Brain Machine Interface
Newly Published Research Will Drastically Stabilize Brain-Computer Interfaces
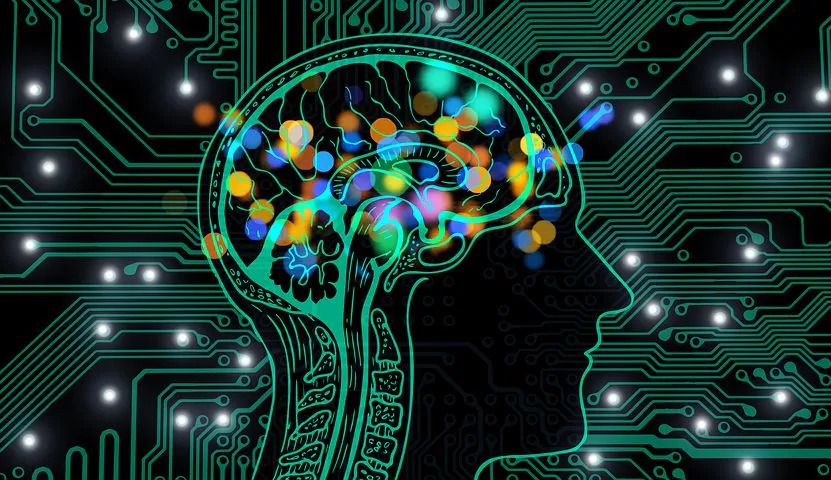
New research coming out of Carnegie Mellon University (CMU) and the University of Pittsburgh (Pitt) will drastically improve and stabilize brain-computer interfaces.
The research was published in Nature Biomedical Engineering, with the paper being titled “A stabilized brain-computer interface based on neural manifold alignment.”
Brain-Computer Interfaces (BCI)
Brain-computer interfaces (BCI) are devices capable of allowing disabled individuals to control prosthetic limbs, computer curses, or other interfaces by using their minds.
One of the biggest challenges associated with using BCIs in a clinical setting is that the neural recordings can be unstable. The individual controlling the BCI can eventually lose control due to variations in the signals picked up by BCI.
Whenever this loss of control happens, the individual must go through a recalibration process. The individual has to reset the connection between their mental commands and the tasks being performed, and another human technician often has to be present.
William Bishop is a fellow at Janelia Farm Research Campus. He was previously a PhD student and postdoctoral fellow in the Department of Machine Learning at CMU.
“Imagine if every time we wanted to use our cell phone, to get it to work correctly, we had to somehow calibrate the screen so it knew what part of the screen we were pointing at,” says Bishop. “The current state of the art in BCI technology is sort of like that. Just to get these BCI devices to work, users have to do this frequent recalibration. So that's extremely inconvenient for the users, as well as the technicians maintaining the devices.”
New Machine Learning Algorithm
The researchers presented a new machine learning algorithm capable of accounting for the varying signals. The individual is able to keep control of the BCI even when the instabilities are present. The researchers developed this after finding that neural population activity takes place in a low-dimensional “neural manifold.”
Alan Degenhart is a postdoctoral researcher in electrical and computer engineering at CMU.
“When we say ‘stabilization,' what we mean is that our neural signals are unstable, possibly because we're recording from different neurons across time,” says Degenhart. “We have figured out a way to take different populations of neurons across time and use their information to essentially reveal a common picture of the computation that's going on in the brain, thereby keeping the BCI calibrated despite neural instabilities.”
Previous Methods
Previous approaches to self-recalibration methods have also been faced with challenges surrounding instabilities. Unlike other methods, this one does not rely on the subject performing well during the recalibration process.
Byron Yu is a professor of electrical and computer engineering and biomedical engineering at CMU.
“Let's say that the instability were so large such that the subject were no longer able to control the BCI,” explains Yu. “Existing self-recalibration procedures are likely to struggle in that scenario, whereas in our method, we've demonstrated it can in many cases recover from those catastrophic instabilities.”
Emily Oby, a postdoctoral researcher in neurobiology at Pitt, spoke about the issue of instability as well.
“Neural recording instabilities are not well characterized, but it's a very large problem,” says Oby. “There's not a lot of literature we can point to, but anecdotally, a lot of the labs that do clinical research with BCI have to deal with this issue quite frequently. This work has the potential to greatly improve the clinical viability of BCIs, and to help stabilize other neural interfaces.”
The paper also included authors Steve Chase, professor of biomedical engineering and the Neuroscience Institute at CMU, along with Aaron Batists, associate professor of bioengineering at Pitt, and Elizabeth Tyler-Kabara, associate professor of neurological surgery at Pitt.
The research was funded by the Craig H Neilsen Foundation, the National Institutes of Health, DSF Charitable Foundation, National Science Foundation, PA Dept of Health Research, and the Simons Foundation.