Ethics
Researchers Develop New Tool to Fight Bias in Computer Vision
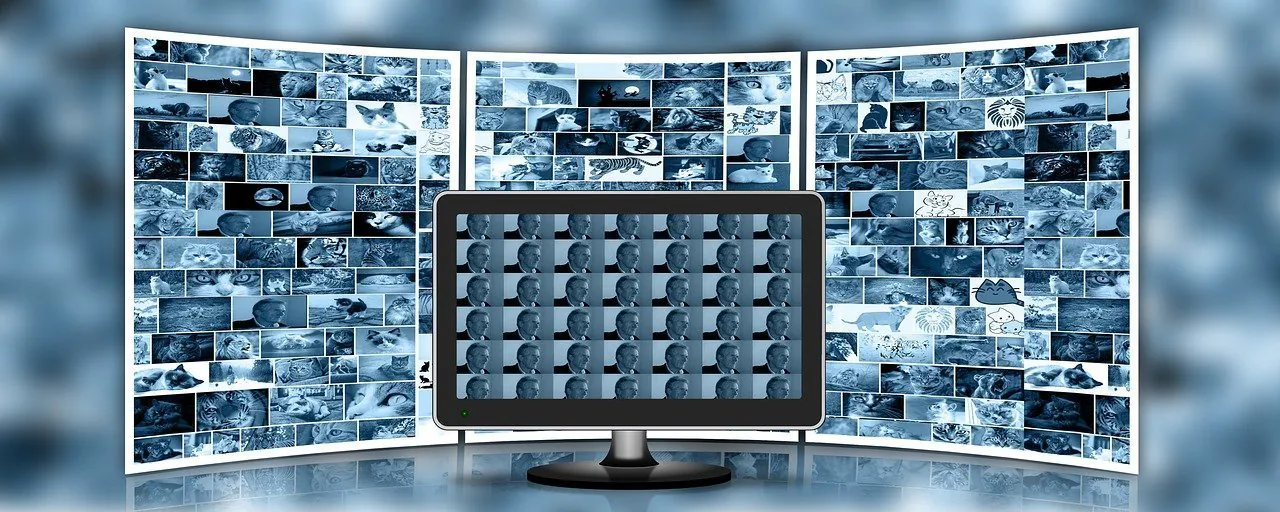
One of the recent issues that has emerged within the field of artificial intelligence (AI) is that of bias in computer vision. Many experts are now discovering bias within AI systems, leading to skewed results in various different applications, such as courtroom sentencing programs.
There is a large effort going forward attempting to fix some of these issues, with the newest development coming from Princeton University. Researchers at the institution have created a new tool that is able to flag potential biases in images that are used to train AI systems.
The work was presented on Aug. 24 at the virtual European Conference on Computer Vision.
Bias in AI Systems
One of the major reasons for the bias present in current AI systems is that they are often trained on large sets of images coming from online sources. These images can be stereotypical, and when they go toward developing computer vision, the result can be unintentionally influenced models. Computer vision is what enables computers to identify people, objects and actions.
The tool that was developed by the researchers is open-source, and it is capable of automatically revealing potential biases in visual data sets. It works by taking action before the image sets are used to train the computer vision models, and issues surrounding underrepresentation and stereotypes can be remedied before they cause an effect.
REVISE
The new tool is called REVISE, and it relies on statistical methods to identify potential biases in a data set. It focuses on the three areas of object-based, gender-based and geography-based.
REVISE is fully automatic and was built upon previous methods that included filtering and balancing data set images so that the user could have more control.
The new tool relies on existing image annotations and measurements to analyze the content within a data set. Some of those existing annotations include object count and countries of origin for the images.
In one example of the tool working, REVISE showed how images of both people and flowers were different depending on gender. Males were more likely to appear with flowers in ceremonies or meetings, and females were more likely to appear with flowers in paintings or staged scenarios.
Olga Russaskovsky is an assistant professor of computer science and principal investigator of the Visual AI Lab. The paper was co-authored with graduate student Angelina Wang and associate professor of computer science, Arvind Narayanan.
After the tool identifies discrepancies, “then there’s the question of whether this is a totally innocuous fact, or something deeper is happening, and that’s very hard to automate,” Russaskovsky said.
Underrepresented or Misrepresented Regions
Various regions around the world are underrepresented in computer vision data sets, and this can lead to bias in AI systems. One of the findings was that a dramatically larger amount of images come from the United States and European countries. REVISE also revealed that images from other parts of the world often do not have image captions in the local language, meaning many could come from a tourist’s view of a nation.
“…this geography analysis shows that object recognition can still be quite biased and exclusionary, and can affect different regions and people unequally,” Russaskovsky continued.
“Data set collection practices in computer science haven't been scrutinized that thoroughly until recently,” said Wang. When it comes to image collection, they are “scraped from the internet, and people don't always realize that their images are being used [in data sets]. We should collect images from more diverse groups of people, but when we do, we should be careful that we're getting the images in a way that is respectful.”
Vicente Ordonez-Roman is an assistant professor of computer science at the University of Virginia.
“Tools and benchmarks are an important step … they allow us to capture these biases earlier in the pipeline and rethink our problem setup and assumptions as well as data collection practices,” said Ordonez-Roman. “In computer vision there are some specific challenges regarding representation and the propagation of stereotypes. Works such as those by the Princeton Visual AI Lab help elucidate and bring to the attention of the computer vision community some of these issues and offer strategies to mitigate them.”
The new tool developed by the researchers is an important step to help remedy the bias present in AI systems. Now is the time to fix these issues, as it will become much more difficult as the systems progress and get more complex.
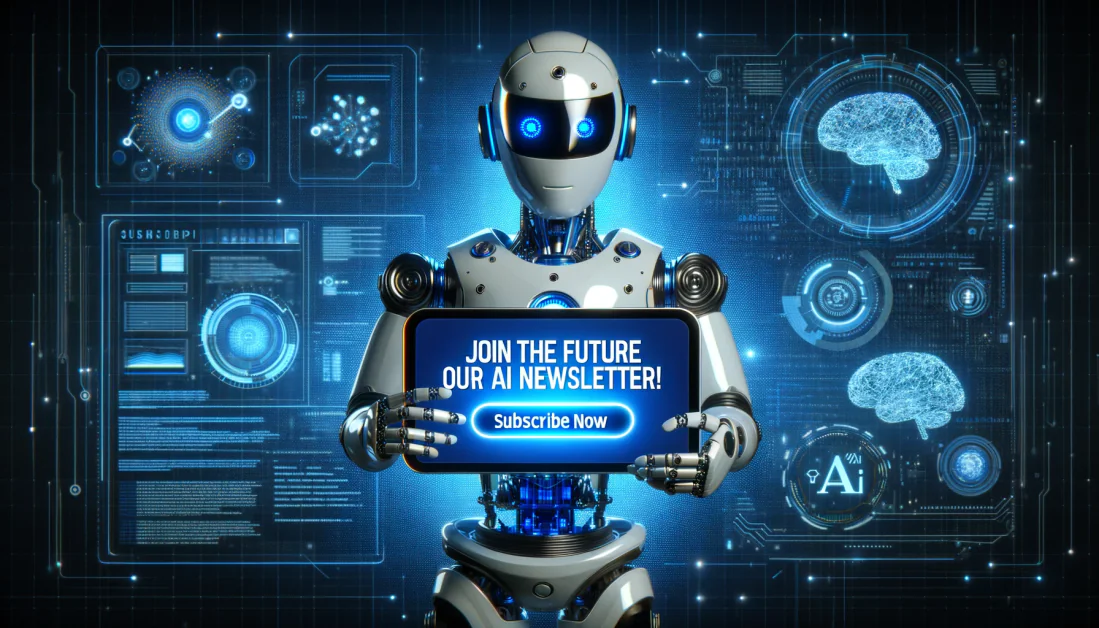
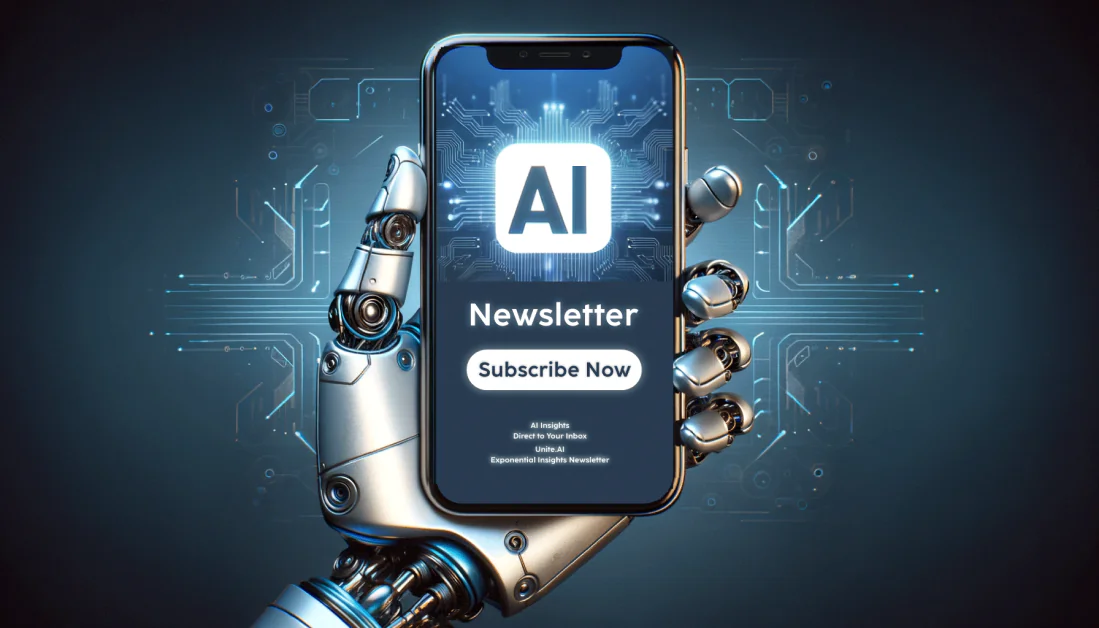