Artificial Intelligence
The Future of Speech Scoring – Thought Leaders
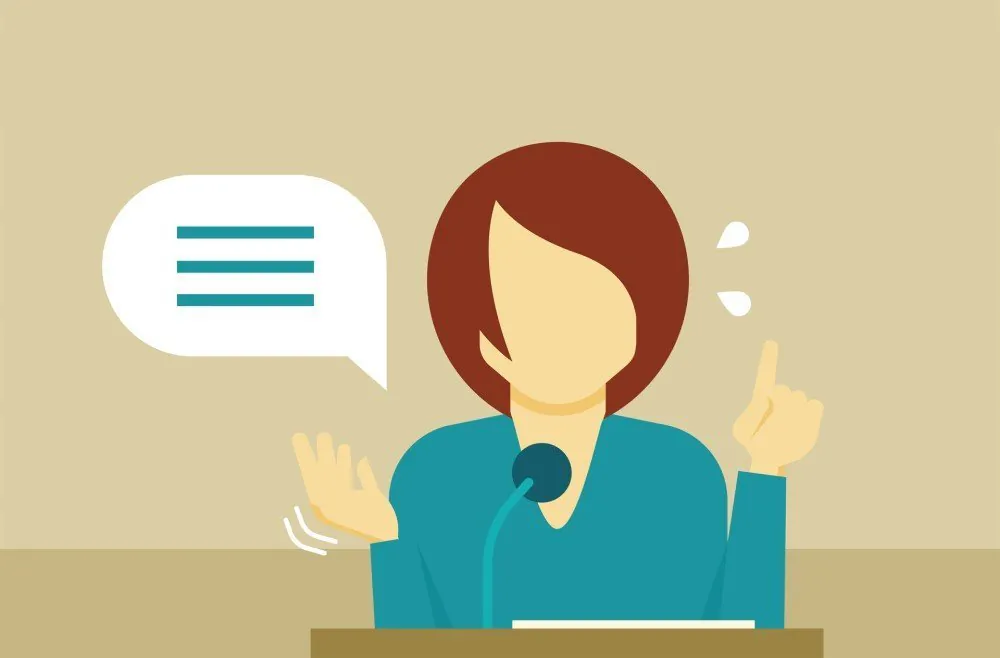
Across the world, the number of English language learners continues to rise. Educational institutions and employers need to be able to assess the English proficiency of language learners – in particular, their speaking ability, since spoken language remains among the most essential language abilities. The challenge, for both assessment developers and end users, is finding a way to do so that is accurate, fast and financially viable. As part of this challenge, scoring these assessments comes with its own set of factors, especially when we consider the different areas (speech, writing, etc.) one is being tested on. With the demand for English-language skills across the globe only expected to increase, what would the future of speech scoring need to look like in order to meet these needs?
The answer to that question, in part, is found in the evolution of speech scoring to date. Rating constructed spoken responses has historically been done using human raters. This process, however, tends to be expensive and slow, and has additional challenges including scalability and various shortcomings of human raters themselves (e.g., rater subjectivity or bias). As discussed in our book Automated Speaking Assessment: Using Language Technologies to Score Spontaneous Speech, in order to address these challenges, an increasing number of assessments now make use of automated speech scoring technology as the sole source of scoring or in combination with human raters. Before deploying automated scoring engines, however, their performance needs to be thoroughly evaluated, particularly in relation to the score reliability, validity (does the system measure what it is supposed to?) and fairness (i.e., the system should not introduce bias related to population subgroups such as gender or native language).
Since 2006, ETS’s own speech scoring engine, SpeechRater®, has been operationalized in the TOEFL® Practice Online (TPO) assessment (used by prospective test takers to prepare for the TOEFL iBT® assessment), and since 2019, SpeechRater has also been used, along with human raters, for scoring the speaking section of the TOEFL iBT® assessment. The engine evaluates a wide range of speaking proficiency for spontaneous non-native speech, including pronunciation and fluency, vocabulary range and grammar, and higher-level speaking abilities related to coherence and progression of ideas. These features are computed by using natural language processing (NLP) and speech processing algorithms. A statistical model is then applied to these features in order to assign a final score to a test taker’s response.
While this model is trained on previously observed data scored by human raters, it is also reviewed by content experts to maximize its validity. If a response is found to be non-scorable due to audio quality or other issues, the engine can flag it for further review to avoid generating a potentially unreliable or invalid score. Human raters are always involved in the scoring of spoken responses in the high-stakes TOEFL iBT speaking assessment.
As human raters and SpeechRater are currently used together to score test takers’ responses in high-stakes speaking assessments, both play a part in what the future of scoring English language proficiency can be. Human raters have the ability to understand the content and discourse organization of a spoken response in a deep way. In contrast, automated speech scoring engines can more precisely measure certain detailed aspects of speech, such as fluency or pronunciation, exhibit perfect consistency over time, can reduce overall scoring time and cost, and are more easily scaled to support large testing volumes. When human raters and automated speech scoring systems are combined, the resulting system can benefit from the strengths of each scoring approach.
In order to continuously evolve automated speech scoring engines, research and development needs to focus on the following aspects, among others:
- Building automatic speech recognition systems with higher accuracy: Since most features of a speech scoring system rely directly or indirectly on this component of the system that converts the test taker’s speech to a text transcription, highly accurate automatic speech recognition is essential for obtaining valid features;
- Exploration of new ways to combine human and automated scores: In order to take full advantage of the respective strengths of human rater scores and automated engine scores, more ways of combining this evidence need to be explored;
- Accounting for abnormalities in responses, both technical and behavioral: High-performing filters capable of flagging such responses and excluding them from automated scoring are necessary to help ensure the validity and reliability of the resulting assessment scores;
- Assessment of spontaneous or conversational speech that occurs most often in day-to-day life: While automated scoring of such interactive speech is an important goal, these items present numerous scoring challenges, including overall evaluation and scoring;
- Exploring deep learning technologies for automated speech scoring: This relatively recent paradigm within machine learning has produced substantial performance increases on many artificial intelligence (AI) tasks in recent years (e.g., automatic speech recognition, image recognition), and therefore it is likely that automated scoring also may benefit from using this technology. However, since most of these systems can be considered “black-box” approaches, attention to the interpretability of the resulting score will be important to maintain some level of transparency.
To accommodate a growing and changing English-language learner population, next-generation speech scoring systems must expand automation and the range of what they are able to measure, enabling consistency and scalability. That is not to say the human element will be removed, especially for high-stakes assessments. Human raters will likely remain essential for capturing certain aspects of speech that will remain hard to evaluate accurately by automated scoring systems for a while to come, including the detailed aspects of spoken content and discourse. Using automated speech scoring systems in isolation for consequential assessments also runs the risk of not identifying problematic responses by test takers— for instance, responses that are off-topic or plagiarized, and, as a consequence, can lead to reduced validity and reliability. Using both human raters and automated scoring systems in combination may be the best way for scoring speech in high-stakes assessments for the foreseeable future, particularly if spontaneous or conversational speech is evaluated.
Written by: Keelan Evanini, Director of Speech Research, ETS & Klaus Zechner, Managing Senior Research Scientist, Speech, ETS
ETS works with education institutions, businesses and governments to conduct research and develop assessment programs that provide meaningful information they can count on to evaluate people and programs. ETS develops, administers and scores more than 50 million tests annually in more than 180 countries at more than 9,000 locations worldwide. We design our assessments with industry-leading insight, rigorous research and an uncompromising commitment to quality so that we can help education and workplace communities make informed decisions. To learn more visit ETS.
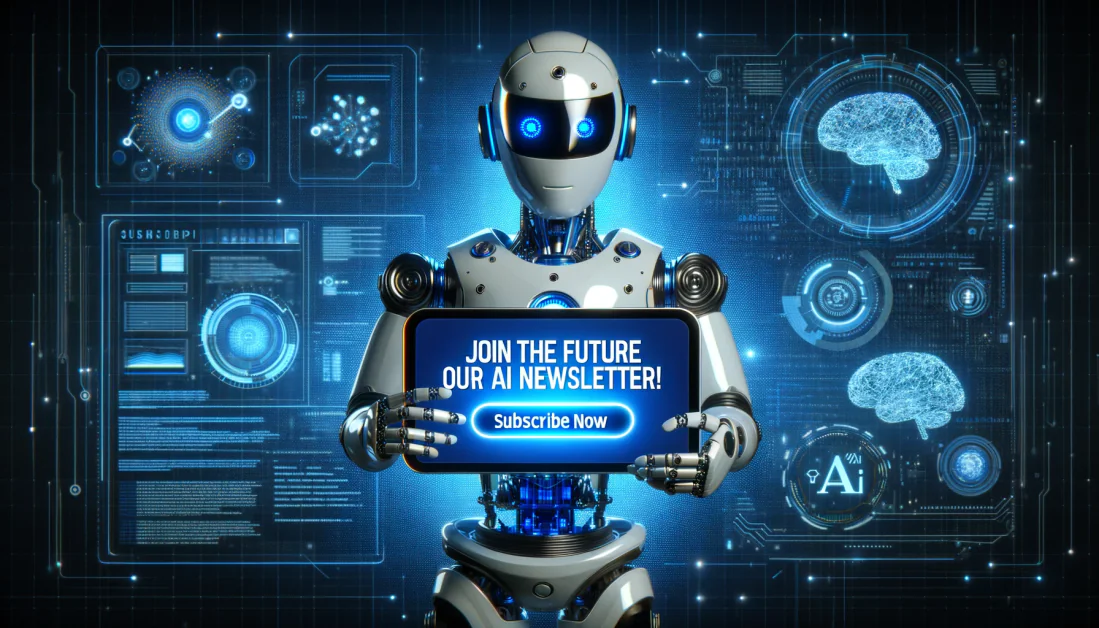
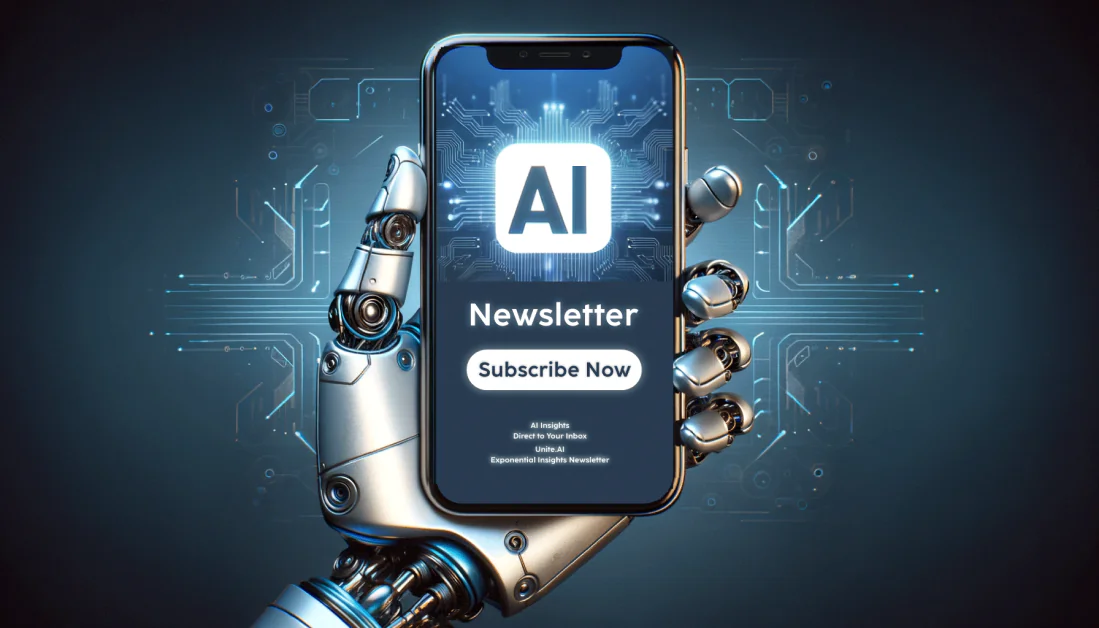