Interviews
Nikola Mrksic, Co-founder and CEO of PolyAI – Interview Series
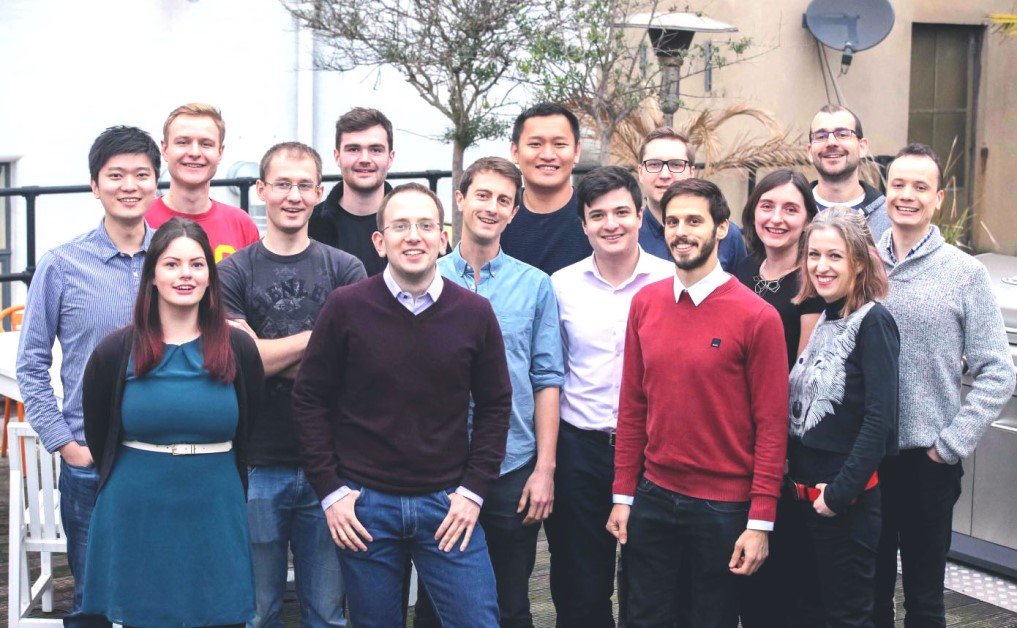
Nikola Mrksic is co-founder and CEO of PolyAI, a leading supplier of enterprise-ready voice assistants for automated customer service.
What initially attracted you to AI?
I've been into maths and computer science from a very early age. During my studies at Cambridge, I got the chance to work with several leading machine learning researchers, including Steve Young and Zoubin Ghahramani. Steve convinced me to join his startup, VocalIQ, to work on building spoken dialogue systems. Later, I ended up doing a PhD with Steve as well, working on building data driven language understanding models that work across different use cases and languages. Conversational AI is a really hard, complex field of work, with many scientific and engineering breakthroughs still ahead of us, and it's kept me busy ever since.
In 2017, you launched PolyAI a conversational AI company, could you discuss the genesis story behind PolyAI?
My co-founders, Shawn Wen, Eddy Su and I did our PhDs at Cambridge at the same time. We had worked on dialogue systems for years, but we soon realized that the kinds of sophisticated systems we were used to working on had very few commercial applications. So we came together to create a conversational AI solution that would be beneficial in the real world. We saw an opportunity for truly conversational, multi-turn, transactional dialogue systems that could interact with real people in everyday life.
We focused on customer service as we felt current technological capabilities and requirements of customers were well matched.
Could you discuss some of the machine learning and natural language processing technologies that are used?
Our main secret sauce is our set of different proprietary encoder models. Weāve pre-trained them on billions of natural conversations, so they can extract intent even when the input speech uses slang or idioms for example. This is incredibly important for communicating over the phone. Customers donāt speak in keywords; they tell stories, interrupt, ask questions and generally just want to take control of the conversation.
Weāve recently announced our ConVEx model, an extremely data-efficient entity extractor, which allows us to accurately extract values from conversations.
Our ASR orchestration process involves using fine tuning speech recognition platforms to neutralize the noise caused by different accents, as well as fine-tuning for different contexts.
Weāve also developed a pretty robust dialogue policy library with pre-designed use cases that include all the common customer service transactions, so we can spin up a new voice assistant for clients extremely quickly.
In your opinion what differentiates a good conversational AI product with a poor conversational AI?
A good product will consistently understand what users mean and will never make users repeat themselves. Calls often happen in noisy environments, so products need to be resilient to messy inputs. As brands reach out to large markets, products need to understand a variety of accents and ways of phrasing intents. Both of these require products to guarantee robust speech recognition capabilities, resilient intent classification and entity extraction.
A great product will be actively engaging for users. It will follow the userās train of thoughts, and be able to handle complex, every-day cases where users may be sharing multiple intentions and pieces of information simultaneously, and they may jump between different contexts. That requires robust multi-label classification and context management.
An engaging product will display human characteristics without being uncanny or too robotic. This means snappy interactions, genuine voices, continuous feedback cues and a degree of randomness and imperfections.
Finally, a great conversational AI product will engage with users wherever they are and offer a seamless, platform-specific experience, which may span across voice, SMS, chat or social messaging platforms. The interaction paradigm should embrace each communication platformās specificity.
What are some of the advantages of companies using conversational AI instead of attempting to funnel inquiries to chat bots?
Customer experience is critical and has become a key driver for retention. The top priority should be making it easy for customers to do what they need to do.
The phone is still most customersā preferred channel for contacting a company. Up to 65% of all customer interactions still happen over the phone. During the COVID-19 pandemic, contact centers have been pushed to the extremes with more customers than ever calling for support.
Of course, a great experience allows customers to communicate however they like, so for anyone who prefers asynchronous communications, we make it simple for brands to offer the same level of experience across textual channels.
How much of a challenge is detecting the intent of what a customer is trying to say?
Thereās a number of challenges with understanding customers through voice channels. Accurately and consistently understanding usersā meaning requires numerous components to work well together.
First, speech recognition is difficult, especially when people are calling from noisy environments like when theyāre on speakerphone, or when driving through traffic or tunnels. Speech recognition can also be difficult in regions with different accents and dialects. Weāve developed an effective way to bias speech recognition models for the given context in order to optimize speech recognition.
Because our ConveRT model has been trained on such a huge amount of conversational data, itās able to detect intent on weak signals, just like us humans can generally understand what someone says, even if we miss a word or two.
Another consideration is understanding when users want to take on several actions at once. For example, someone might say, āI lost my card. Can you let me know if itās been used and block it?ā. In this instance, the model needs to recognize two intents and act on them in an order that makes sense.
The model also needs to be able to extract and understand the entities being volunteered by customers. For example, ādo you have a table Saturday lunch for me, my wife and our 2 kids?ā The surface level intention here is checking availability for a table, but the model needs to pick out the date (Saturday) and the number of people (4) and any other potential information that may be relevant (perhaps children are only allowed in the restaurant area, and canāt be seated at the bar).
Finally, conversation is not always linear. Customers may interrupt with questions unrelated to the voice assistantās prompt, so the assistant needs to be able to ālisten outā for one type of input, while being open to different triggers such as FAQs or changes to information previously provided by the user.
Whatās the process and timeline required for a company that wants to launch a conversational AI bot with PolyAI?
Weāre here to provide voice assistants that have tangible business impact. So we start every engagement with a discovery where we help clients to identify and articulate their CX objectives, key metrics and support processes. This is where we scope out the journeys the voice assistant will need to guide customers through. This, plus our pre trained ConveRT model, means we donāt need huge amounts of conversational data from clients.
From there, weāre able to develop a voice assistant with very little input needed from the client, so itās not at all demanding on in-house IT teams.
Depending on complexity, we can spin up a proof of value in as little as 2 weeks, and a fully-fledged deployment in 2 months.
Thank you for the great interview, readers who wish to learn more should visit PolyAI.