Artificial Intelligence
Neural Networks Help Remove Clouds From Aerial Images
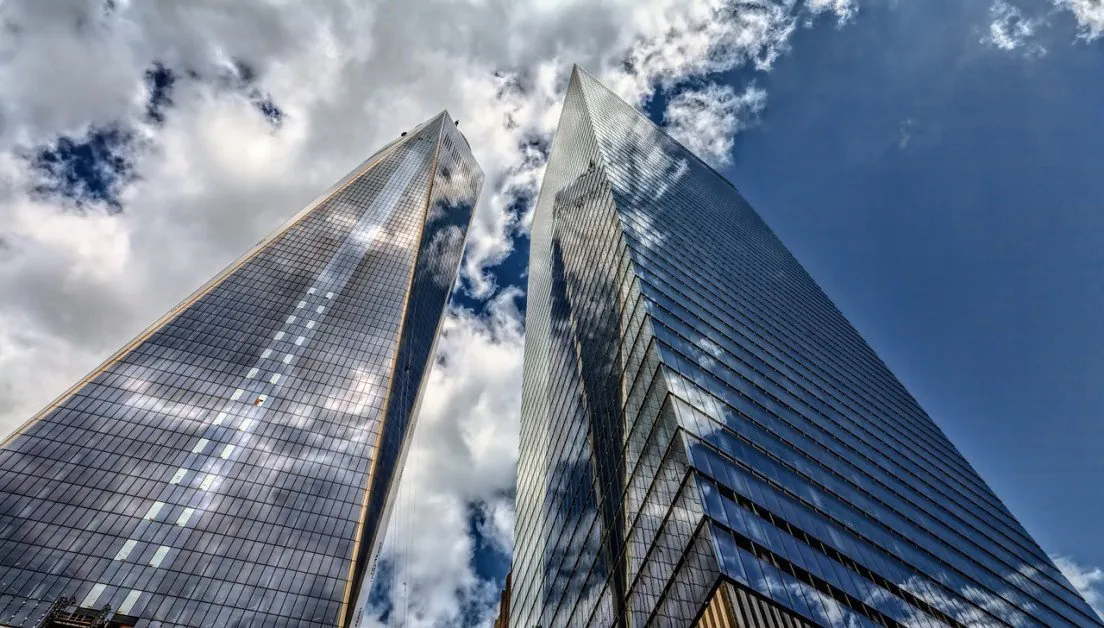
Researchers and scientists from the Division of Sustainable Energy and Environmental Engineering at Osaka University were able to digitally remove clouds from aerial images by using generative adversarial networks (GANs). With the resulting data, they could automatically generate accurate datasets of building image masks.
The research was published in Advanced Engineering Informatics.
The team put two artificial intelligence (AI) networks against each other to improve the data quality, and it didn't require previously labeled images. According to the team, these new developments could be used in fields like civil engineering, where computer vision technology is important.
Machine Learning for Repairing Images
Machine learning is often used to repair obscured images, like aerial images of buildings obscured by clouds. This task is able to be done manually, but it is time consuming and not as effective as machine learning algorithms. Even those algorithms already available require a large set of training images, so it is crucial to advance the tech further.
This is what the researchers at Osaka University did when they applied generative adversarial networks. One network is the “generative network,” and it proposes reconstructed images without the clouds. This network is put against a “discriminative network,” which relies on a convolutional neural network to distinguish between the digitally repaired pictures and real images without clouds.
As the networks proceed through this process, they both get increasingly better, which is what enables them to create highly realistic images with the clouds digitally erased.
Kazunosuke Ikeno is the first author of the paper.
“By training the generative network to ‘fool' the discriminative network into thinking an image is real, we obtain reconstructed images that are more self-consistent,” Ikeno says.
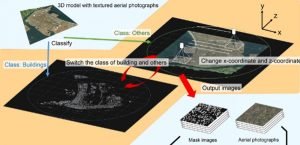
Image: 2021 Kazunosuke IKENO et al., Advanced Engineering Informatics
Training the System
The team relied on 3D virtual models with photographs from an open-source dataset, and this was used as the input. This enabled the system to automatically generate digital “masks” that overlaid reconstructed buildings over the cloud.
Tomohiro Fukuda is senior author of the research.
“This method makes it possible to detect buildings in areas without labeled training data,” Fukuda says.
The trained model was able to detect buildings with an “intersection over union” value of 0.651. This value is the measurement of how accurately the reconstructed area corresponds to the actual area.
According to the team, this method can improve the quality of other datasets with images that are obscured, it just needs to be extended. This can include images in various fields, such as healthcare, where it could be used to improve medical imaging.