Robotics
Unity Advances Robotics Industry With New Releases
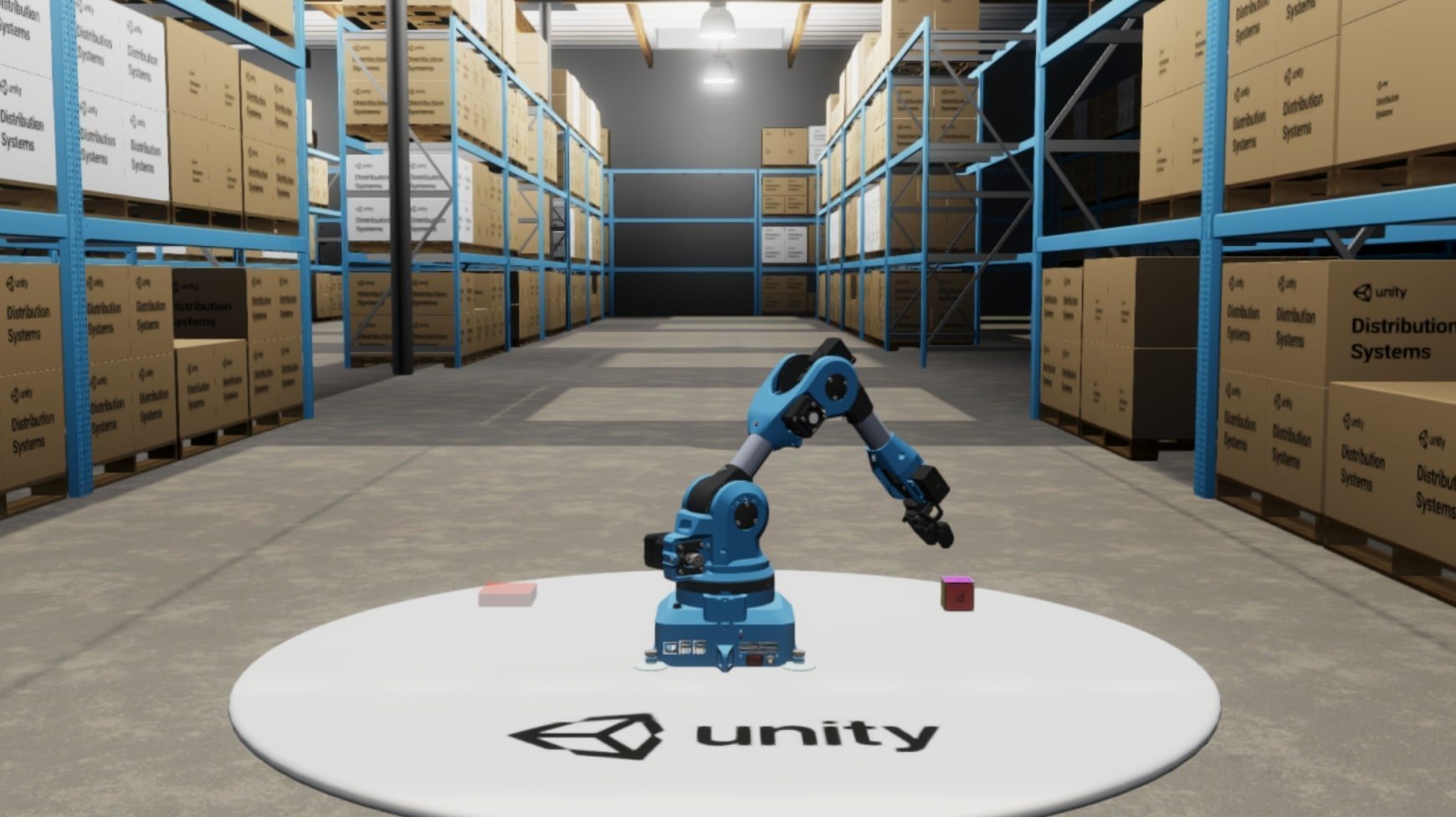
Unity is one of the leading platforms for developing and operating real-time 3D, or RT3D, content. The company has recently announced its Object Pose Estimation, which aims to enhance the Robotics Industry, specifically in the industrial setting, through the use of computer vision and simulation technologies.
Object Pose Demonstration
The Object Pose Demonstration took place alongside a corresponding demonstration, showing how robots can learn through synthetic data.
Dr. Danny Lange is Senior Vice President of Artificial Intelligence at Unity.
“This is a powerful example of a system that learns instead of being programmed, and as it learns from the synthetic data, it is able to capture much more nuanced patterns than any programmer ever could,” he said. “Layering our technologies together shows how we are crossing a line, and we are starting to deal with something that is truly AI, and in this case, demonstrating the efficiencies possible in training robots.”
When Dr. Lange refers to layering the company's technologies, he is partly referring to Unity's recent releases that support the Robot Operating System (ROS), which is a flexible framework for developing robot software.
Building on Previous Releases
Prior to the release of the Object Pose Estimation demo, Unity released the company’s URDF Importer, an open-source Unity package, along with the ROS-TCP-Connector, which aims to drastically reduce the latency of messages between ROS nodes and Unity. This enables the robot operating in a simulated environment to act in near real-time.
Simulation technology is often relied on when testing applications in dangerous, expensive, or rare situations. By using simulation, the applications can be validated before deploying to the robot, which enables early detection of potential problems. By combining Unity's built-in physics engine and the Unity Editor, there can be an endless number of virtual environments.
With the combination of these tools, the demonstration showed how large amounts of synthetic, labeled training data can be created. It was then used to train a simple deep learning model to predict the position of a cube. The demo provided a tutorial for those looking to recreate the project.
“With Unity, we have not only democratized data creation, we’ve also provided access to an interactive system for simulating advanced interactions in a virtual setting,” Lange continued.
“You can develop the control systems for an autonomous vehicle, for example, or here for highly expensive robotic arms, without the risk of damaging equipment or dramatically increasing cost of industrial installations. To be able to prove the risk of intended applications in a high-fidelity virtual environment will save time and money for many industries poised to be transformed by robotics combined with AI and Machine Learning.”
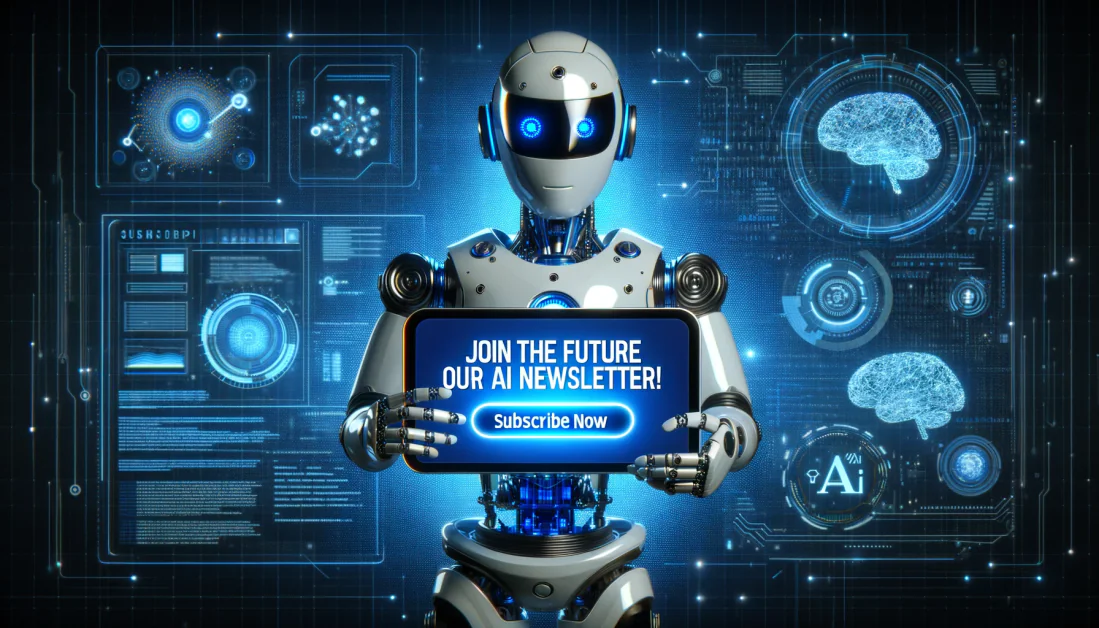
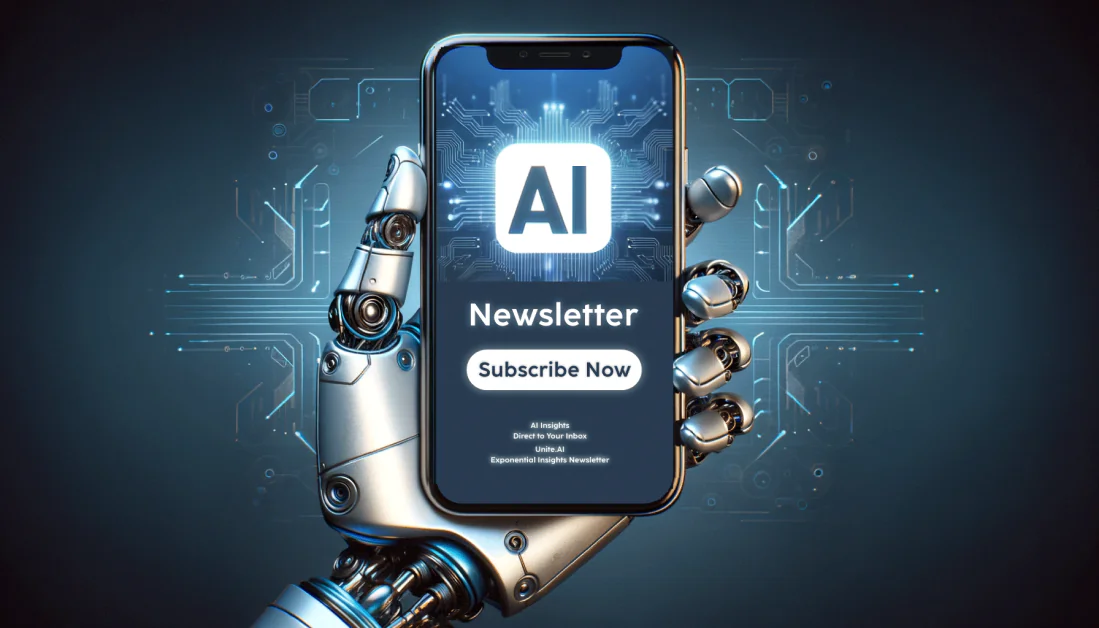