Artificial Intelligence
AI Model Can Take Blurry Images And Enhance Resolution By 60 Times
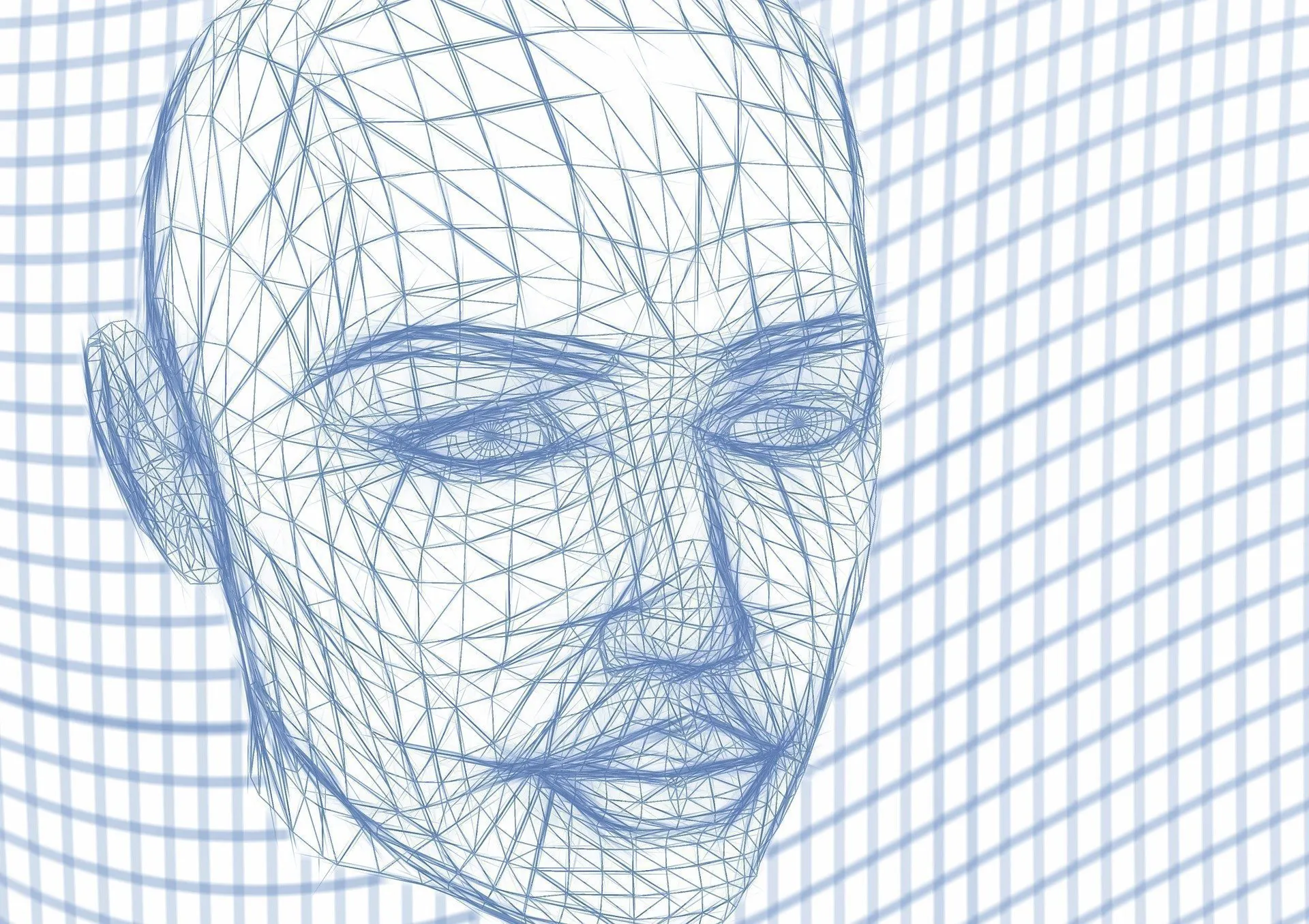
Researchers from Duke University have developed an AI model capable of taking highly blurry, pixellated images and rendering them with high detail. According to TechXplore, the model is capable of taking relatively few pixels and scaling the images up to create realistic looking faces that are approximately 64 times the resolution of the original image. The model hallucinates, or imagines, features that are between the lines of the original image.
The research is an example of super-resolution. As Cynthia Rudin from Duke Universityâs computer science team explained to TechXplore, this research project sets a record for super-resolution, as never before have images been created with such feal from such a small sample of starting pixels. The researchers were careful to emphasize that the model doesnât actually recreate the face of the person in the original, low-quality image. Instead, it generates new faces, filling in details that werenât there before. For this reason, the model couldnât be used for anything like security systems, as it wouldnât be able to turn out of focus images into images of a real person.
Traditional super-resolution techniques operate by making guesses about what pixels are needed to turn the image into a high-resolution image, based on images that the model has learned about beforehand. Because the added pixels are the result of guesses, not all the pixels will match with their surrounding pixels and certain regions of the image may look fuzzy or warped. The researchers from Duke University used a different method of training their AI model. The model created by the Duke researchers operates by first taking low-resolution images and adding detail to the image over time, referencing high-resolution AI-generated faces as examples. The model references the AI-generated faces and tries to finds ones that resemble the target images when the generated faces are scaled down to the size of the target image.
The research team created a Generative Adversarial Network model to handle the creation of new images. GANs are actually two neural networks that are both trained on the same dataset and pitted against one another. One network is responsible for generating fake images that mimic the real images in the training dataset, while the second network is responsible for detecting the fake images from the genuine ones. The first network is notified when its images have been identified as fake, and it improves until the fake images are hopefully indistinguishable from the genuine images.
The researchers have dubbed their super-resolution model PULSE, and the model consistently produces high-quality images even if given images so blurry that other super-resolution methods canât create high-quality images from them. The model is even apable of making realistic looking faces from images where the features of the face are almost indistinguishable. For instance, when given an image of a face with 16×16 resolution, it can create a 1024 x 1024 image. More than a million pixels are added during this process, filling in details like strands of hair, wrinkles, and even lighting. When the researchers had people rate 1440 PULSE generated images against images generated by other super-resolution techniques, the PULSE generated images consistently scored the best.
While the researchers used their model on images of peopleâs faces, the same techniques they use could be applied to almost any object. Low-resolution images of various objects could be used to create high-resolution images of that set of objects, opening-up possible applications for a variety of different industries and fields from microscopy, satellite imagery, education, manufacturing, and medicine.