Artificial Intelligence
AI Browser Tools Aim To Recognize Deepfakes and Other Fake Media
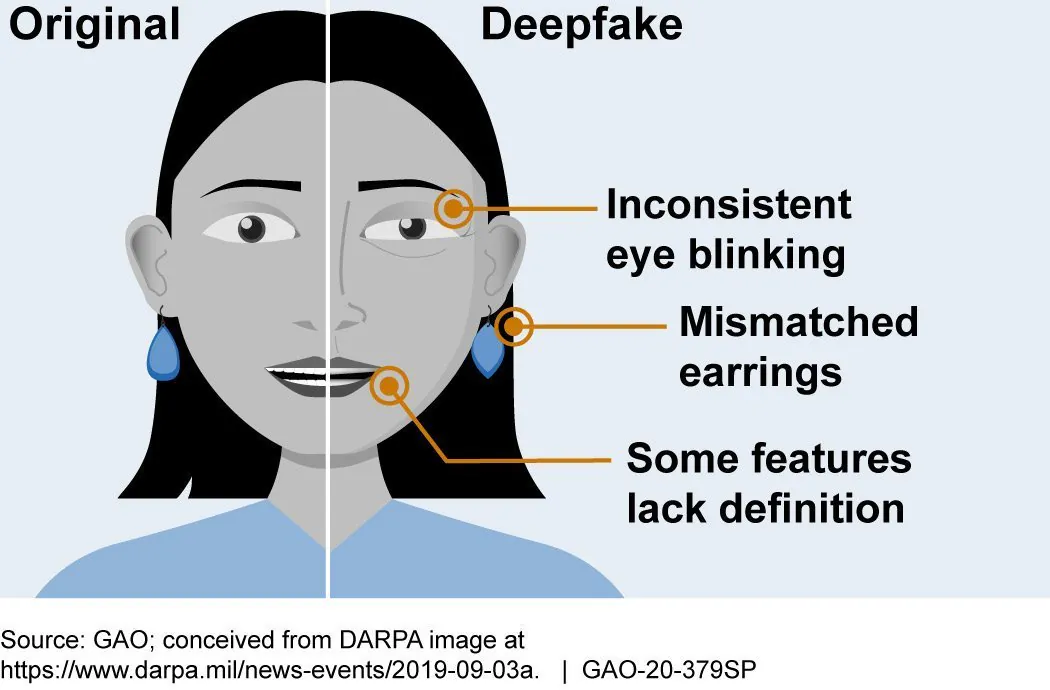
Efforts by tech companies to tackle misinformation and fake content are kicking into high gear in recent times as sophisticated fake content generation technologies like DeepFakes become easier to use and more refined. One upcoming attempt to help people detect and fight deepfakes is RealityDefender, produced by the AI Foundation, which has committed itself to developing ethical AI agents and assistants that users can train to complete various tasks.
The AI Foundation’s most notable project is a platform that allows people to create their own digital personas that look like them and represent them in virtual hangout spaces. The AI Foundation is overseen by the Global AI Council and as part of their mandate they must anticipate the possible negative impacts of AI platforms, then try to get ahead of these problems. As reported by VentureBeat, One of the tools that the AI Foundation has created to assist in the detection of deepfakes is dubbed Reality Defender. Reality Defender is a tool that a person can use in their web browser (check on that), which will analyze video, images, and other types of media to detect signs that the media has been faked or altered in some fashion. It’s hoped that the tool will help counteract the increasing flow of deepfakes on the internet, which according to some estimates have roughly doubled over the course of the past six months.
Reality defender operates by utilizing a variety of AI-based algorithms that can detect clues suggesting an image or video might have been faked. The AI models detect subtle signs of trickery and manipulation, and the false positives the model detects are labeled as incorrect by the users of the tool. The data is then used to retrain the model. AI companies who create non-deceptive deepfakes have their content tagged with an “honest AI” tag or watermark that lets people readily identify the AI-generated fakes.
Reality Defender is just one of a suite of tools and an entire AI responsibility platform that AI Foundation is attempting to create. AI Foundation is pursuing the creation of Guardian AI, a responsibility platform built on the precept that individuals should have access to personal AI agents that work for them and that can help guard against their exploitation by bad actors. Essentially, AI Foundation is aiming to both expand the reach of AI in society, bringing it to more people, while also guarding against the risks of AI.
Reality Defender isn’t the only new AI-driven product aiming to reduce misinformation on the United States. A similar product is called SurfSafe, which was created by two undergraduates from UC Berkeley, Rohan Phadte and Ash Bhat. According to The Verge, SurfSafe operates by allowing its users to click on a piece of media that they are curious about and the program will carry out a reverse image search and try to find similar content from various trusted sources on the internet, flagging images that are known to be doctored.
It’s unclear just how effective these solutions will be in the long run. Dartmouth College professor and forensics expert Hany Farid was quoted by The Verge as saying that he is “extremely skeptical” that plans systems like Reality Defender will work in a meaningful capacity. Farid explained that one of the key challenges with detecting fake content is that media isn’t purely fake or real. Farid explained:
“There is a continuum; an incredibly complex range of issues to deal with. Some changes are meaningless, and some fundamentally alter the nature of an image. To pretend we can train an AI to spot the difference is incredibly naïve. And to pretend we can crowdsource it is even more so.”
Furthermore, it's difficult to include crowdsourcing elements, such as tagging false positives, because humans are typically quite bad at identifying fake images. Humans often make mistakes and miss subtle details that mark an image as fake. It’s also unclear how to deal with bad faith actors who troll when they flag content.
It seems likely that, in order to be maximally effective, fake-detecting tools will have to be combined with digital literacy efforts that teach people how to reason about the content they interact with online.