Thought Leaders
Explainability: The Next Frontier for Artificial Intelligence in Insurance and Banking
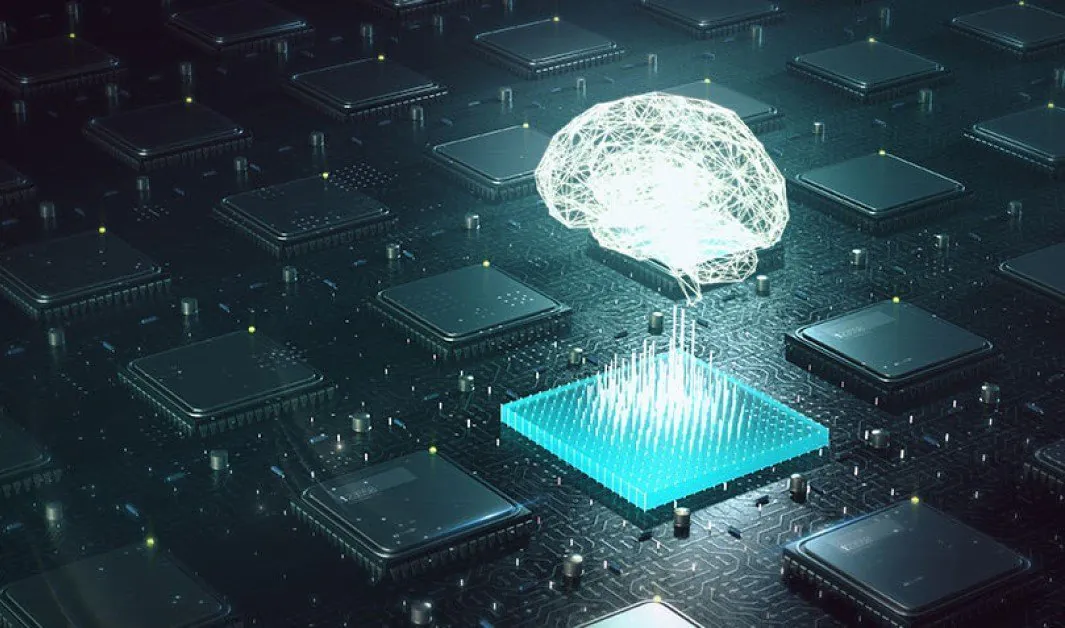
By Dr. Ori Katz, Analytical Research Scientist, Earnix.
“Any sufficiently advanced technology is indistinguishable from magic”, argued the science fiction writer Arthur C. Clarke. Indeed, sometimes advanced technology, such as new machine learning algorithms, resemble magic. Evolving applications of machine learning, including image classification, voice recognition, and its use in the insurance and banking industries have seemingly otherworldly properties.
Many companies are wary of changing their traditional analytical models – and rightly so. Magic is dangerous, especially if it is not well understood. Neural networks and tree ensemble algorithms are “black boxes”, their inner structure can be extremely complex. At the same time, several studies [1] have shown how neural networks and tree-based algorithms can outperform even the most carefully tuned traditional insurance risk models constructed by experienced actuaries. This is due to the ability of the new algorithms to automatically identify hidden structure in the data. The mystery and usefulness of neural networks and tree-based algorithms are juxtaposed. There is an inherent trade-off between the accuracy of an analytical model and its level of “explainability.” How can we trust models if we cannot understand how they reach their conclusions? Should we just give in to the magic, sacrifice our trust in and control over something we cannot fully comprehend for accuracy?
Managers and analysts are not the only ones who are concerned about this trade-off. Over the past few years, regulators have started to explore the dark side of the magic to increase their ability to monitor these industries. The banking and insurance industries are highly regulated in many aspects and current regulation trends involve taking a closer look at the models that are used to make predictions. The Recital 71 of the European General Data Protection Regulation (GDPR), for instance, states that customers should have the right to obtain an explanation of a single automated decision after it has been made. Since its inception, this element of the regulation has been at the center of a highly contentious academic debate.
The urgent need for explaining “black-box” analytical models has led to the emergence of a new research field: Explainable Artificial Intelligence. Experts are developing tools that enable us to peek inside the black-box and unravel at least some of the magic. Two types of tools that researchers have created include “Global Explainability” tools, which can help us understand key features driving the overall model predictions, and “Local Explainability” tools, which are meant to explain a specific prediction.
The following plot is an example of local explainability. It is based on the ideas of the Nobel Prize-winning economist Lloyd Shapley, who developed a game-theory method for calculating the contribution of several players cooperating in the same task. In Explainable Artificial Intelligence, the “players” are the model features, while the “task” is the prediction of the model. The numbers that describe the contribution of each feature are called “Shapley Values”. Researchers recently developed methods for fast estimation of Shapley Values [2], allowing us to fairly distribute a prediction among the different features.
Using Shapley Values to Explain the Predicted Renewal Demand of a Specific Customer
The plot, based on simulated data, shows the result of a demand model that predicts the probability of auto insurance policy renewal. This is a local explanation for a specific customer. The demand model is based on a complex ensemble of decision trees, but the plot presents the separate contribution of each feature to the final prediction. In this example, the model predicts that the average individual in the data will renew the policy with a probability of 0.64. However, for this specific customer, the predicted probability is much higher, at 0.72. The plot allows you to see the cause of this difference.
While we cannot fully understand the internal structure of this complex model, Shapley Values allow us to see what the most important features are for a specific prediction, unraveling a part of the magic. Averaging the individual Shapley Values over the population lets us see which features are most important and obtain global explainability of the model. Other popular explainability tools include “Permutation Feature Importance”, simple surrogate models that are fitted locally, and counterfactual examples, to name a few [3].
The new explainability tools are the necessary next step in the evolution of machine learning. They can allow insurance companies and banks to understand and trust their machine learning models, comply with new regulations, and provide their customers with valuable information. We can now partially overcome the trade-off between accuracy and explainability and enjoy the advantages of the new machine learning models with fewer concerns about their black-box nature.
In our rapidly digitalizing world, becoming fully analytics-driven is the baseline survival criteria for insurers and banks. This ability has always been important – but it became vital with the volatile market conditions that 2020 has brought upon us. Insurers and banks need smarter analytics to model a complex new reality on which they can base their business decisions and serve their customers faster and better. Explainability tools can allow insurers and banks to achieve that. With time, we will get to the point where machine learning models are no longer considered magic, but an essential tool in the core arsenal of any data-driven business.
Sources:
[1] Bärtl, M., & Krummaker, S. (2020). Prediction of claims in export credit finance: A comparison of four machine learning techniques. Risks, 8(1), 22.
Noll, A., Salzmann, R., & Wuthrich, M. V. (2020). Case study: French motor third-party liability claims. Available at SSRN 3164764.
Fauzan, M. A., & Murfi, H. (2018). The accuracy of XGBoost for insurance claim prediction. Int. J. Adv. Soft Comput. Appl, 10(2).
Weerasinghe, K. P. M. L. P., & Wijegunasekara, M. C. (2016). A comparative study of data mining algorithms in the prediction of auto insurance claims. European International Journal of Science and Technology, 5(1), 47-54.
[2] Lundberg, S. M., & Lee, S. I. (2017). A unified approach to interpreting model predictions. In Advances in neural information processing systems (pp. 4765-4774).
[3] See here for more details: https://christophm.github.io/interpretable-ml-book/index.html
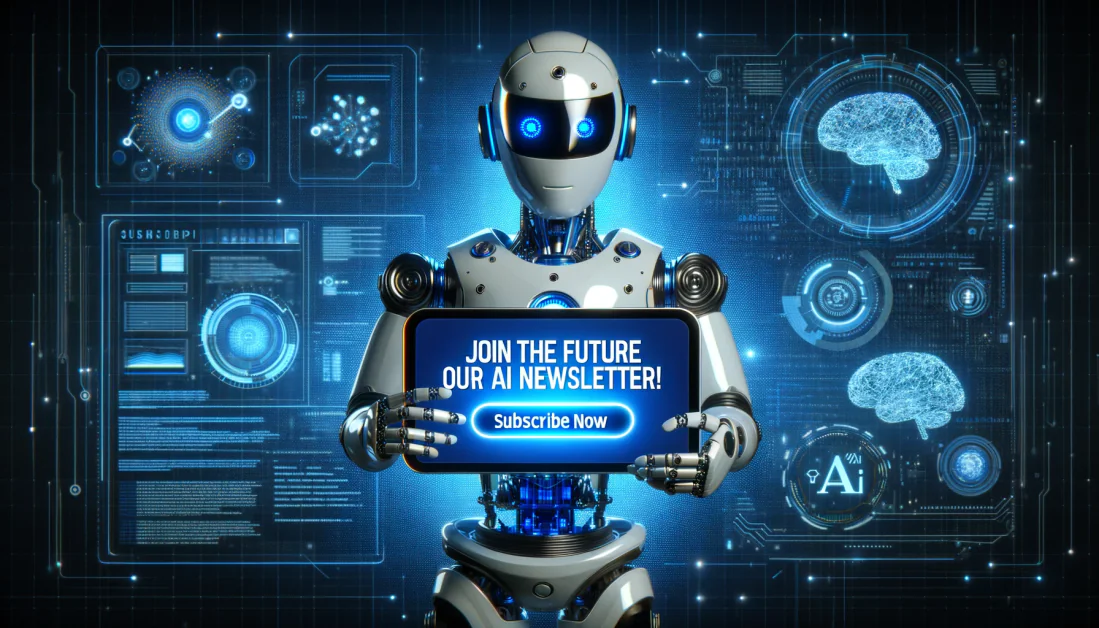
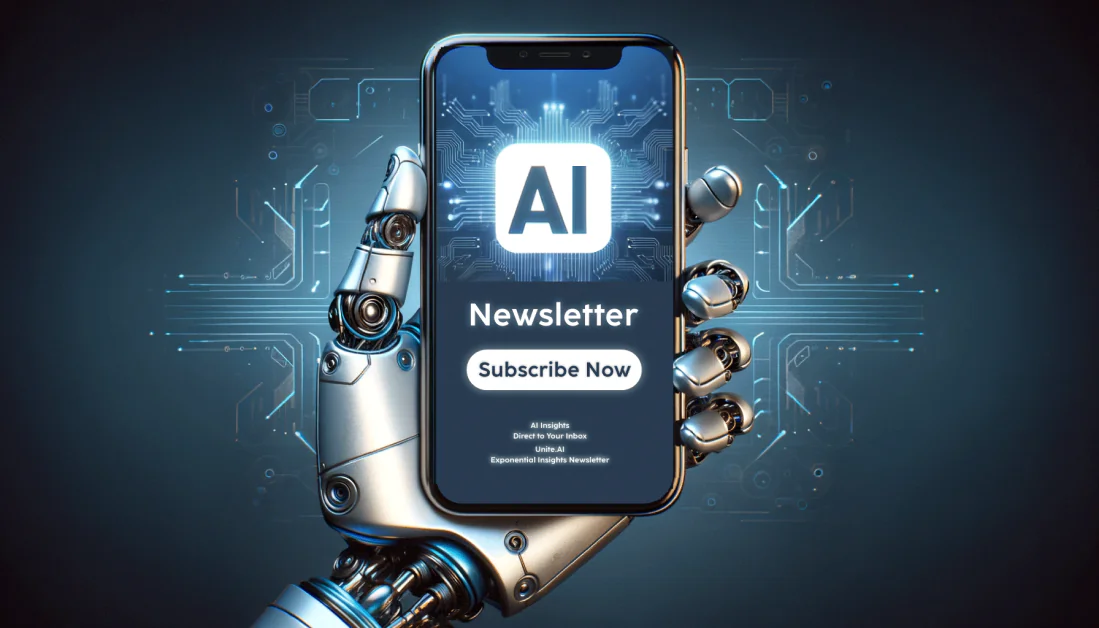