Interviews
Mo Abdolell, CEO & Founder, Densitas Inc – Interview Series
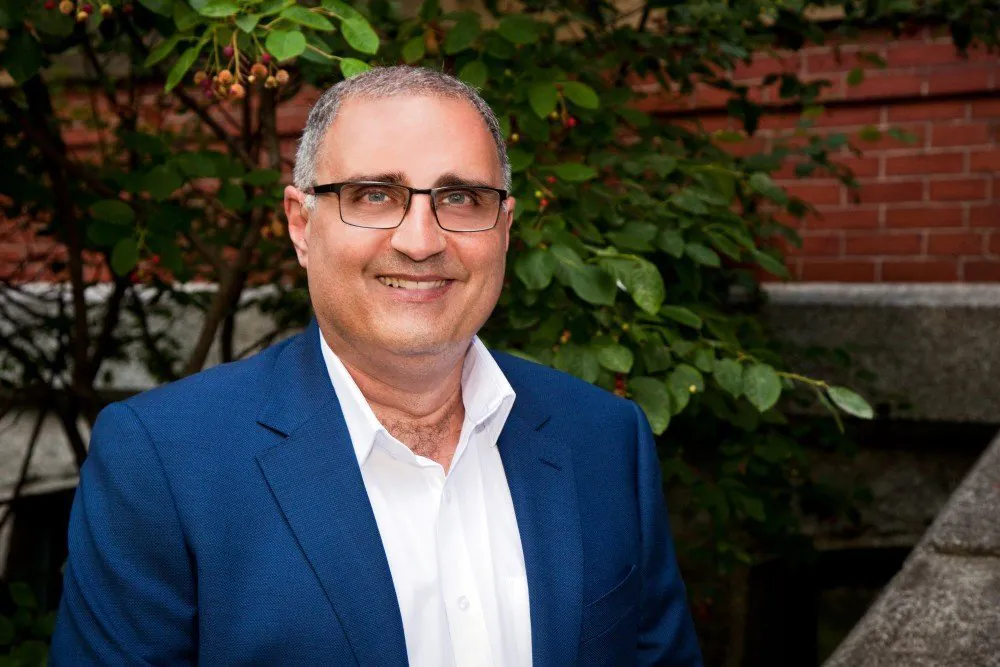
Mo Abdolell is CEO/Founder of Densitas Inc., a company focused on the mammography enterprise that delivers machine learning solutions for personalized breast health with technologies focused on breast density, clinical image quality, tailored risk.
Mo is also Associate Professor, Diagnostic Radiology, Dalhousie University and as a consulting biostatistician has 25 years experience in study design, statistical analysis and machine learning in biomedical/clinical research.
Can you walk us through your journey behind launching Densitas, Inc?
From as early as I can remember I wanted to help improve the health of underserved populations globally. I wanted to work for the World Health Organization in some capacity that made good use of my mathematical inclinations. Well that never quite worked out. Instead, I studied biostatistics in graduate school at the Dalla Lana School of Public Health, University of Toronto, completing my thesis in machine learning before moving on to work in hospitals and health research institutions. I have taught and supervised graduate students in diverse areas including biostatistics, epidemiology, biomedical engineering and health/medical informatics with a focus on diagnostic imaging. This has afforded me a front row seat at the intersection of diverse scientific disciplines and has given me a broad perspective on how artificial intelligence can be used to deliver better patient outcomes.
Someone who had a big impact on the decision to launch Densitas has been Dr. Judy Caines, who was the founding medical director of the Nova Scotia Breast Screening Program in Halifax, Canada. Dr. Caines was a true luminary and innovator in her clinical practice with an unwavering focus on achieving practical and sustainable solutions for improving quality of care and patient outcomes in women's breast health. Her absolute commitment and drive for excellence was inspiring. She supported and encouraged my early investigations in digital mammography, one of which led to the development of an algorithm to measure breast density as it appears in a mammogram. The very first thing that Dr. Caines said when the algorithm was completed was that she wanted to use it in clinical practice. This was the nudge that led to the launch of the company, ultimately closing the loop on my long standing desire to contribute in some way to improving global health by establishing a partnership with RAD-AID International to deliver better breast health in low-income countries and medically underserved regions of the world.
It may come as a surprise to many that breast density is a factor in the risk of breast cancer. Could you share your views on this?
Breast density refers to the amount of epithelial and stromal components of the breast tissue, and breast cancers most commonly appear in epithelial cells. So, the more epithelial tissue in the breast the greater chance that cancer may appear in the epithelial cells.
Additionally, dense breast tissue and underlying breast cancers both appear white on a mammogram, meaning that dense tissue can mask the presence of cancer and increase the risk of missing the cancer. In fact, half of all women have dense breasts, and half of breast cancers in dense breasts are missed.
These facts are consistent with research that shows women with extremely dense breasts have a 4-6x increased risk of having breast cancer compared to women with fatty breasts, and risk models that incorporate breast density predict breast cancer better than those that do not.
What are the different machine learning technologies that are used when it comes to analyzing breast density?
At a high level there are essentially three data modelling strategies for building algorithms that compute breast density. These include statistical learning, machine learning and deep learning, with each successive approach demanding increasingly larger labelled training data sets. Statistical learning and machine learning algorithms both require hand-crafted image features to be developed as inputs to predict breast density. Deep learning does not require hand-crafted image features to be developed, but rather discovers these features itself driven by the available training data.
How can AI Help Reduce Burnout for radiologists?
Burnout is recognized by the World Health Organization as an âoccupational phenomenonâ characterized by feelings of exhaustion, isolation, cynicism, and reduced professional engagement.
Bureaucratic tasks like charting, reporting, administrative paperwork and mandated accreditation responsibilities are the leading reported causes of burnout for the majority of radiologists.
AI automation integrated with digitalization can deliver better reporting and workflow efficiencies as well as better image quality and improved process management that liberate radiologists from tedious and repetitive reporting and administrative burdens that lead to burnout. Such solutions allow radiologists to dedicate more time to interpretive tasks and focus on patient care.
Densitas also helps imaging departments with workflow efficiencies, and compliance with national guidelines. Could you share with us how Densitas enables this?
Nearly 40 states across the U.S. have passed breast density notification legislation that mandates women must be informed of their breast density. This covers over 85% of the screen eligible population across the country. Breast density is visually reported according to the American College of Radiology BI-RADS density scale which has been shown to be unreliable and not reproducible, time-consuming, an additional reporting step, and distracting from the primary task of cancer detection.
A key aspect of the FDA Mammography Quality Standards Act (MQSA) Enhancing Quality Using the Inspection Program (EQUIP) is that the Lead Interpreting Physician shall ensure proper maintenance and updates of records concerning quality control (including corrective actions) and patient positioning, and corrective actions in the event of inadequate clinical image quality. Additionally, the MQSA EQUIP requires establishment of a QA program and maintenance of associated records. The MQSA EQUIP sets standards but is not prescriptive in its requirements. Clinical image quality is subjectively assessed and not standardized. Administration of a quality system focussed on the responsibilities of the LIP and lead QC technologist is not typically digitalized, is very time consuming and not reimbursable. Yet, mammography facility accreditation hinges on a facilityâs ability to demonstrate the effective implementation of a such a system.
The densitasai⢠platform delivers AI automation of breast density, clinical image quality and breast cancer risk assessment at the mammogram level at point-of-care as well as at the clinic and health system level through an advanced web-based analytics interface.
Integrations with industry leaders including Nuance, ikonopedia, Three Palm Software, and major PACS vendors ensure that breast density, image quality and risk scores are automatically embedded in systems that are already well-established in radiologistsâ reporting and workflows, eliminating transcription errors, improving reporting speed, and prioritizing studies for review.
The advanced web-based embedded analytics system provides automated reporting and auditing, digitalized workflows, health system wide QC and benchmarking of performance and resource utilization, and automation of administrative tasks.
Can you discuss Densitas partnership with RAD-AID International and how it helps underserved regions?
The aim of the partnership is to provide low-resource institutions with education, clinical support, and hands-on training so that they may adopt sustainable mammography practices that leverage practical AI powered applications. For breast imaging departments in participating institutions, the program is expected to help advance the quality of patient management decision-making and improve early disease detection.
Kenya is a country with nearly 5 million women who are eligible for screening mammography, but there are only 3 fellowship-trained breast imagers in the entire country. Tanzania is a country of 58 million people, but has just 450 technologists for the entire country. These are just two examples of many such underserved countries across the world. Screening so many women with only a handful of specialist-trained breast radiologists and radiological technologists is just impossible.
Large scale population-wide screening programs like breast cancer screening are characterized by high patient volumes that drive the need for efficient clinical workflows, standardization of processes and care, optimization of repetitive reporting and administrative tasks, cost-effective patient and process management, and adherence to national accreditation standards.
Densitasâ artificial intelligence solutions address these challenges with automated breast density, breast cancer risk and clinical image quality assessments.
Ultimately the goal is to help improve breast cancer screening through earlier detection and better treatment to save lives.
Densitas has also launched a program to help with radiology personnel during the COVID-19 pandemic. What is the program being offered?
In these unprecedented times, the primary focus of care is necessarily devoted to critically ill patients.
An unfortunate consequence of COVID-19 is that breast cancer screening has largely been put on hold.
Yet, even in the hardest hit regions we are seeing mammography facilities already planning to ramp up, starting with diagnostic and followed by screening mammography exam bookings. As that happens, the sheer number of patients awaiting breast screening in the coming weeks and months (rescheduled and net new) will present a monumental challenge to mammography facilities and health systems, with radiology personnel stretched thin.
It will be important to ensure that clinical image quality is maintained at the highest standard despite the stress of overloaded imaging throughput. AI automation of clinical image quality assessment, integrated in a comprehensive analytics and reporting platform, will boost MQSA inspection preparedness and will free up crucial resources for patient care. AI automation of breast density and breast cancer risk scoring and reporting will increase workflow efficiency and support tailored follow-up screening protocols.
We want to help. For a limited time we are offering our densitasai⢠platform as a no risk, no charge trial to select qualifying health systems, hospitals, and imaging centers.
The densitasai⢠platform will (1) help battle the impending backlog with AI automation of tasks, (2) provide workflow efficiencies that lessen the burden of tedious, (3) time-consuming and repetitive tasks and help mitigate staff burnout, (4) alleviate significant resource and administrative demands of FDA Mammography Quality Standards Act inspections, (5) digitalize processes to maximize social-distancing principles in clinical care on an ongoing basis.
The densitasai⢠platform can be deployed remotely.
Densitas will engage and support 20 sites. The deadline for registration and qualification is June 30, 2020 or when we are fully subscribed, whichever comes first.
Click Here to read more on how AI Can Help Reduce Burnout in Your Mammography Practice.
Thank you for the interview. There is a lot of important information here on breast health. Anyone who wishes to learn more should visit Densitas.