Artificial Intelligence
How to perform an AI Audit in 2023
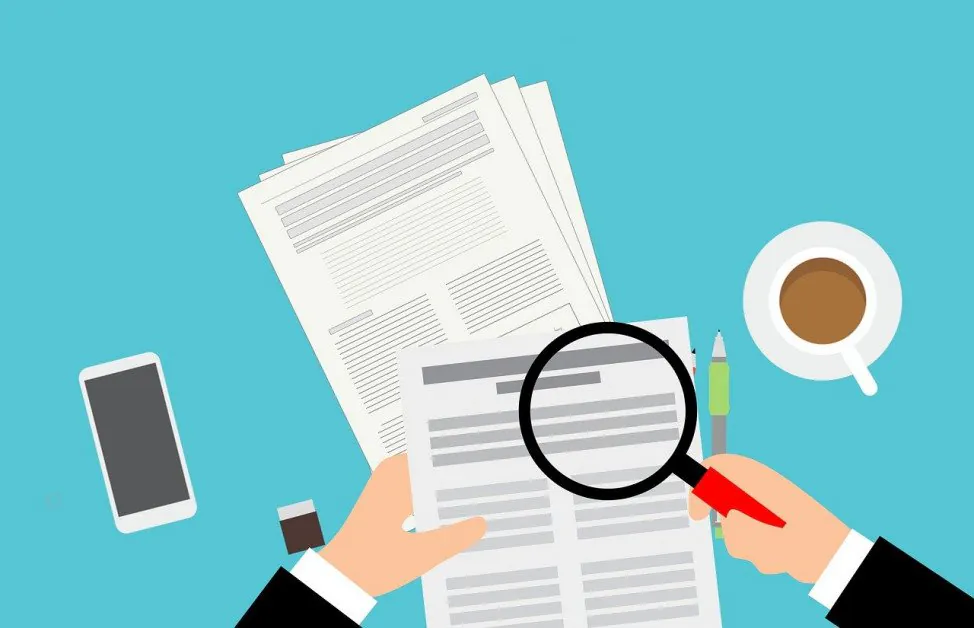
AI audit refers to evaluating AI systems to ensure they work as expected without bias or discrimination and are aligned with ethical and legal standards. AI has experienced exponential growth in the last decade. Consequently, AI-related risks have become a concern for organizations. As Elon Musk said:
“AI is a rare case where I think we need to be proactive in regulation rather than reactive.”
Organizations must develop governance, risk assessment, and control strategies for employees working with AI. AI accountability becomes critical in decision-making where stakes are high such as deploying policing in one area and not in the other, hiring and rejecting candidates.
This article will present an overview of AI audit, frameworks and regulations for AI audits, and a checklist for auditing AI applications.
Factors to Consider
- Compliance: Risk assessment related to an AI system’s compliance with legal, regulatory, ethical, and social considerations.
- Technology: Risk assessment related to technical capabilities, including machine learning, security standards, and model performance.
Challenges for Auditing AI Systems
- Bias: AI systems can amplify the biases in the data they are trained on and make unfair decisions. Recognizing this problem, a research problem research institute at Stanford University, Human Centered AI (HAI), launched a $71,000 Innovation Challenge to Design Better AI Audits. The objective of this challenge was to prohibit discrimination in AI systems.
- Complexity: AI systems, especially those employing deep learning, are complex and lack interpretability.
Existing Regulations & Frameworks for AI Audit
Regulations and frameworks act as the north star for auditing AI. Some important auditing frameworks and regulations are discussed below.
Auditing Frameworks
- COBIT Framework (Control Objectives for Information and related Technology): It is the framework for IT governance and management of an enterprise.
- IIA’s (Institute of Internal Auditors) AI Auditing Framework: This AI framework aims to assess the design, development, and working of AI systems and their alignment with the organization’s objectives. Three main components of IIA’s AI Auditing Framework are Strategy, Governance, and Human Factor. It has seven elements which are as follows:
- Cyber Resilience
- AI Competencies
- Data Quality
- Data Architecture & Infrastructure
- Measuring Performance
- Ethics
- The Black Box
- COSO ERM Framework: This framework provides a frame of reference for assessing the risks for AI systems in an organization. It has five components for internal auditing:
- Internal Environment: Ensuring that Organization’s governance and management are managing AI risks
- Objective Setting: Collaborating with stakeholders to make risk strategy
- Event Identification: Identifying risks in the AI systems such as unintended biases, data breaching
- Risk Assessment: What will be the impact of the risk?
- Risk Response: How will the organization respond to risk situations, such as sub-optimal data quality?
Regulations
The General Data Protection Regulation (GDPR) is a law in the EU regulation that puts obligations on organizations to use personal data. It has seven principles:
- Lawfulness, Fairness, and Transparency: Personal data processing must abide by the law
- Purpose Limitation: Using data only for a specific purpose
- Data Minimization: Personal data must be adequate and limited
- Accuracy: Data should be accurate and up to date
- Storage Limitation: Don’t store personal data that is not required anymore
- Integrity and Confidentiality: Personal data used to be processed securely
- Responsibility: Controller to process data responsibly following compliances
Other regulations include CCPA and PIPEDA.
Checklist for AI Audit
Data Sources
Identifying and vetting the data sources is the primary consideration in auditing AI systems. Auditors check for data quality and whether the company can use the data.
Cross Validation
Ensuring that the model is appropriately cross-validated is one of the checklists of the auditors. Validation data should not be used for training, and the validation techniques should ensure model generalizability.
Secure Hosting
In some cases, AI systems use personal data. It is important to evaluate that hosting or cloud services meet the information security requirements such as OWASP (Open Web Application Security Project) guidelines.
Explainable AI
Explainable AI refers to interpreting and understanding the decisions made by the AI system and the factors affecting it. Auditors check if models are sufficiently explainable using techniques such as LIME and SHAP.
Model Outputs
Fairness is the first thing that auditors ensure in model outputs. The model outputs should remain consistent when variables such as gender, race, or religion are changed. Moreover, the quality of predictions using the appropriate scoring method is also assessed.
Social Feedback
AI Auditing is a continuous process. Once deployed, auditors should see the social impact of the AI system. The AI system and risk strategy should be modified and audited accordingly based on the feedback, usage, consequences, and influence, either positive or negative.
Companies Who Audit AI Pipelines & Applications
Five major companies that audit AI are as follows:
- Deloitte: Deloitte is the largest professional services firm in the world and provides services related to auditing, taxation, and financial advisory. Deloitte employs RPA, AI, and analytics to help organizations in the risk assessment of their AI systems.
- PwC: PwC is the second largest professional services network by revenue. They have developed audit methodologies to help organizations ensure accountability, reliability, and transparency.
- EY: In 2022, EY announced an investment of $1 billion in an AI-enabled technology platform to provide high-quality auditing services. Firms that are AI-driven are well-informed to audit AI systems.
- KPMG: KPMG is the fourth largest accounting services-providing firm. KPMG provides customized services in AI governance, risk assessment, and controls.
- Grant Thronton: They help clients manage risks related to AI deployment and compliance with AI ethics and regulations.
Benefits of Auditing AI Systems
- Risk Management: Auditing prevents or mitigates risks associated with AI systems.
- Transparency: Auditing ensures that AI applications are free from bias and discrimination.
- Compliances: Auditing AI applications means that the system follows legal and regulatory compliances.
AI Auditing: What the Future Holds
Organizations, regulatory authorities, and auditors should keep in touch with AI advancements, realize its potential threats, and frequently revise the regulations, frameworks, and strategies to ensure fair, risk-free, and ethical use.
In 2021, 193 member states of UNESCO adopted a global agreement on the ethics of AI. AI is a continuously evolving ecosystem.
Want more AI-related content? Visit unite.ai.
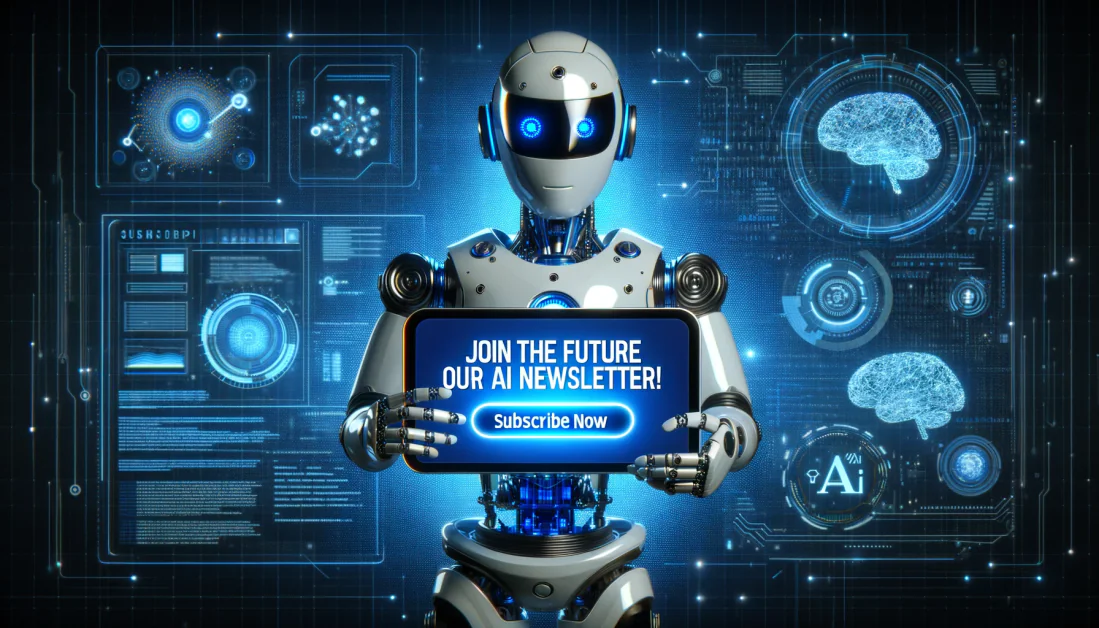
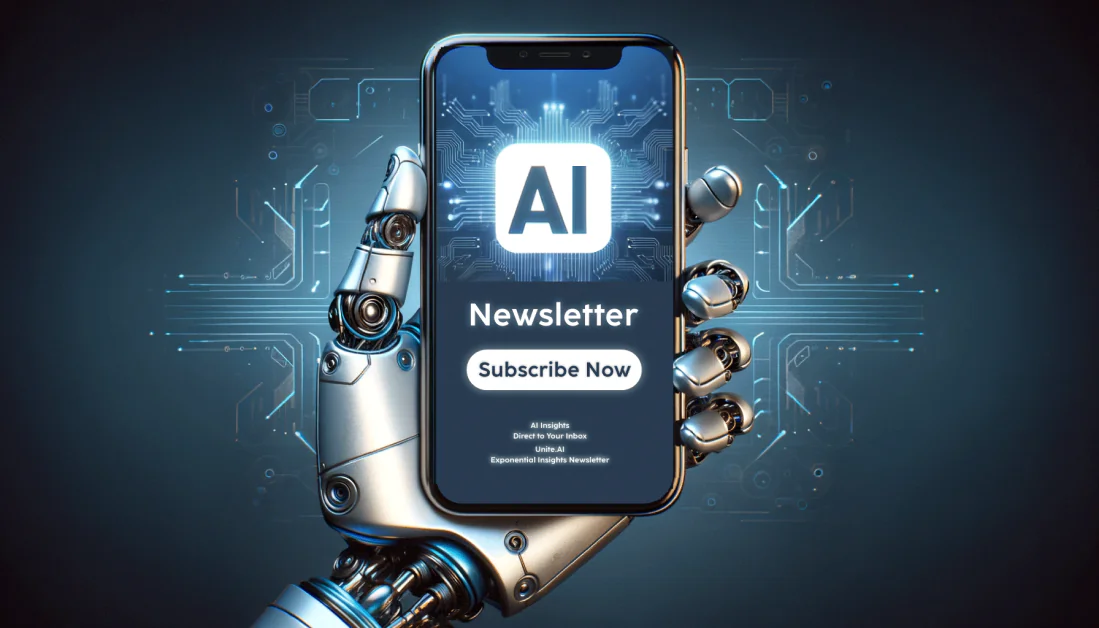