Thought Leaders
Generative AI in the Healthcare Industry Needs a Dose of Explainability

The remarkable speed at which text-based generative AI tools can complete high-level writing and communication tasks has struck a chord with companies and consumers alike. But the processes that take place behind the scenes to enable these impressive capabilities can make it risky for sensitive, government-regulated industries, like insurance, finance, or healthcare, to leverage generative AI without employing considerable caution.
Some of the most illustrative examples of this can be found in the healthcare industry.
Such issues are typically related to the extensive and diverse datasets used to train Large Language Models (LLMs) – the models that text-based generative AI tools feed off in order to perform high-level tasks. Without explicit outside intervention from programmers, these LLMs tend to scrape data indiscriminately from various sources across the internet to expand their knowledge base.
This approach is most appropriate for low-risk consumer-oriented use cases, in which the ultimate goal is to direct customers to desirable offerings with precision. Increasingly though, large datasets and the muddled pathways by which AI models generate their outputs are obscuring the explainability that hospitals and healthcare providers require to trace and prevent potential inaccuracies.
In this context, explainability refers to the ability to understand any given LLM’s logic pathways. Healthcare professionals looking to adopt assistive generative AI tools must have the means to understand how their models yield results so that patients and staff are equipped with full transparency throughout various decision-making processes. In other words, in an industry like healthcare, where lives are on the line, the stakes are simply too high for professionals to misinterpret the data used to train their AI tools.
Thankfully, there is a way to bypass generative AI’s explainability conundrum – it just requires a bit more control and focus.
Mystery and Skepticism
In generative AI, the concept of understanding how an LLM gets from Point A – the input – to Point B – the output – is far more complex than with non-generative algorithms that run along more set patterns.
Generative AI tools make countless connections while traversing from input to output, but to the outside observer, how and why they make any given series of connections remains a mystery. Without a way to see the ‘thought process’ that an AI algorithm takes, human operators lack a thorough means of investigating its reasoning and tracing potential inaccuracies.
Additionally, the continuously expanding datasets used by ML algorithms complicate explainability further. The larger the dataset, the more likely the system is to learn from both relevant and irrelevant information and spew “AI hallucinations” – falsehoods that deviate from external facts and contextual logic, however convincingly.
In the healthcare industry, these types of flawed outcomes can prompt a flurry of issues, such as misdiagnoses and incorrect prescriptions. Ethical, legal, and financial consequences aside, such errors could easily harm the reputation of the healthcare providers and the medical institutions they represent.
So, despite its potential to enhance medical interventions, improve communication with patients, and bolster operational efficiency, generative AI in healthcare remains shrouded in skepticism, and rightly so – 55% of clinicians don’t believe it’s ready for medical use and 58% distrust it altogether. Yet healthcare organizations are pushing ahead, with 98% integrating or planning a generative AI deployment strategy in an attempt to offset the impact of the sector’s ongoing labor shortage.
Control the Source
The healthcare industry is often caught on the back foot in the current consumer climate, which values efficiency and speed over ensuring ironclad safety measures. Recent news surrounding the pitfalls of near limitless data-scraping for training LLMs, leading to lawsuits for copyright infringement, has brought these issues to the forefront. Some companies are also facing claims that citizens’ personal data was mined to train these language models, potentially violating privacy laws.
AI developers for highly regulated industries should therefore exercise control over data sources to limit potential mistakes. That is, prioritize extracting data from trusted, industry-vetted sources as opposed to scraping external web pages haphazardly and without expressed permission. For the healthcare industry, this means limiting data inputs to FAQ pages, CSV files, and medical databases – among other internal sources.
If this sounds somewhat limiting, try searching for a service on a large health system’s website. US healthcare organizations publish hundreds if not thousands of informational pages on their platforms; most are buried so deeply that patients can never actually access them. Generative AI solutions based on internal data can deliver this information to patients conveniently and seamlessly. This is a win-win for all sides, as the health system finally sees ROI from this content, and the patients can find the services they need instantly and effortlessly.
What’s Next for Generative AI in Regulated Industries?
The healthcare industry stands to benefit from generative AI in a number of ways.
Consider, for instance, the widespread burnout afflicting the US healthcare sector of late – close to 50% of the workforce is projected to quit by 2025. Generative AI-powered chatbots could help alleviate much of the workload and preserve overextended patient access teams.
On the patient side, generative AI has the potential to improve healthcare providers’ call center services. AI automation has the power to address a broad range of inquiries through various contact channels, including FAQs, IT issues, pharmaceutical refills and physician referrals. Aside from the frustration that comes with waiting on hold, only around half of US patients successfully resolve their issues on their first call resulting in high abandonment rates and impaired access to care. The resultant low customer satisfaction creates further pressure for the industry to act.
For the industry to truly benefit from generative AI implementation, healthcare providers need to facilitate intentional restructuring of the data their LLMs access.
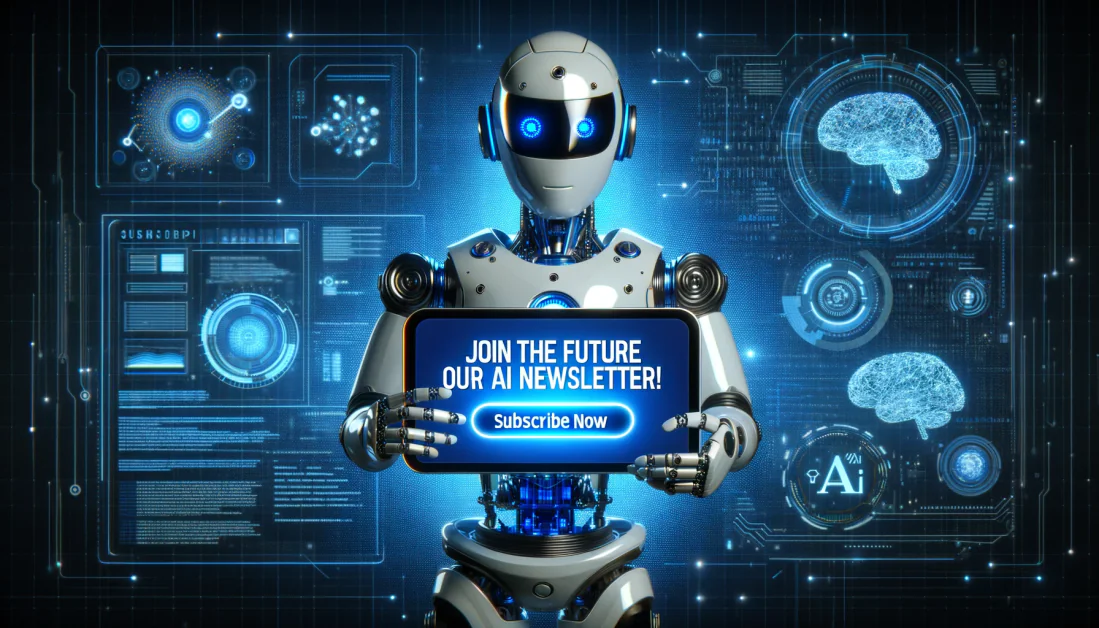
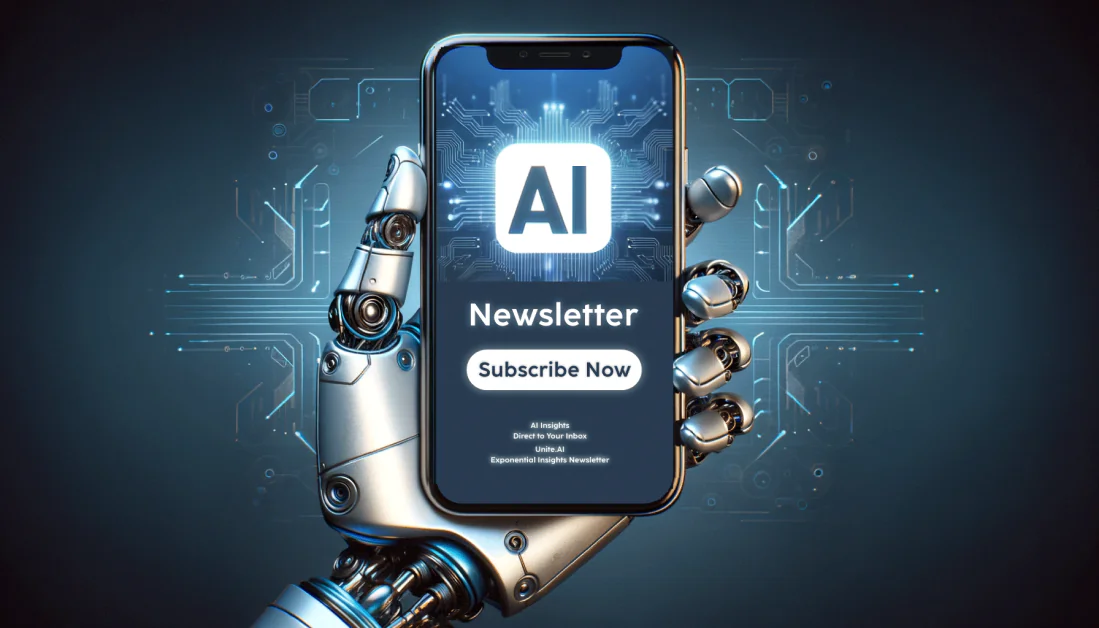