Artificial Intelligence
Top 10 LLM Vulnerabilities
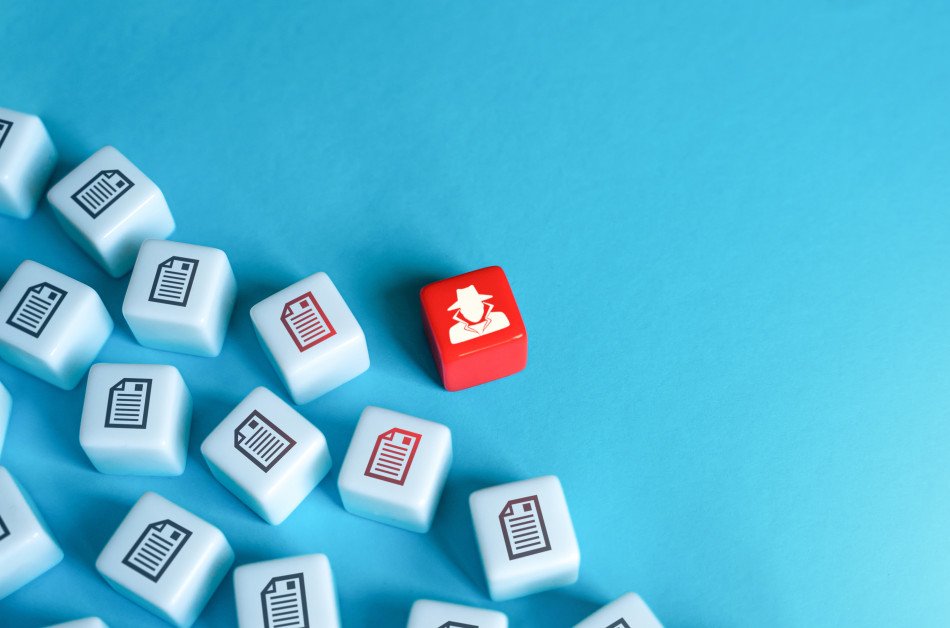
In artificial intelligence (AI), the power and potential of Large Language Models (LLMs) are undeniable, especially after OpenAI’s groundbreaking releases such as ChatGPT and GPT-4. Today, there are numerous proprietary and open-source LLMs in the market that are revolutionizing industries and bringing transformative changes in how businesses function. Despite rapid transformation, there are numerous LLM vulnerabilities and shortcomings that must be addressed.
For instance, LLMs can be used to conduct cyberattacks like spear phishing by generating human-like personalized spear phishing messages in bulk. Latest research shows how easy it is to create unique spear phishing messages using OpenAI’s GPT models by crafting basic prompts. If left unaddressed, LLM vulnerabilities could compromise the applicability of LLMs on an enterprise scale.
An illustration of an LLM-based spear phishing attack
In this article, we’ll address major LLM vulnerabilities and discuss how organizations could overcome these issues.
Top 10 LLM Vulnerabilities & How to Mitigate Them
As the power of LLMs continues to ignite innovation, it is important to understand the vulnerabilities of these cutting-edge technologies. The following are the top 10 vulnerabilities associated with LLMs and the steps required to address each challenge.
1. Training Data Poisoning
LLM performance is heavily reliant on the quality of training data. Malicious actors can manipulate this data, introducing bias or misinformation to compromise outputs.
Solution
To mitigate this vulnerability, rigorous data curation and validation processes are essential. Regular audits and diversity checks in the training data can help identify and rectify potential issues.
2. Unauthorized Code Execution
LLMs' ability to generate code introduces a vector for unauthorized access and manipulation. Malicious actors can inject harmful code, undermining the model’s security.
Solution
Employing rigorous input validation, content filtering, and sandboxing techniques can counteract this threat, ensuring code safety.
3. Prompt Injection
Manipulating LLMs through deceptive prompts can lead to unintended outputs, facilitating the spread of misinformation. By developing prompts that exploit the model's biases or limitations, attackers can coax the AI into generating inaccurate content that aligns with their agenda.
Solution
Establishing predefined guidelines for prompt usage and refining prompt engineering techniques can help curtail this LLM vulnerability. Additionally, fine-tuning models to align better with desired behavior can enhance response accuracy.
4. Server-Side Request Forgery (SSRF) Vulnerabilities
LLMs inadvertently create openings for Server-Side Request Forgery (SSRF) attacks, which enable threat actors to manipulate internal resources, including APIs and databases. This exploitation exposes the LLM to unauthorized prompt initiation and the extraction of confidential internal resources. Such attacks circumvent security measures, posing threats like data leaks and unauthorized system access.
Solution
Integrating input sanitization and monitoring network interactions prevents SSRF-based exploits, bolstering overall system security.
5. Overreliance on LLM-generated Content
Excessive reliance on LLM-generated content without fact-checking can lead to the propagation of inaccurate or fabricated information. Also, LLMs tend to “hallucinate,” generating plausible yet entirely fictional information. Users may mistakenly assume the content is reliable due to its coherent appearance, increasing the risk of misinformation.
Solution
Incorporating human oversight for content validation and fact-checking ensures higher content accuracy and upholds credibility.
6. Inadequate AI Alignment
Inadequate alignment refers to situations where the model's behavior doesn't align with human values or intentions. This can result in LLMs generating offensive, inappropriate, or harmful outputs, potentially causing reputational damage or fostering discord.
Solution
Implementing reinforcement learning strategies to align AI behaviors with human values curbs discrepancies, fostering ethical AI interactions.
7. Inadequate Sandboxing
Sandboxing involves restricting LLM capabilities to prevent unauthorized actions. Inadequate sandboxing can expose systems to risks like executing malicious code or unauthorized data access, as the model may exceed its intended boundaries.
Solution
For ensuring system integrity, forming a defense against potential breaches is crucial which involves robust sandboxing, instance isolation, and securing server infrastructure.
8. Improper Error Handling
Poorly managed errors can divulge sensitive information about the LLM's architecture or behavior, which attackers could exploit to gain access or devise more effective attacks. Proper error handling is essential to prevent inadvertent disclosure of information that could aid threat actors.
Solution
Building comprehensive error-handling mechanisms that proactively manage various inputs can enhance the overall reliability and user experience of LLM-based systems.
9. Model Theft
Due to their financial value, LLMs can be attractive targets for theft. Threat actors can steal or leak code base and replicate or use it for malicious purposes.
Solution
Organizations can employ encryption, stringent access controls, and constant monitoring safeguards against model theft attempts to preserve model integrity.
10. Insufficient Access Control
Insufficient access control mechanisms expose LLMs to the risk of unauthorized usage, granting malicious actors opportunities to exploit or abuse the model for their ill purposes. Without robust access controls, these actors can manipulate LLM-generated content, compromise its reliability, or even extract sensitive data.
Solution
Strong access controls prevent unauthorized usage, tampering, or data breaches. Stringent access protocols, user authentication, and vigilant auditing deter unauthorized access, enhancing overall security.
Ethical Considerations in LLM Vulnerabilities
The exploitation of LLM vulnerabilities carries far-reaching consequences. From spreading misinformation to facilitating unauthorized access, the fallout from these vulnerabilities underscores the critical need for responsible AI development.
Developers, researchers, and policymakers must collaborate to establish robust safeguards against potential harm. Moreover, addressing biases ingrained in training data and mitigating unintended outcomes must be prioritized.
As LLMs become increasingly embedded in our lives, ethical considerations must guide their evolution, ensuring that technology benefits society without compromising integrity.
As we explore the landscape of LLM vulnerabilities, it becomes evident that innovation comes with responsibility. By embracing responsible AI and ethical oversight, we can pave the way for an AI-empowered society.
Want to enhance your AI IQ? Navigate through Unite.ai‘s extensive catalog of insightful AI resources to amplify your knowledge.
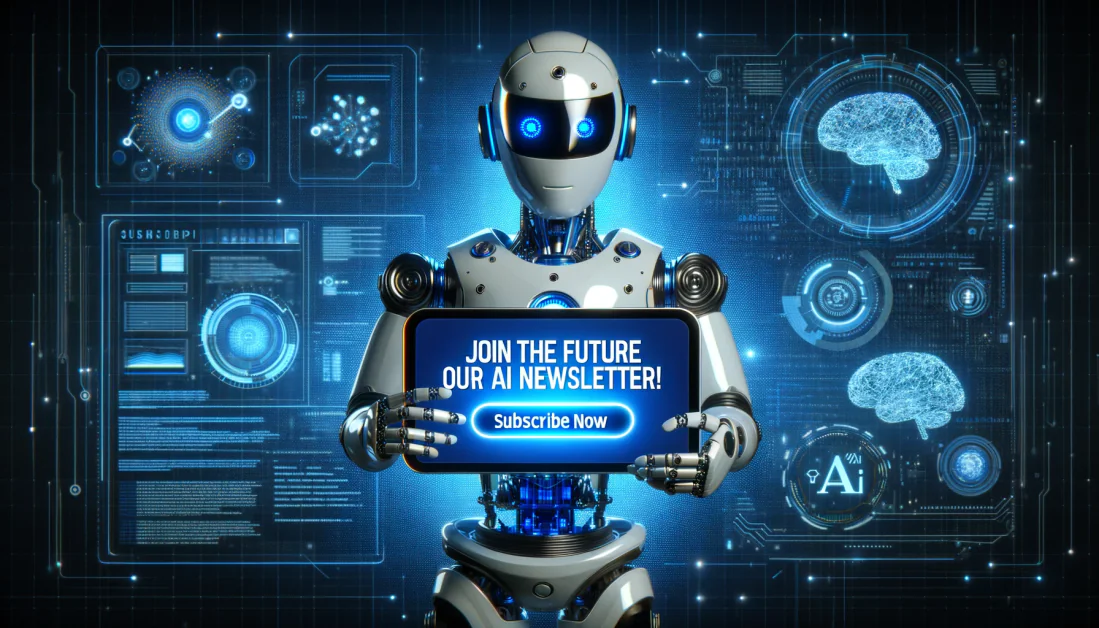
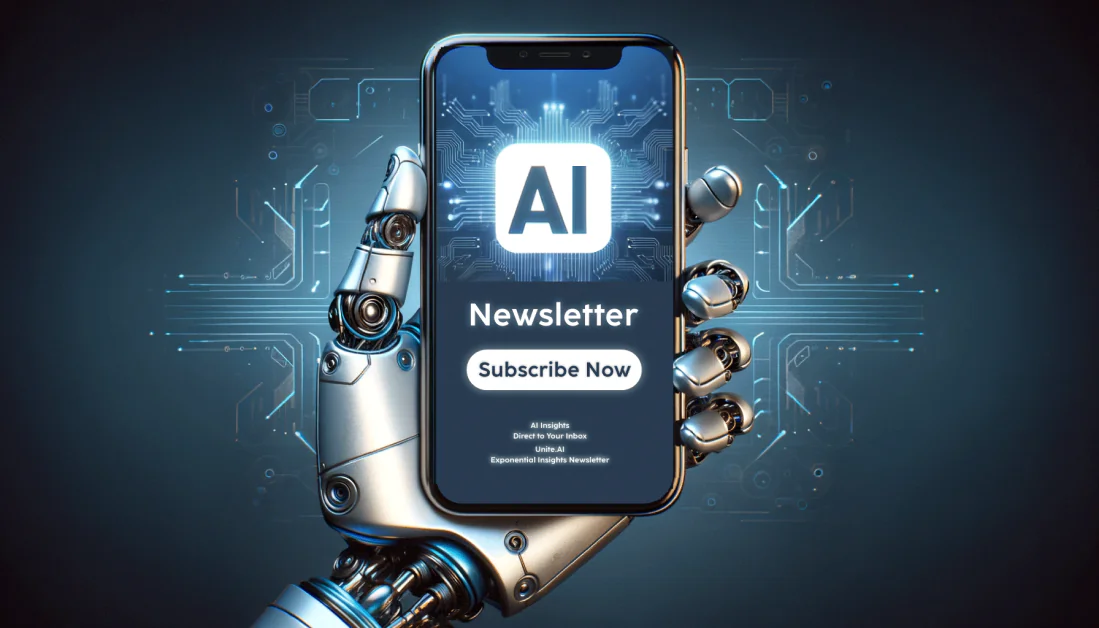