Thought Leaders
What To Do When COVID Changes Make Your Data Obsolete – Thought Leaders
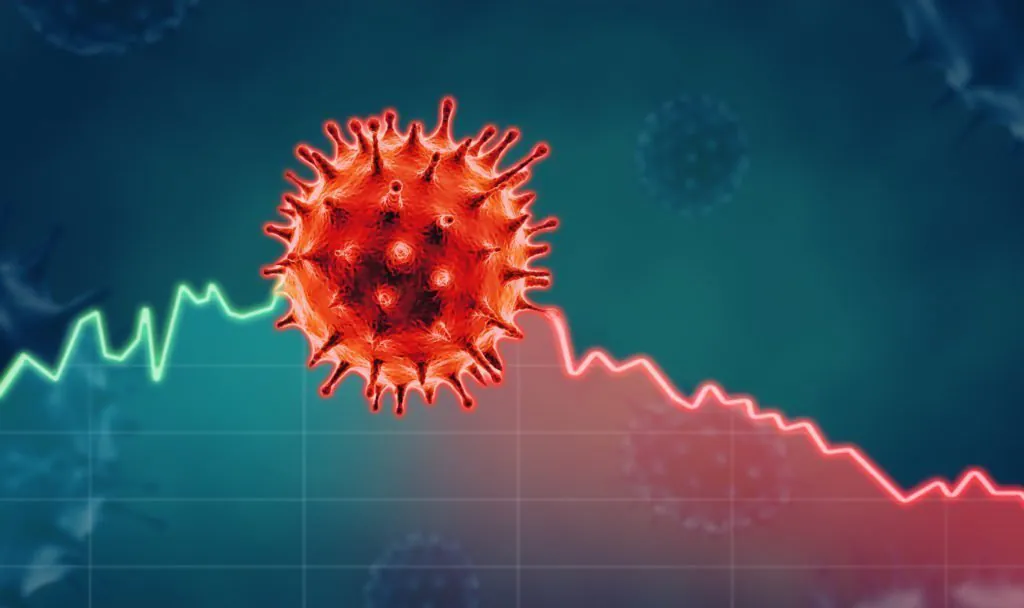
For humans, the unpredictability of life is an existential concern. It’s a similar threat for analytical models. Like us, algorithms attempt to predict the future by generalizing past events. The more random the history, the more we both struggle to create a pattern out of the chaos.
A month ago we thought COVID might be over. Now, with the emergence of the Delta variant, the economy is in a strange dance between open and closed. And if we wanted to find out what’s next — say, if we fed a predictive model a summary of the past year and a half — it very well might tell us that we’re headed for another lockdown.
Data, like food or fashion trends, expire. If you only supply your models with limited historical data, they can’t paint a very accurate picture of what’s going on in the market today, much less what will happen tomorrow.
When the reality of daily life changes by the week and even by the day, you need to refresh your models, constantly, with new data assets and new dimensions that provide insights into the current context.
When reality changes, you need to change with it
Every fluctuation in public safety guidelines has sparked a remarkable shift in consumer behavior. Unfortunately, each of those shifts in consumer behavior rendered large portions of organizations’ analytical models obsolete.
Now think about the companies that, despite the constant change, have maintained and even grew their business during the pandemic. They acted quickly to find the right data sets that could give them visibility into customer’s changing habits. The companies that tried to stick with their same old data sources, even though the world had changed completely, lost their share of the market.
The retail industry, for example, had to embrace the massive transition to online shopping at the start of the pandemic, the complexity of online-offline combination transactions (like curbside pickup) and the revival of in-person experiences as COVID restrictions have lifted.
Savvy retail companies sought alternative data sources to provide the proper context with each step in the process. At first, they looked for COVID-related data sets — which included CDC data about the spread of the disease, for example — to adjust their sales forecasting models. Then, they reviewed foot traffic vs. online traffic to determine their approach to reopening. As the pandemic wore on, retail companies examined sales and credit card spending data against demographic attributes to understand how their typical customer profile had changed.
Data deterioration déjà vu
As much as we’re all looking forward to the reopening (whenever it comes), this dramatic societal change forewarns a similar outcome as the first global shutdown. Organizations that have trained their analytics models using data sets related to the pandemic-era market will once again have to seek out new sources.
But unlike the first lockdown, this time we have the opportunity to prepare for the significant change when it comes. We know we’ll need to find alternate data sources and create models that can make sense of the new reality.
So, what should you do when you know your data will expire? First, look for ways to broaden your data sources outside of your historical data. The organizations with the most powerful analytical programs treat data acquisition the same way they treat customer acquisition — they build a scalable approach for continuously discovering, vetting and onboarding new data assets. This approach boils down to three components: people, systems and processes, in that order.
People
There’s a lot of data sources out there. How do you know that the ones you’re using are working? How do you know what you’re missing? By building a team dedicated to acquiring the right data, working with vendors to do so and managing your own data assets. Roles to hire include a Head of Data Acquisition, Data Architects, Data Strategist and Data Scientists.
Systems
Remember how some companies struggled with the transition to remote work because they didn’t have centralized IT? It’s a lesson we still need to be reminded of when it comes to analytical models. I’ve seen big organizations buying and integrating the same data two or three times at different stages. A centralized place where different sides of the business can collaborate on data sets entering the organization is necessary.
Even more importantly, your data acquisition ecosystem should allow you to iterate on a variety of data and accelerate your PLCs, tests and business initiatives. Within a few days, you should be able to not just catalog new data sources but deploy them to production. Time is everything when reality changes so quickly.
Processes
Speaking of time, if it takes your organization two or three months to examine a single data source (much less integrate it) that’s a sign you’re not very well prepared to absorb new data. Create a workflow for the lifecycle of data acquisition, from testing to buying to integration. This process should involve a cross-functional partnership consisting of IT, procurement, legal, compliance and security so each team can address the respective sourcing issues.
Conclusion
Smart data leaders know data sources are a competitive asset to reacting quickly to market conditions. Once you’ve invested in a data acquisition strategy, it doesn’t matter whether we go back into lockdown or rebound quickly. You’ll be ready to land on your feet amid any major disruption.
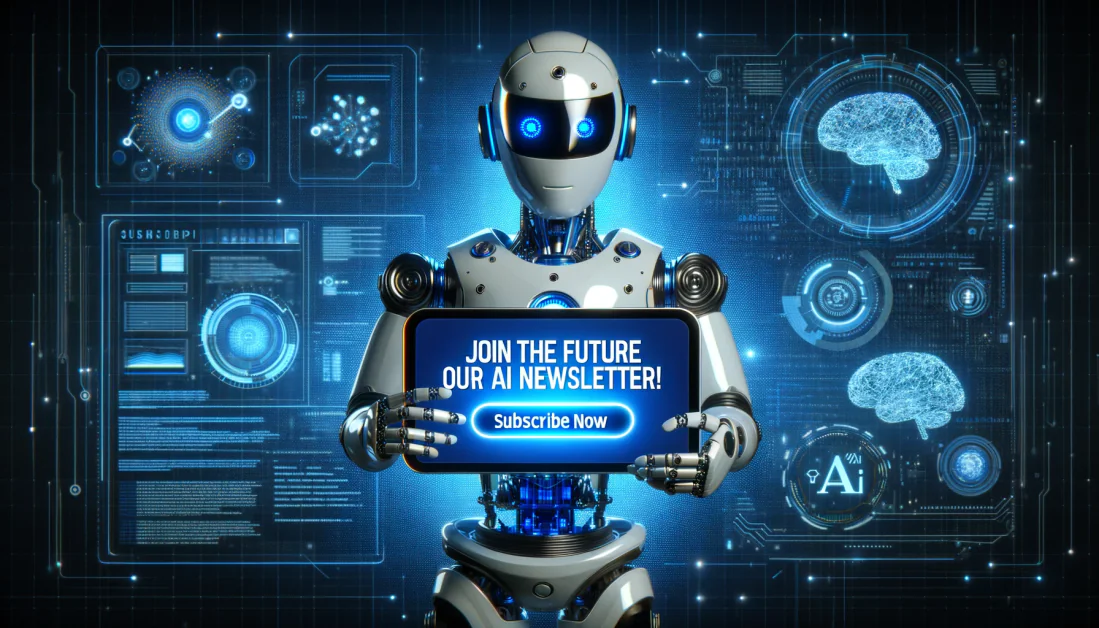
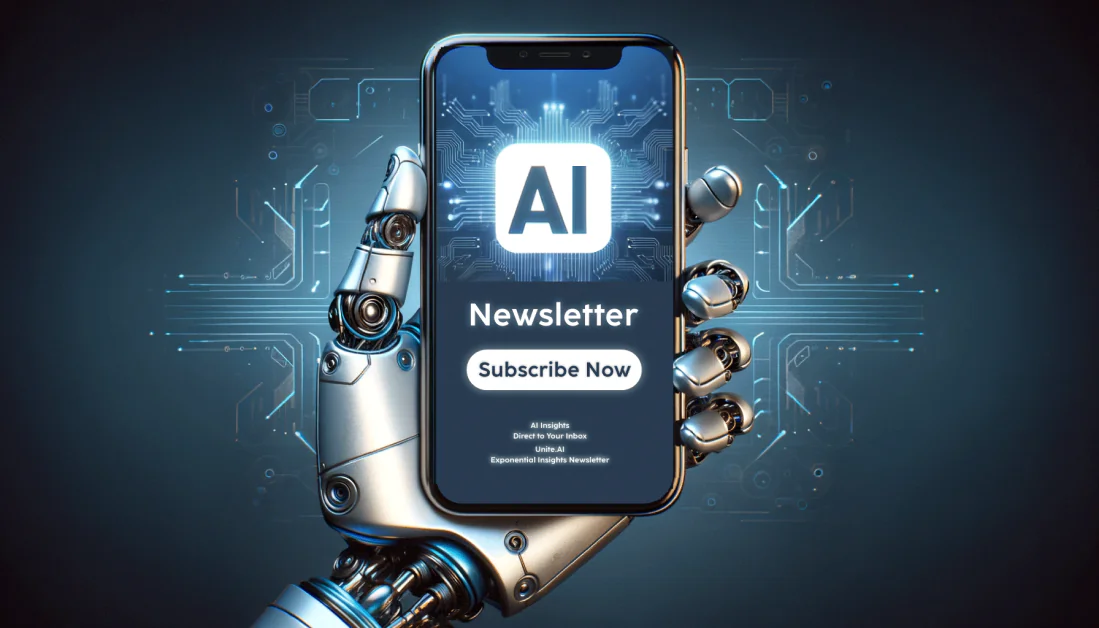