Thought Leaders
How Explainable AI Enhances Reliability and Trustworthiness
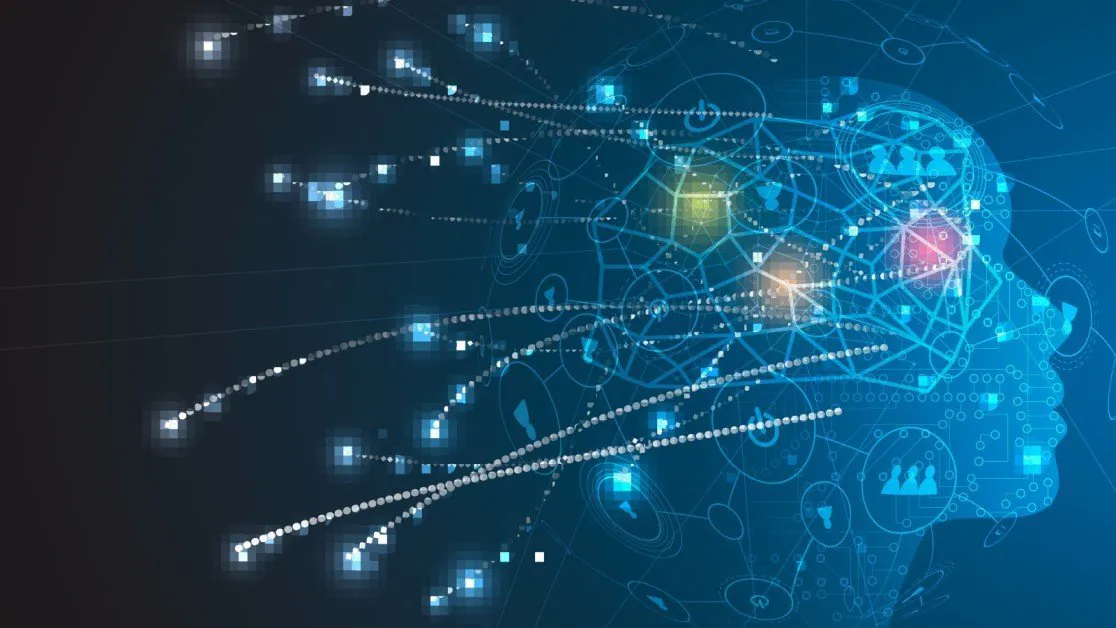
As Artificial intelligence (AI) is getting democratized across enterprises, it is slowly getting embedded in the fabric of our existence. An important aspect of this democratization is that end-users should be able to fully comprehend the process and mechanisms that AI is using to reach to a conclusion or how it is operating to deliver the desired outcomes. As human beings, we have a deep-rooted need to uncover the “why” and “how” of any phenomenon, which has accelerated our technological progress. In the context of AI, this understanding is termed as “explainability.”
Why Explainability is the need of the hour?
More often than not, we approach AI as a “black box”, where we only have awareness of the inputs and outputs, but somewhere the processes used are lost on us. Compounding this problem is the fact that the algorithms that power most popular forms of AI, such as complex deep learning-based prediction systems and Natural Language Processing (NLP) are highly abstract to even its most accomplished practitioners.
Trust and Transparency: For users to trust the predictions of AI, it must have some level of explainability inherent in it. For example, if a medical practitioner must recommend a treatment based on the predictions of AI, he/she needs to be confident on the prediction. A bank must have full confidence the decision to reject or approve a loan and be able to justify the same to all stakeholders. An AI used for screening and hiring must prove that the underlying mechanisms are fair and equitable to all cohorts of applicants.
Makes AI more human and increases adoption: In Mckinsey’s The state of AI in 2020 report we learn that a manufacturer uses extremely transparent models for acceptance from their factory workers, who need to trust the judgements made by AI regarding their safety. For rapid adoption of AI, getting the stakeholder buy-in is the major obstacle for scaling from simple point solutions to the enterprise level and get the most from the investment made. This is alleviated to a great extent if the performance is explainable to the larger audience. From a business perspective, explainability enhances the overall user-experience and increases customer satisfaction. As per the findings of an IBM Institute for Business Value survey, 68 percent of top executives believe customers will demand more explainability from AI in the next three years.
Uncover biases and improve model performance: A developer needs to know how he/she can improve the performance of the model, and how exactly to debug and finetune it. A clear explainability framework is one of the most important tools for conducting the thorough analysis that is needed.
Get sharper, well rounded insights: A complete 360-degree view is needed for fully understanding any prescriptions made by AI. For example, if AI is used for making an investment decision, one would also need to know the rationale behind it, so as to transfer this learning in other areas and also understand the potential pitfalls of taking that decision. A strong understanding of how AI operates will also enable decision makers to uncover new use-cases.
Regulations and Accountability: Several regulations like the GDPR are mandating a right to explanation, for addressing the accountability issues that arise from an automated decision-making process. In systems like autonomous vehicles, if something goes wrong leading to loss of life and property, proper knowledge is needed on the root cause, which will be hard to pinpoint in a black-box system.
How can AI be more explainable?
Explainable Artificial Intelligence Systems (XAI) are developed using different techniques that focus either on explaining the model as a whole or explaining the reasoning behind individual prediction through the assistance of some algorithm.
Majorly all explainability techniques are relying on:
- Disintegrating a model into individual components)
- Visualization of model predictions ( for example if a model classifies a car to be of a certain brand, it highlights the part which caused it to flag it as such)
- Explanation Mining (using machine learning techniques for finding relevant data that explains the prediction of an artificial intelligence algorithm).
In one such technique called proxy modeling, a simpler and more comprehendible model like a decision tree is used to approximately represent the more elaborate AI model. These simplistic explanations give a fair idea of the model at a high level but can sometimes suppress certain nuances.
Another approach is called “interpretability by design” This approach puts constraints in the design and training of AI network in a new fashion, that attempts to build the overall network from smaller and simpler explainable chunks . This involves a tradeoff between level of accuracy with explainability and limits certain approaches from the data scientist’s toolkit. It might also be highly compute intensive.
AI training and testing can also employ agnostic data verification techniques such as local interpretable model (LIME) and Shapley Additive exPlanations (SHAP), and these should be tailored to achieve high accuracy through the use of F-score, precision and other metrics. And, of course, all results should be monitored and verified using a wide variety of data. Using LIME, for example, organizations are able to create temporary models that mimic the predictions of non-transparent algorithms like machine learning. These LIME models can then create a wide range of permutations based on a given data set and its corresponding output, which can then be used to train simple and more interpretable models along with full lists of explanations for each decision and/or prediction. SHAP framework which has its foundations in game theory and specifically from cooperative game theory is a model that is . It combines optimal credit allocation with local explanations using the original Shapley values from game theory and their descendants.
Principled Operations
At a more strategic level, however, AI reliability frameworks should incorporate a broad set of principles aimed at ensuring proper outcomes both at the outset of deployment and over time as models evolve in the presence of changing circumstances. At a minimum, these frameworks should include things like:
- Bias Detection – all data sets should be scrubbed of bias and discriminatory attributes and then given the proper weight and discretion when applied to the training model;
- Human Involvement – operators should be able to investigate and interpret algorithm outputs at all times, particularly when models are used for law enforcement and the preservation of civil liberties;
- Justification – all predictions must be able to withstand scrutiny, which by nature requires a high degree of transparency to allow outside observers to gauge the processes and criteria used to produce results;
- Reproducibility – reliable AI models must be consistent in their predictions and must exhibit high levels of stability when encountering new data.
But XAI should not just be looked at as a means to improve profitability, but to bring in the accountability to ensure that institutions can explain and justify the effect of their creations on society as a whole.
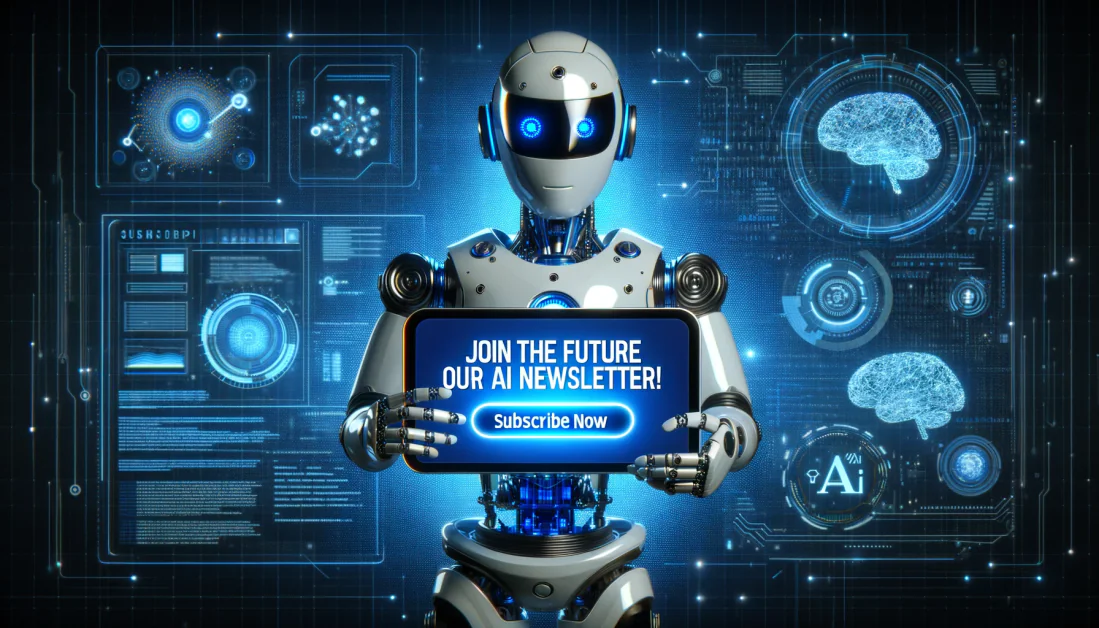
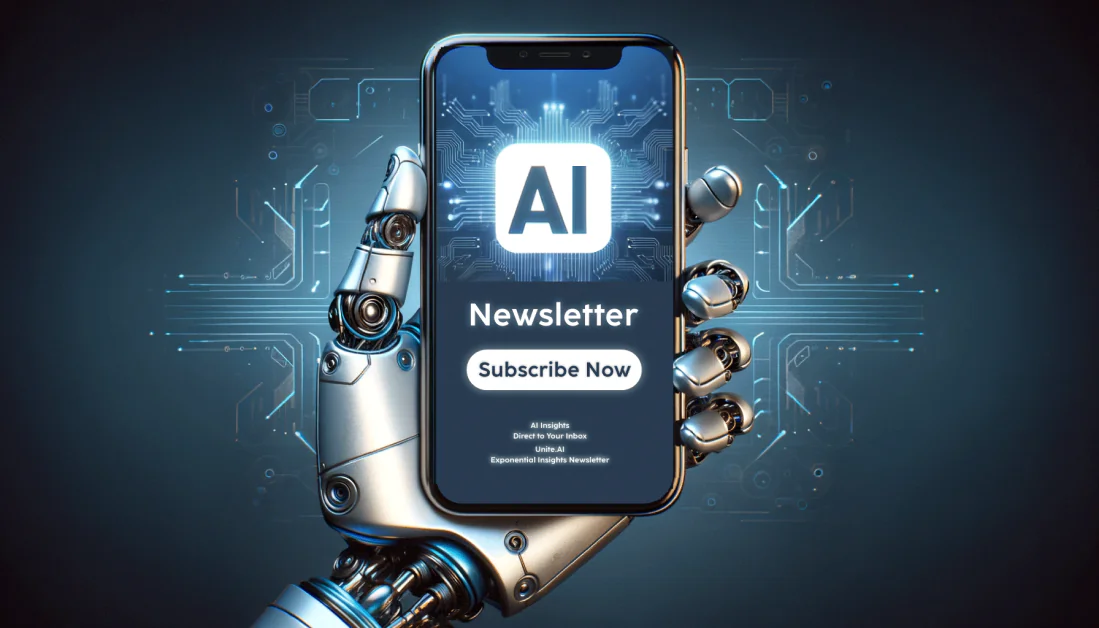