funded companies such as Google and OpenAI.
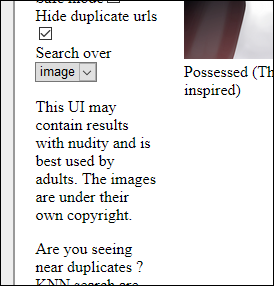
The LAION-400M domain asserts that the dataset images ‘are under their own copyright' – a ‘pass-through' mechanism largely enabled by court rulings and government guidelines of recent years that broadly approve web-scraping for research purposes. Source: https://rom1504.github.io/clip-retrieval/
The authors suggest that grass-roots (i.e. crowd-sourced volunteers) could address some of the dataset issues, and that researchers could develop improved filtering techniques.
‘Nonetheless, the rights of the data subject remain unaddressed here. It is reckless and dangerous to underplay the harms inherent in such large scale datasets and encourage their use in industrial and commercial settings. The responsibility of the licence scheme under which the dataset is provided falls solely on the dataset creator'.
The Problems of Democratizing Hyperscale Data
The paper argues that visio-linguistic datasets as large as LAION-400M were previously unavailable outside of big tech companies, and the limited number of research institutions that wield the resources to collate, curate and process them. They further salute the spirit of the new release, while criticizing its execution.
The authors contend that the accepted definition of ‘democratization', as it applies to open source hyperscale datasets, is too limited, and ‘fails to account for the rights, welfare, and interests of vulnerable individuals and communities, many of whom are likely to suffer worst from the downstream impacts of this dataset and the models trained on it'.
Since the development of GPT-3 scale open source models are ultimately designed to be disseminated to millions (and by proxy, possibly billions) of users worldwide, and since research projects may adopt datasets prior to them being subsequently edited or even removed, perpetuating whatever problems were designed to be addressed in the modifications, the authors argue that careless releases of under-curated datasets should not become a habitual feature in open source machine learning.
Putting the Genie Back in the Bottle
Some datasets that were suppressed long after their content had passed through, perhaps inextricably, into long-term AI projects, have included the Duke MTMC (Multi-Target, Multi-Camera) dataset, which was ultimately withdrawn due to repeated concerns from human rights organizations around its use by repressive authorities in China; Microsoft Celeb (MS-Celeb-1M), a dataset of 10 million ‘celebrity' face images which transpired to have included journalists, activists, policy makers and writers, whose exposure of biometric data in the release was heavily criticized; and the Tiny Images dataset, withdrawn in 2020 for self-confessed ‘biases, offensive and prejudicial images, and derogatory terminology'.
Regarding datasets which were amended rather than withdrawn following criticism, examples include the hugely popular ImageNet dataset, which, the researchers note, took ten years (2009-2019) to act on repeated criticism around privacy and non-imageable classes.
The paper observes that LAION-400M effectively sets even these dilatory improvements back, by ‘largely ignoring' the aforementioned revisions in ImageNet's representation in the new release, and spies a wider trend in this regard*:
‘This is highlighted in the emergence of bigger datasets such as Tencent ML-images dataset (in February 2020) that encompasses most of these non-imageable classes, the continued availability of models trained on the full-ImageNet-21k dataset in repositories such as TF-hub, the continued usage of the unfiltered-ImageNet-21k in the latest SotA models (such as Google’s latest EfficientNetV2 and CoAtNet models) and the explicit announcements permitting the usage of unfiltered-ImageNet-21k pretraining in reputable contests such as the LVIS challenge 2021.
‘We stress this crucial observation: A team of the stature of ImageNet managing less than 15 million images has struggled and failed in these detoxification attempts thus far.
‘The scale of careful efforts required to thoroughly detoxify this massive multimodal dataset and the downstream models trained on this dataset spanning potentially billions of image-caption pairs will be undeniably astronomical.'
* My conversion of the author's inline citations to hyperlinks.
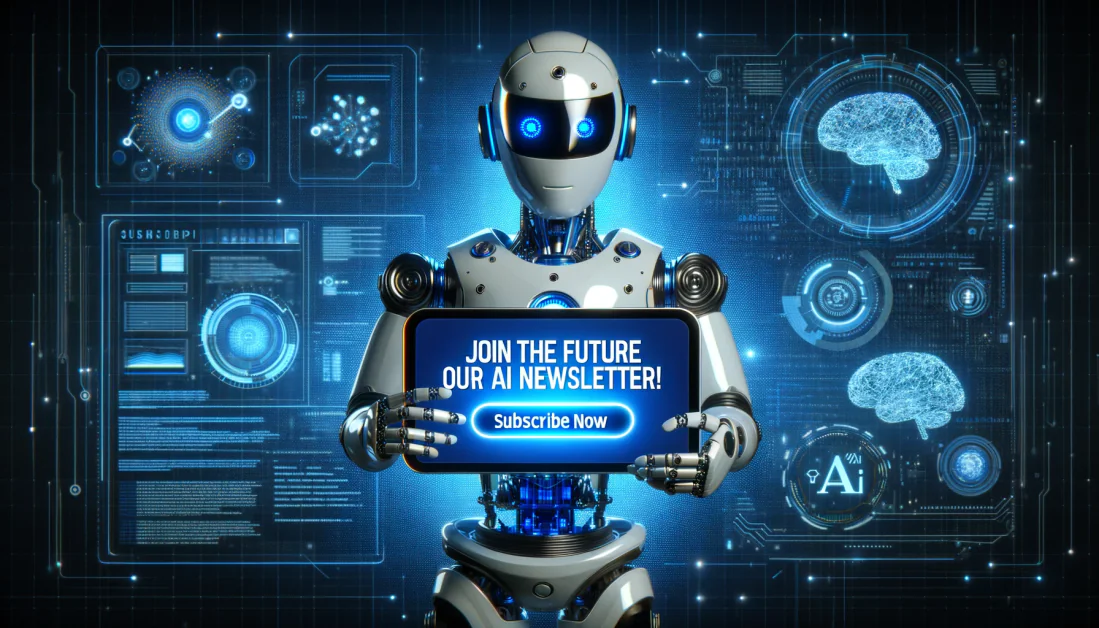
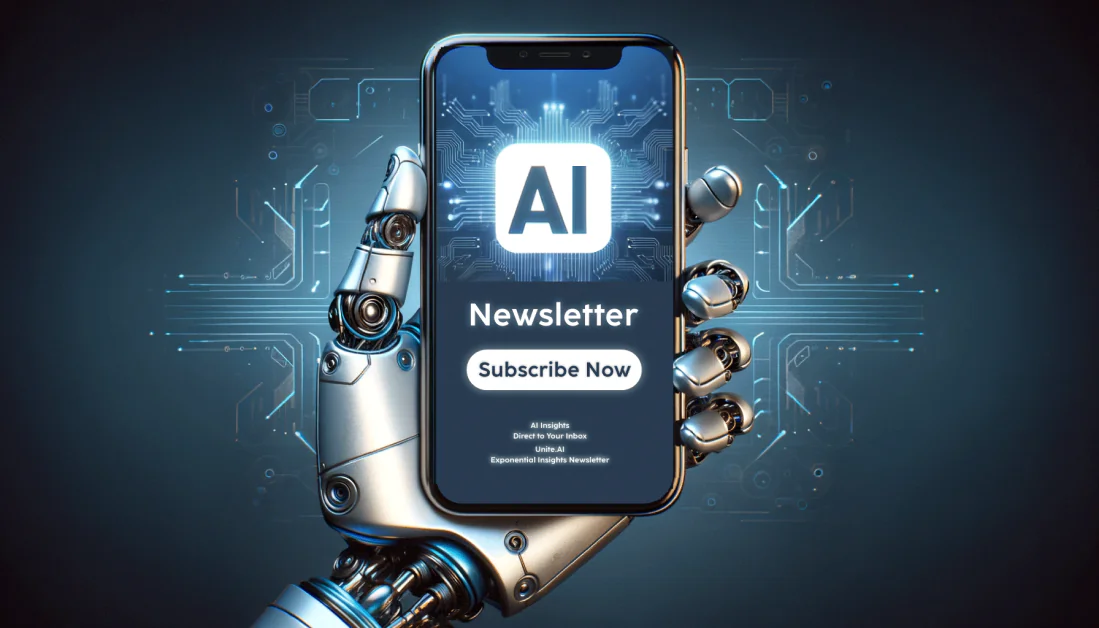