Thought Leaders
Importance of Data Quality in AI Implementation
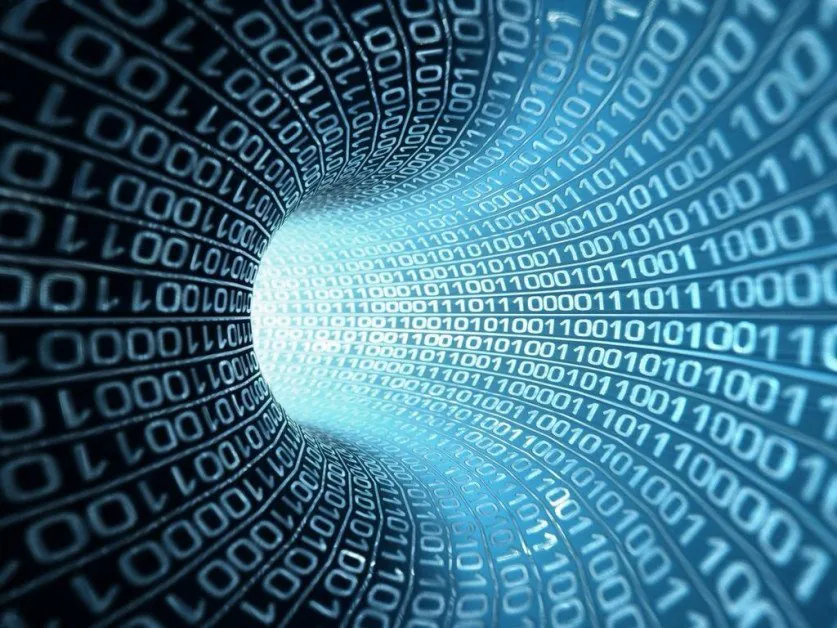
Artificial Intelligence and Machine Learning technologies can significantly benefit industries of all sizes. According to a McKinsey report, businesses that employ artificial intelligence technologies will double their cash flow by 2030. Conversely, companies that don’t deploy AI will witness a 20% reduction in their cash flow. However, such benefits go beyond finances. AI can help companies combat labor shortages. AI also significantly improves customer experience and business outcomes, making businesses more reliable.
Since AI has so many advantages, why isn’t everybody adopting AI? In 2019, a PwC survey revealed that 76% of companies plan to use AI to improve their business value. However, only a meager 15% have access to high-quality data to achieve their business goals. Another study from Refinitiv suggested that 66% of respondents said poor quality data impairs their ability to deploy and adopt AI effectively.
The survey found that the top three challenges of working with machine learning and AI technologies revolve around – “accurate information about the coverage, history, and population of the data,” “identification of incomplete or corrupt records,” and “cleaning and normalization of the data.” This demonstrates that poor quality data is the main hindrance for businesses to getting high-quality AI-powered analytics.
Why is Data So Important?
There are many reasons why data quality is crucial in AI implementation. Here are some of the most important ones:
1. Garbage In and Garbage Out
It’s pretty simple to understand that output depends heavily on the input. In this case, if the data sets are full of errors or skewed, the result will also set you off on the wrong foot. Most data-related issues are not necessarily about the quantity of data but the quality of data you feed into the AI model. If you have low-quality data, your AI models will not work properly however good they might be.
2. Not All AI Systems are Equal
When we think of datasets, we usually think in terms of quantitative data. But there are also qualitative data in the form of videos, personal interviews, opinions, pictures, etc. In AI systems, quantitative datasets are structured and qualitative datasets are unstructured. Not all AI models can handle both kinds of datasets. So, selecting the right data type for the suitable model is essential to get the expected output.
3. Quality vs. Quantity
It’s believed that AI systems need to ingest a lot of data to learn from it. In a debate about quality versus quantity, the latter is usually preferred by companies. However, if the datasets are high-quality yet shorter in nature, it will give you some guarantee that the output is relevant and robust.
4. Characteristics of a Good Dataset
The characteristics of a good dataset may be subjective and mainly depend on the application that AI is serving. However, there are some general features that one must be looking for while analyzing datasets.
- Completeness: The dataset must be complete with no empty grids or spots in the datasets. Every cell should have a data piece in it.
- Comprehensiveness: The datasets should be as comprehensive as they can get. For instance, if you’re looking for a cyber threat vector, then you must have all signature profiles and all necessary information.
- Consistency: The datasets must fit under the definite variables they have been assigned to. For instance, if you’re modeling package boxes, your selected variables (plastic, paper, cardboard, etc.) must have appropriate pricing data to fall into those definite categories.
- Accuracy: Accuracy is the key to a good dataset. All the information you feed the AI model must be trustworthy and completely accurate. If large portions of your datasets are incorrect, your output will be inaccurate too.
- Uniqueness: This point is similar to consistency. Each data point must be unique to the variable it is serving. For instance, you don’t want to price of a plastic wrapper to fall under any other category of packaging.
Ensuring Data Quality
There are many ways to ensure that the data quality is high, like ensuring that the data source is trustworthy. Here are some of the best techniques to make sure that you get the best quality data for your AI models:
1. Data Profiling
Data profiling is essential to understanding data before using it. Data profiling offers insight into the distribution of values, the maximum, minimum, average values, and outliers. Additionally, it helps in formatting inconsistencies in data. Data profiling helps understand if the data set is usable or not.
2. Evaluating Data Quality
Using a central library of pre-built data quality rules, you can validate any dataset with a central library. If you have a data catalog with built-in data tools, you can simply reuse those rules to validate customer names, emails, and product codes. Additionally, you can also enrich and standardize some data.
3. Monitoring and Evaluating Data Quality
Scientists have data quality pre-calculated for most datasets they want to use. They can narrow it down to see what specific issue an attribute has and then decide whether to use that attribute or not.
4. Data Preparation
Researchers and scientists usually have to tweak the data a bit to prepare it for AI modeling. These researchers need easy-to-use tools to parse attributes, transpose columns and calculate values from the data.
The world of artificial intelligence is continuously changing. While each company uses data in a different way, data quality remains imperative to any AI implementation project. If you have reliable, good-quality data, you eliminate the need for massive data sets and increase your chances of success. Like all other organizations, if your organization is shifting towards AI implementation, check if you have good quality data. Ensure that your sources are trustworthy and perform due diligence to check if they conform with your data requirements.
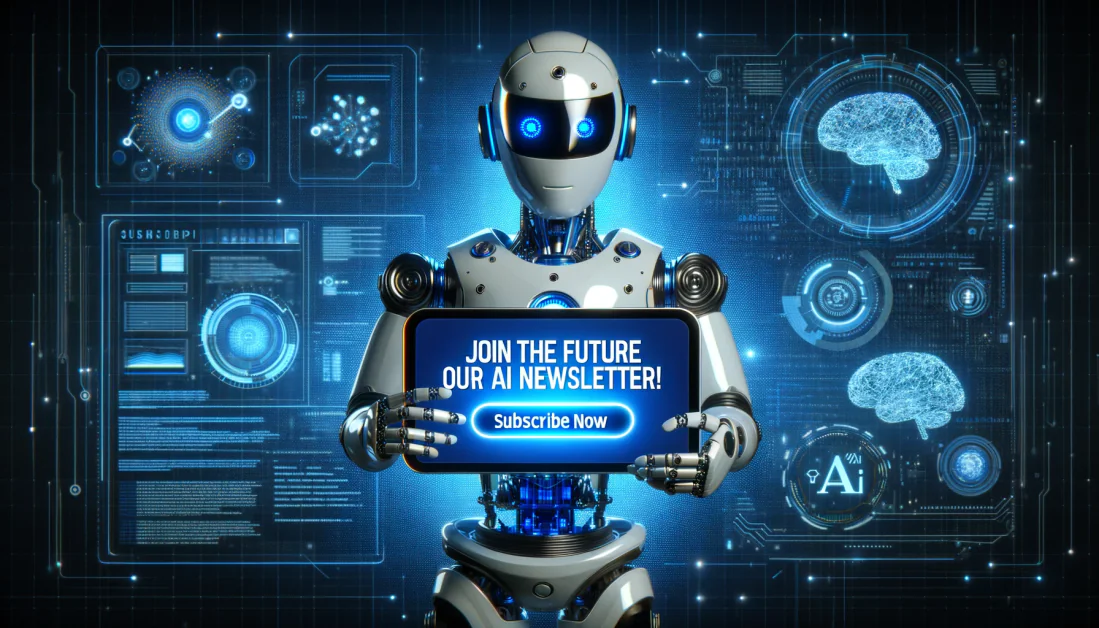
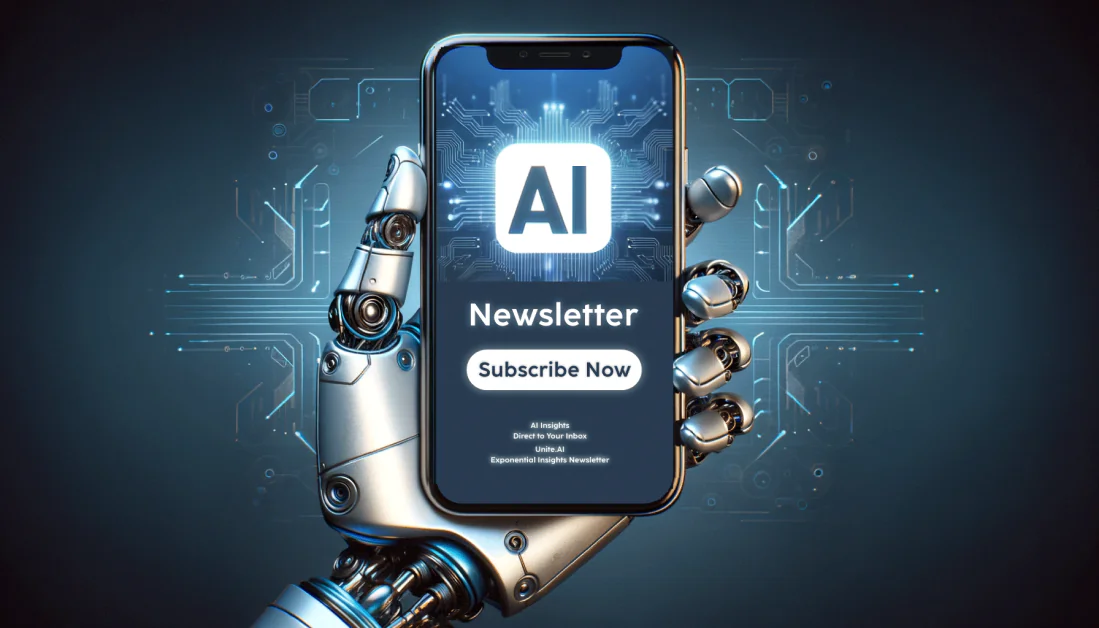